For something so effortless and automatic, vision is a tough job for the brain. It’s remarkable that we can transform electromagnetic radiation—light—into a meaningful world of objects and scenes. After all, light focused into an eye is merely a stream of photons with different wave properties, projecting continuously on our retinas, a layer of cells on the backside of our eyes. Before it’s transduced by our eyes, light has no brightness or color, which are properties of animal perception. It’s basically a mess of energy. Our retinas transform this energy into electrical impulses that propagate within our nervous system. Somehow this comes out as a world: skies, children, art, auroras, and occasionally ghosts and UFOs.
It’s even more amazing when you take a closer look. The image projected in each eye is upside down, having a slightly different perspective than the image in the other eye. Our nervous system reorients them, matches each point in the projection, and uses the difference in geometry between the images to create the appearance of depth—stereopsis—in a unified scene. It processes basic features like shape and motion. It separates the objects from each other and the background, and distinguishes visual motion caused by our own movement or the movement of objects. Estimates of the percentage of the human brain devoted to seeing range from 30 percent to over 50 percent.
Each new cell-type seemed like finding a new piece of a jigsaw puzzle.
Today, mapping the human brain is one of the great projects in science. Scientists have a long way to go before they understand the brain’s architecture and interaction of billions of neurons behind our every action and perception. Visual processing, though, is one of the most well understood aspects of brain activity. Scientists are now modeling the brain’s visual system with ever-more powerful computers, gaining new insights into how vision works, and in turn transforming those insights into new technologies.
Computers can now detect the presence of specific objects and identify people; they can reconstruct three-dimensional scenes from two-dimensional images, find tumors, help forensic scientists identify evidence, and navigate vehicles autonomously through busy streets. They are being developed to explore the bottom of the ocean and defuse dangerous minefields. In the future, computer vision is projected to play a key role in proactive safety systems, identifying, Minority Report-style, criminal suspects, or patterns of criminal behavior, before they happen.
How has vision technology come so far? It arose from an aspect of science that leads to many breakthroughs: serendipity. Vision science wouldn’t be where it is today without an accident that took place in a physiology lab in Baltimore over half a century ago.
In 1958, studying the responses of nerve cells in living animals was difficult. To study vision, it was typical of physiologists to use anaesthetized cats. But the examination setups that carefully held them in place were extremely sensitive to movement; even a pulsation from a heartbeat could throw everything off. This changed when David Hubel, a 32-year-old scientist from Canada, through careful machining methods, invented a tungsten electrode and a hydraulic positioning system, as part of his postdoctoral research at Johns Hopkins University.
Armed with the advanced tech, Hubel and a new colleague, Torsten Wiesel—a budding Swedish researcher around the same age—set out to explore the visual cortex of living cats. It was brave new territory for physiology and they were optimistic because, with the fancy electrode, it was now possible to record the activity of individual neurons. They wanted to figure out exactly what these cells might be doing to contribute to vision. Would they, for example, find cells that responded to different, identifiable objects—forks, mountains, kitchens? They were going in blind, if you can excuse the pun.
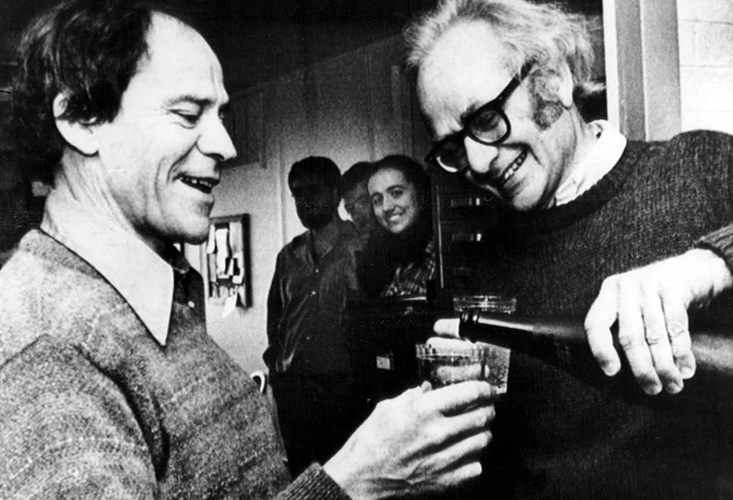
Building on knowledge dating back to Santiago Ramón y Cajal’s 19th-century discoveries in neuroscience, they knew a thing or two about the wiring and response patterns of the nerve cells in the retina and in the brainstem that feed into cells in the visual cortex. They knew how to trigger these retinal and brainstem neurons to pass electrical impulses—information, essentially—by projecting spots of light. These spots had to be focused within the area of the cat’s visual field that a given cell was sensitive to.
Recording sessions took many hours—it was a victory in and of itself if you could find a cell that you could reliably stimulate even just a bit, and sometimes you didn’t “get lucky” until searching into nightfall. That meant you’d be spending all night in the lab trying, through trial and error, to find a pattern in a cell’s responses by playing with the location, size, or luminance of the spot. It was tedious work. Once Hubel and Wiesel even tried using pictures of women from magazines to stimulate the cat’s neurons. But after a month of the experiments, they simply weren’t getting anywhere.
Then, what started out as another uneventful recording session turned into an adventure that won them a Nobel Prize. After a few hours of trying again with spots, they finally located a cortical cell in a cat that gave them a small response. Then, while swapping out the projector slide, while there was no spot on their screen, the cell suddenly went off like a machine gun! It was unlike any response they’d heard before. What caused it? Their hearts were racing.
After calming down, they tried reinserting the slide again. But to their dismay, it didn’t work. They realized they had to do exactly what they had just done. But what did they do? If only they could remember. I asked Wiesel, who is now 97, to recount what happened next. Excitedly, over Zoom, Wiesel told me, “We got the bright idea to change the orientation of the slide edge, and that’s how we discovered it! We rushed down the halls and called in everybody because we realized that this was amazing. Really amazing.” What they discovered was that the only thing that could have triggered so much activity in the cell was the thin dark line on the projector screen resulting from the edge of the glass slide with the dot on it. The key to getting the cell to fire was to project that slide edge at the right orientation.
In the following weeks, Hubel and Wiesel discovered a response pattern in the cortical cells. Projected lines triggered each of them, but only when shown at specific angles. When you went away from a cell’s “preferred” angle, there was a graded decrease in the number of electrical impulses they churned out, reaching a minimum of firing with the line at about a 90-degree angle, relative to whatever its maximum response orientation was. Meanwhile, almost as if to intentionally confuse them, the two scientists also discovered other cortical cells that strangely had no preferred orientation. These cells didn’t care about the line’s angle, just that there was a line. They called the former simple cells and the latter complex cells because they attributed differences in the response patterns to simple wiring and complex wiring, relatively speaking. Nonetheless, each new cell-type seemed like finding a new piece of a jigsaw puzzle. But was there a unifying principle here? Did these pieces fit together?
Somehow this comes out as a world: skies, children, art, auroras, ghosts, and UFOs.
They did. The upshot of their exploration was that each type of cell they found had a response pattern that could be generated from the cells that fed into it, and the cells that fed into those cells. It seemed there was a network composed of nodes and layers in a hierarchy that began at the retina. It was a revelation of how these cells function together to create a neural representation of something in the cat’s visual field; a physical instantiation corresponding exactly to what the cat could be seeing at that very moment. It was a profound connection between mind and brain.
Hubel and Wiesel showed that vision was constructed through a cascade of firing cells. Each bit of visual information stimulated a particular cell that triggered others. This expanding network constituted a hierarchical system in the brain that resulted in the identity of an image—an object, or face. The idea, taken to an extreme, forms the basis for what’s become known as the Grandmother Cell hypothesis—that at the highest level, there could be cells so abstract, they only activate when stimulated by a conceptual entity, like a grandmother. Although the idea remains controversial, in 2005, researchers at UCLA came eerily close to proving it when they found cells that fired when subjects viewed specific celebrity faces, like Jennifer Aniston, or Bill Clinton.
I asked Margaret Livingstone, a student and longtime colleague of David Hubel who’s now a visual neuroscientist at Harvard, about the importance of Hubel and Weisel’s early discoveries. Without hesitation, she explained that the significance is “that our brains are arranged in a hierarchical series of areas that each perform similar computations on their inputs, like a neural network.”
In the late 1950s, alongside Hubel and Wiesel’s discoveries, the United States Navy funded the development of a computer with a sci-fi B-movie name: Perceptron. Large, clunky, and nightmarish, really, it contained a camera with an array of 400 photocells connected, rat’s-nest style, to a layer of artificial “neurons” that could process image information and identify patterns after training. Although The New York Times touted it as “the embryo of an electronic computer that … will be able to walk, talk, see, write, reproduce itself and be conscious of its existence,” once in use it was soon revealed that even its basic pattern recognition capability was disappointingly limited to the types of patterns it was trained on, relative to such outrageously expanded expectations.
Success in computer vision required inspiration from Hubel and Wiesel’s discoveries. In 1980, it came in the form of the Neocognitron (see a pattern here?), a neural network invented by the Japanese computer scientist, Kunihiko Fukushima. Paralleling biological visual architecture, the neocognitron had elementary units called s-cells that fed into c-cells, modeled after simple and complex cells, respectively. They were networked in a cascading, hierarchical fashion, just like in a brain. After training, it could recognize typed and even handwritten characters. It was the first of its kind that could learn to create information from unique spatial patterns drawn by anyone and use that information to essentially make decisions about abstract categories to identify meaningful, understandable symbols.
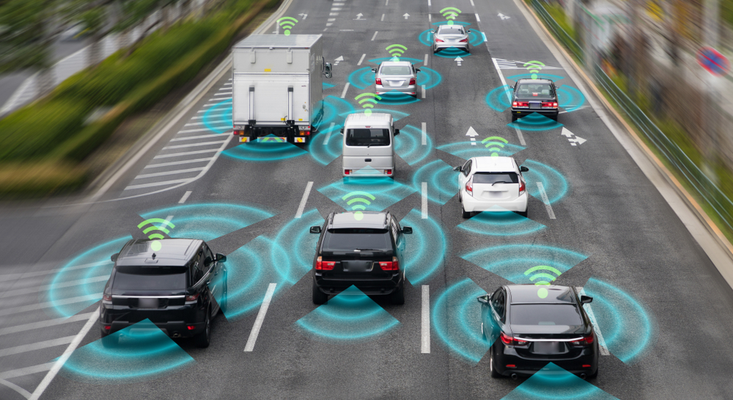
In the late 1990s, the Neocognitron was followed up by another biologically inspired model dubbed HMAX for “Hierarchical Model and X,” which was more flexible in terms of the kinds of objects it could recognize. It could identify them from different viewpoints and at different sizes. Leading this project was Tomaso Poggio, originally trained in theoretical physics, and now regarded as a pioneering computational neuroscientist at MIT. He, along with the late David Marr, are credited with using a physiologically inspired approach to modeling neural processes computationally to create vision in computer systems.
Poggio’s HMAX also included simple and complex processing nodes where the simple nodes fed into the complex nodes. The output of the highest processing nodes in the hierarchy approximated a neural representation of the object in view—the outputs were even termed “neurons”—such that their model firing rates peaked when the images they were shown contained objects they were trained to identify. In a 1999 publication about HMAX, published in Nature, Poggio and his colleagues remark that their model was an extension of Hubel and Wiesel’s hierarchical model of visual processing.
Neocognitron “was the first computer model that really followed this hierarchy,” Poggio told me in a recent interview. “In my group, we did something similar, which was more faithful to the physiology that Hubel and Wiesel found. You could think of it as a sequence with layers of logical operations that correspond to simple and complex cells and their combinations to get the selectivity properties of neurons high up in the visual pathway.” Basically, with HMAX, Poggio was able to mimic neurons that receive input from not only simple and complex cells, but many others further along in the processing sequence. In cat and human brains, these “high-up” neurons are activated not by oriented lines, but by complex figures like 3-D shapes, no matter what viewpoint. To do this, these neurons must learn the features they become tuned to identify.
We got the bright idea to change the orientation of the slide edge. That’s how we discovered it!
Incredibly, the connective structure or architecture of these models is seen today in cutting-edge algorithms that train computer vision systems to identify objects on the fly. The most widely known example involves autonomous driving. Tesla’s smart-summon feature allows its vehicles to park autonomously and maneuver themselves out of a parking spot. Newer models will have camera-based neural net technology for full guidance during autopilot.
Computers with visual intelligence are currently employed in security, agriculture, and medicine, where they’re used to spot, with varying degrees of efficiency, the bad apples—suspects, weeds, and tumors. The breakthroughs have come along with huge increases in information processing power and massive sets of training images, in what’s known as Deep Learning, a system-architecture with multiple layers of processing nodes between input and output. In a manner similar to simple and complex cells, the layers of these models are networked in a feed-forward manner as they are in mammalian brains. Indeed, Poggio said, “The architecture of Deep Learning models were originally inspired by the biological data of Hubel and Wiesel.”
While Deep Learning’s architecture is inspired by the human brain, its methods of visual recognition are not a perfect parallel. For example, AI systems that learn to recognize objects or people often rely on a process called backpropagation, which involves re-weighting the connection strength between artificial neurons according to error rates, in ways that are agreed upon by neuroscientists to be anatomically and physiologically unlikely or even impossible. Poggio said that “‘backprop’ doesn’t correspond accurately with what we know about how the brain processes visual information.” He also describes it as a remarkably clunky, inefficient process. “In some AI systems it requires megawatts of power, yet our brains do it while running only on the energy from the food we eat,” Poggio said.
Still, he pointed out, scientists are recapitulating the brain’s visual processing power with computations in more detail, potentially leading to better visual processing in AI. Already artificial neural networks that show direct correspondence with actual brain architecture can accurately predict neural responses to images. In a 2019 experiment, a group of scientists at MIT designed a computer model to stimulate neural activity in the brain’s visual system, based on neuroscientific findings. They had the computer generate an image that triggered the desired pattern of neural activity. When the scientists showed the synthesized images to monkeys, with electrodes implanted in their brains to record their neural activity, the monkeys’ visual system fired just as the scientists predicted. The potential to stimulate neural activity, notably in people who have suffered a brain injury, is, scientists say, exciting. It’s something out of science fiction.
Wiesel told me that he and Hubel felt that kind of excitement when they made their first discoveries. “We felt more like explorers than scientists,” Wiesel said. Their explorations are still charting new courses in science today.
Phil Jaekl is a freelance science writer and author with an academic background in cognitive neuroscience. His latest book is Out Cold: A Chilling Descent into the Macabre, Controversial, Lifesaving History of Hypothermia. He lives in the Norwegian Arctic in Tromsø.
Lead image: Blue Planet Studio / Shutterstock