If I claimed that Americans have gotten more self-centered lately, you might just chalk me up as a curmudgeon, prone to good-ol’-days whining. But what if I said I could back that claim up by analyzing 150 billion words of text? A few decades ago, evidence on such a scale was a pipe dream. Today, though, 150 billion data points is practically passé. A feverish push for “big data” analysis has swept through biology, linguistics, finance, and every field in between.
Although no one can quite agree how to define it, the general idea is to find datasets so enormous that they can reveal patterns invisible to conventional inquiry. The data are often generated by millions of real-world user actions, such as tweets or credit-card purchases, and they can take thousands of computers to collect, store, and analyze. To many companies and researchers, though, the investment is worth it because the patterns can unlock information about anything from genetic disorders to tomorrow’s stock prices.
But there’s a problem: It’s tempting to think that with such an incredible volume of data behind them, studies relying on big data couldn’t be wrong. But the bigness of the data can imbue the results with a false sense of certainty. Many of them are probably bogus—and the reasons why should give us pause about any research that blindly trusts big data.
In the case of language and culture, big data showed up in a big way in 2011, when Google released its Ngrams tool. Announced with fanfare in the journal Science, Google Ngrams allowed users to search for short phrases in Google’s database of scanned books—about 4 percent of all books ever published!—and see how the frequency of those phrases has shifted over time. The paper’s authors heralded the advent of “culturomics,” the study of culture based on reams of data and, since then, Google Ngrams has been, well, largely an endless source of entertainment—but also a goldmine for linguists, psychologists, and sociologists. They’ve scoured its millions of books to show that, for instance, yes, Americans are becoming more individualistic; that we’re “forgetting our past faster with each passing year”; and that moral ideals are disappearing from our cultural consciousness.
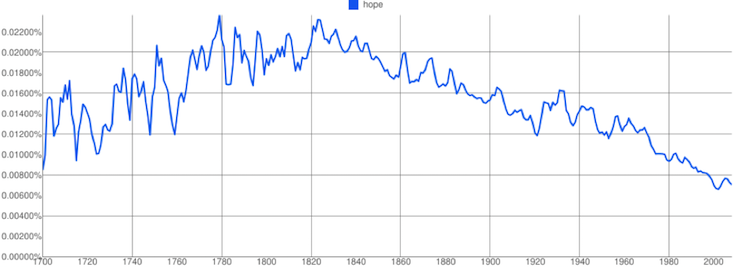
The problems start with the way the Ngrams corpus was constructed. In a study published last October, three University of Vermont researchers pointed out that, in general, Google Books includes one copy of every book. This makes perfect sense for its original purpose: to expose the contents of those books to Google’s powerful search technology. From the angle of sociological research, though, it makes the corpus dangerously skewed.
Some books, for example, end up punching below their true cultural weight: The Lord of the Rings gets no more influence than, say, Witchcraft Persecutions in Bavaria. Conversely, some authors become larger than life. From the data on English fiction, for example, you might conclude that for 20 years in the 1900s, every character and his brother was named Lanny. In fact, the data reflect how immensely prolific (but not necessarily popular) the author Upton Sinclair was: He churned out 11 novels about one Lanny Budd.
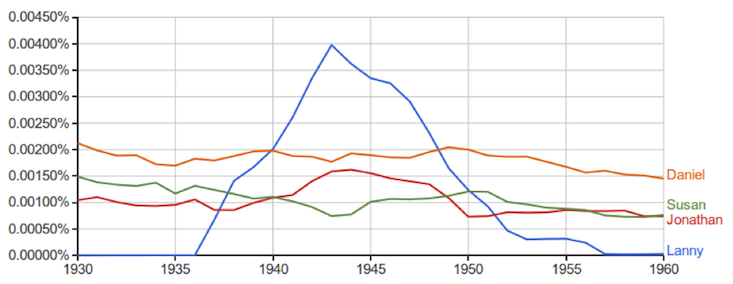
Still more damning is the fact that Ngrams isn’t a consistent, well-balanced slice of what was being published. The same UVM study demonstrated that, among other changes in composition, there’s a marked increase in scientific articles starting in the 1960s. All this makes it hard to trust that Google Ngrams accurately reflects the shifts over time in words’ cultural popularity.
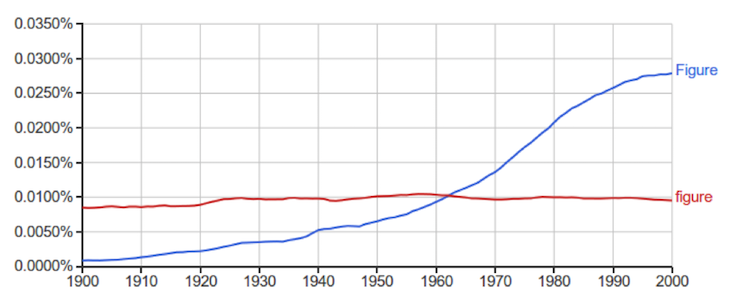
Even once you get past the data sources, there’s still the thorny issue of interpretation. Sure, words like “character” and “dignity” might decline over the decades. But does that mean that people care about morality less? Not so fast, cautions Ted Underwood, an English professor at the University of Illinois, Urbana-Champaign. Conceptions of morality at the turn of the last century likely differed sharply from ours, he argues, and “dignity” might have been popular for non-moral reasons. So any conclusions we draw by projecting current associations backward are suspect.
Of course, none of this is news to statisticians and linguists. Data and interpretation are their bread and butter. What’s different about Google Ngrams, though, is the temptation to let the sheer volume of data blind us to the ways we can be misled.
This temptation isn’t unique to Ngrams studies; similar errors undermine all sorts of big data projects. Consider, for instance, the case of Google Flu Trends (GFT). Released in 2008, GFT would count words like “fever” and “cough” in millions of Google search queries, using them to “nowcast” how many people had the flu. With those estimates, public health officials could act two weeks before the Centers for Disease Control could calculate the true numbers from doctors’ reports.
When big data isn’t seen as a panacea, it can be transformative.
Initially, GFT was claimed to be 97 percent accurate. But as a study out of Northeastern University documents, that accuracy was a fluke. First, GFT completely missed the “swine flu” pandemic in the spring and summer of 2009. (It turned out that GFT was largely predicting winter.) Then, the system began to overestimate flu cases. In fact, it overshot the peak 2013 numbers by a whopping 140 percent. Eventually, Google just retired the program altogether.
So what went wrong? As with Ngrams, people didn’t carefully consider the sources and interpretation of their data. The data source, Google searches, was not a static beast. When Google started auto-completing queries, users started just accepting the suggested keywords, distorting the searches GFT saw. On the interpretation side, GFT’s engineers initially let GFT take the data at face value; almost any search term was treated as a potential flu indicator. With millions of search terms, GFT was practically guaranteed to over-interpret seasonal words like “snow” as evidence of flu.
But when big data isn’t seen as a panacea, it can be transformative. Several groups, like Columbia University researcher Jeffrey Shaman’s, for example, have outperformed the flu predictions of both the CDC and GFT by using the former to compensate for the skew of the latter. “Shaman’s team tested their model against actual flu activity that had already occurred during the season,” according to the CDC. By taking the immediate past into consideration, Shaman and his team fine-tuned their mathematical model to better predict the future. All it takes is for teams to critically assess their assumptions about their data.
Lest I sound like a Google-hater, I hasten to add that the company is far from the only culprit. My wife, an economist, used to work for a company that scraped the entire Internet for job postings and aggregate them into statistics for state labor agencies. The company’s managers boasted that they analyzed 80 percent of the jobs in the country, but once again, the quantity of data blinded them to the ways it could be misread. A local Walmart, for example, might post one sales associate job when it actually wants to fill ten, or it might leave a posting up for weeks after it was filled.
So rather than succumb to “big data hubris,” the rest of us would do well to keep our skeptic hats on—even when someone points to billions of words.
Jesse Dunietz, a Ph.D. student in computer science at Carnegie Mellon University, has written for Motherboard and Scientific American Guest Blogs, among others. Follow him on Twitter @jdunietz.