We don’t know what our customers look like,” said Craig Berman, vice president of global communications at Amazon, to Bloomberg News in June 2015. Berman was responding to allegations that the company’s same-day delivery service discriminated against people of color. In the most literal sense, Berman’s defense was truthful: Amazon selects same-day delivery areas on the basis of cost and benefit factors, such as household income and delivery accessibility. But those factors are aggregated by ZIP code, meaning that they carry other influences that have shaped—and continue to shape—our cultural geography. Looking at the same-day service map, the correspondence to skin color is hard to miss.
Such maps call to mind men like Robert Moses, the master planner who, over decades, shaped much of the infrastructure of modern New York City and its surrounding suburbs. Infamously, he didn’t want poor people, in particular poor people of color, to use the new public parks and beaches he was building on Long Island. Though he had worked to pass a law forbidding public buses on highways, Moses knew the law could someday be repealed. So he built something far more lasting: scores of overpasses that were too low to let public buses pass, literally concretizing discrimination. The effect of these and dozens of similar decisions was profound and persistent. Decades later, bus laws have in fact been overturned, but the towns that line the highways remain as segregated as ever. “Legislation can always be changed,” Moses said. “It’s very hard to tear down a bridge once it’s up.”
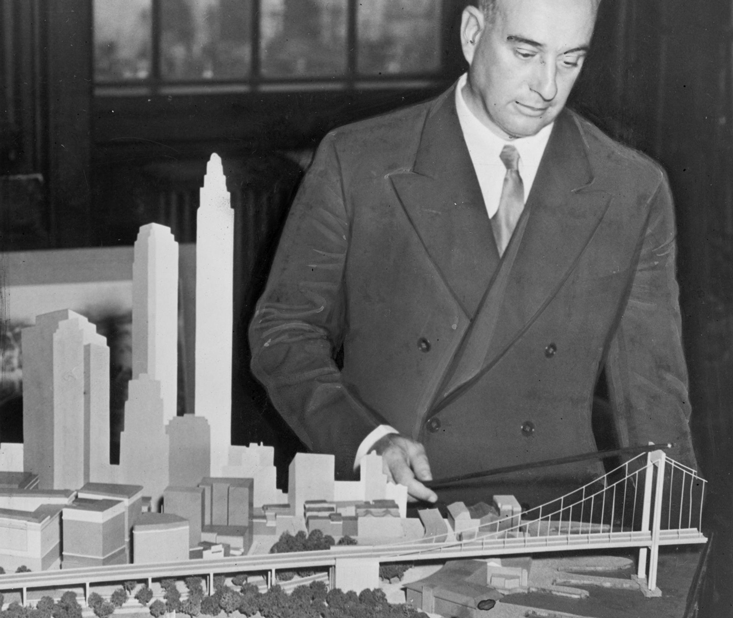
Today, a new set of superhighways, built from data shaped by the old structures, refresh these divisions. While the architects of the new infrastructure may not have the same insidious intent, they can’t claim ignorance of their impact, either. Big-data practitioners understand that large, richly detailed datasets of the sort that Amazon and other corporations use to deliver custom-targeted services inevitably contain fingerprints of protected attributes like skin color, gender, and sexual and political orientation. The decisions that algorithms make on the basis of this data can, invisibly, turn on these attributes, in ways that are as inscrutable as they are unethical.
What gets chosen is usually whatever is easiest to quantify, rather than the fairest.
Kate Crawford investigates algorithmic prejudice at Microsoft Research and co-founded the “AI Now” initiative, a research effort focusing on the dangers posed by artificial intelligence systems as they are being used today. She told me that a fundamental question in algorithmic fairness is the degree to which algorithms can be made to understand the social and historical context of the data they use. “You can tell a human operator to try to take into account the way in which data is itself a representation of human history,” Crawford says. “How do you train a machine to do that?” Machines that can’t understand context in this way will, at best, merely pass on institutionalized discrimination—what’s called “bias in, bias out.”
Incomplete efforts to redress hidden biases can make matters worse. Crawford’s colleague, Solon Barocas of Cornell University, has observed that end users can “uncritically accept the claims of vendors” that algorithms have been cleansed of prejudice. This is particularly true of applications, like the judicial system, where the status quo is rife with prejudice and there is great allure to the promise of more objective machines. Stripping away algorithmic bias can also require privileging one subjective definition of what it means to be fair over another—and what gets chosen is usually whatever is easiest to quantify, rather than the fairest.
For all of its pitfalls, though, finding and fighting bias in data and algorithms also comes with a sliver of opportunity: It can make the outlines of prejudice visible in new ways.
COMPAS is a piece of software used by courts throughout the United States. The program estimates how likely a defendant is to re-offend based on his or her response to 137 survey questions. This estimate is then used to inform bail decisions.
The COMPAS questionnaire doesn’t ask about skin color, heritage, or even ZIP code. But it does ask questions like whether a defendant lives in a neighborhood with “much crime,” and whether they have had trouble finding jobs that pay “more than minimum wage.” That these questions are more appropriately posed to a society, rather than an individual, underscores the bias in them: The answers are correlated with protected attributes, including race, which means that algorithms can learn to effectively “see” those attributes in the data. However, Northpointe, the company behind COMPAS, has claimed to have calibrated COMPAS so that the accuracy of its re-arrest predictions is independent of skin color.
In 2015, journalists from ProPublica set out to test this claim using the public records of one COMPAS customer, Broward County in Florida. They found that when COMPAS predicted that a defendant was high-risk, and that defendant was then actually re-arrested, its prediction was indeed colorblind in the most straightforward sense. But when COMPAS’ prediction was inaccurate (either predicting re-arrest when none happened, or not predicting an actual re-arrest) it routinely underestimated the probability of white recidivism and over-estimated the probability of black recidivism. In other words, it contained a bias hidden from the perspective of one set of statistics, but plainly visible in another.
ProPublica reported this finding in an article subtitled “There’s software used across the country to predict future criminals. And it’s biased against blacks.” Northpointe disputed their assessment, and responded with a statistical re-analysis of their claims. Northpointe shifted the argument away from the disparity in error rates, instead focusing on the fact that risk scores reflect an actual, underlying prevalence: More Afro-American defendants do in fact go on to be re-arrested. That means, they argued, that it is not surprising that they have higher risk scores as a population.
At the center of Northpointe’s argument was an essentialist fallacy: Because persons that police classified as Afro-Americans were re-arrested more often in the training dataset, they claimed, COMPAS is justified in predicting that other persons classified as Afro-Americans by police—even in a different city, state, and time period—are more likely to be re-arrested. The cycling of classification into data then back into classification echoes W.E.B. Dubois’ 1923 definition, “the black man is the man who has to ride Jim Crow in Georgia.”
One of the great benefits of the big data age is that the logic of our decisions can be formally analyzed, and numerically picked apart, in ways that were previously impossible.
While this episode illustrated many of the dangers of algorithmic decisions, it also produced a swell of academic research that came to a surprising conclusion: The very idea of assigning a risk score to a defendant entails a tradeoff between two different and incompatible definitions of the word “fair.” What’s more, this tradeoff is universal. “Any system that carries out this process will have this challenge,” Jon Kleinberg, a professor of computer science at Cornell, tells me, “whether it’s an algorithm or a system of human decision-makers.”
Kleinberg and his colleagues published a study proving that the two definitions of fairness used by Northpointe and ProPublica are mathematically incompatible. In technical terms, what they showed is that predictive parity (whether risk scores have the same overall accuracy for black and white defendants) and error rate balance (whether risk scores get the outcome wrong in the same ways, for different groups) are mutually exclusive. When the base rate of the outcome measure—re-arrest, in the case of COMPAS—is different between any two groups, applying the same standard to both groups will necessarily introduce error rate bias against the group with the higher base rate. “‘Calibration’ is what’s leading to this problem,” Kleinberg said. This is true of any system that uses risk scores—be it machine algorithm or human institution—no matter what factors it uses to generate them.
Remarkably, this incompatibility had never been shown before. Its discovery points to one of the great benefits of the big data age: The logic of our decisions can be formally analyzed, and numerically picked apart, in ways that were previously impossible. As a result, judges now know to consider these kinds of broader imbalances in the decisions they make. “The issues that ProPublica surfaced were really about how we think about prediction, as much as how we think about algorithms,” Kleinberg says.
Academics have also suggested how COMPAS might be fixed. Alexandra Chouldechova, professor of statistics and public policy at Carnegie Mellon University’s Heinz School, has shown that if COMPAS’ designers allow it to be slightly more inaccurate overall for Afro-American defendants, they can ensure that the algorithm makes mistakes at the same rate for different races. “This,” she observes, “may be a tradeoff you want to make.”
Google Translate has a hidden sexism. To see it, try translating the phrases “o bir doktor” and “o bir hemşire” from Turkish to English. Because the Turkish phrases use the gender-neutral pronoun “o,” Google Translate is forced to choose a gendered pronoun on its own. The result: It translates the first phrase to “he is a doctor,” and the second to “she is a nurse.”
The translation is the focus of a 2016 paper by Tolga Bolukbasi and colleagues at Boston University as an example of a type of language model known as word embedding. These models, used to power translation services, search algorithms, and autocomplete features, are trained on collected bodies of natural language (like Google News articles), usually without much intervention from human language experts. Words in the model are mapped as points in a high-dimensional space, so that the distance and direction between a given pair of words indicates how close they are in meaning and what semantic relationship they have.
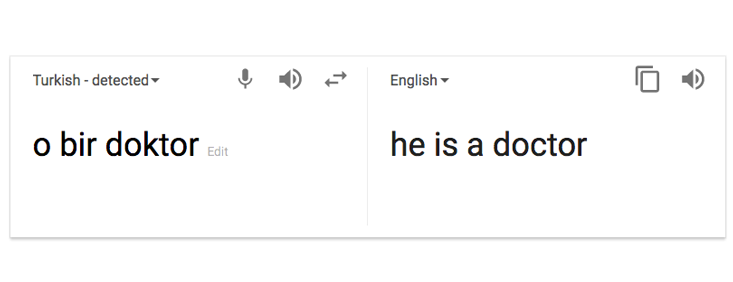
For example, the distance between “Man” and “Woman” is roughly the same, and in the same direction, as “King” is from “Queen.” Word embedding models can also perpetuate hidden biases, like those in the Google translation. The infrastructure, billions of texts collected in digital corpora over decades, is beginning to inform our daily communication in ways that are hard to understand—and to change. But many of the biases that infrastructure encodes predate their institutionalization in digital form. And, as with COMPAS, studying those biases as they appear in algorithms presents a new opportunity.
Bolukbasi and his colleagues have devised a technique for “de-biasing” language by moving words within the spaces of word embedding models. Imagine placing the words “doctor,” “nurse,” “man,” and “woman” onto the points of a square, with man and woman at the bottom and doctor and nurse at the top. The line connecting doctor and nurse is exactly parallel to that between man and woman. As a result, the system treats their relationship as analogous. Bolukbasi’s debiasing strategy pushes both doctor and nurse to the midpoint of the top edge so that “doctor” and “nurse” are the same distance from “man” and “woman.” The system has, in effect, “forgotten” the analogy; what pronoun the translation might then use is a choice left to the system designers.
In the best case, data infrastructure will force us to expose and confront our definitions of fairness and decision-making in ways that we might not have done without it.
The impact of shifting associations between words can be considerable. Arvind Narayanan, a professor of computer science at Princeton University, has developed a tool for measuring prejudice in machine learning models, together with colleagues Aylin Caliskan and Joanna Bryson. The trio began with a much-studied psychological measure called the Implicit Association Test. In a common variant of the test, the greater the speed with which subjects affirm association of positive words with words reflecting social categories, the greater their ease with that association. Across many such pairings, the average difference in response time—usually on the order of milliseconds—is a measure of the degree of implicit bias. Narayanan and colleagues swapped response time for distance between words, creating what they call a word embedding association test. The word embedding association test replicated the same set of stereotypes that Implicit Association Test studies have identified, on the same set of words.
Over two decades, the Implicit Association Test has exposed a wide variety of implicit biases, from gender to nationality to race, across populations and in many different contexts. Because prejudice is so pervasive, some have speculated natural human tendencies—for example to dominance hierarchies, and in-group identification—are responsible for these biases; in this view, prejudice is an inevitable fact of human nature. The authors of the word embedding association test paper speculate that their work supports another, though not exclusive, possibility: that “mere exposure to language contributes to these implicit biases in our minds.” In other words, if prejudices are reflected, and thus transmitted, in the statistics of language itself, then the way we speak doesn’t just communicate the way we view each other, it constructs it. If de-biasing projects like Bolukbasi’s can work, we can begin to shift our biases at scale and in a way that was previously impossible: with software. If they don’t, we face the danger of reinforcing and perpetuating those biases through a digital infrastructure that may last for generations.
The idea that you can do this at all is pretty cool,” Narayanan tells me. Still, he wonders how far it can go. He points out that Bolukbasi’s paper assumes that gender is binary, or at least that the connection between gendered words follows a straight line. “I don’t think [we] have any clue of how [debiasing] can work for a concept that is perhaps marginally more complex,” he cautions. He points in particular to racial stereotypes, where the very notion of categories is as problematic as the means used to define them.
When I ask Bolukbasi about this, he responds that the approach can in principle work with any number of categories, though he admits that it does require discrete categories, defined a priori. He used crowdworkers recruited from Amazon’s Mechanical Turk (a service billed as “artificial Artificial Intelligence”) to decide the categories in his gender work. The same workers also evaluated which analogies were biased, and how successful the program was in removing those biases. In other words, the decision about what is biased, and what it means for a bias to be removed, remains deeply tied to the median social consensus, encoding a populist brake on progress.
There are even more daunting concerns. Barocas and Crawford have recently pointed out that most work on fairness in algorithms has focused on what are known as “allocative harms”—the apportioning of resources, such as same-day service, or judgments, such as risk scores. They call for more attention to what critical race scholars like bell hooks term “representational harms.” A Google Image search for “CEO,” for instance, produces images that are overwhelmingly of white men. Narayanan says that these problems may be overlooked in discussions of fairness because “they are harder to formulate mathematically—in computer science, if you can’t study something in formal terms, its existence is not as legitimate as something you can turn into an equation or an algorithm.”
In the worst case, these and other limitations on our treatment of bias in data will make the algorithms we are building this generation’s concrete bridges, projecting the status quo forward for years to come. In the best case, data infrastructure will force us to expose and confront our definitions of fairness and decision-making in ways that we might not have done without it.
This tension is difficult to reconcile with our usual notions of technological progress. It’s tempting to presume that technology changes more quickly than society and that software can reinforce social progress by rapidly encoding new norms and insulating them from regressive or malicious actors. A sentencing algorithm can do less harm than a blatantly bigoted judge. But it can also obscure the history and context of bias and hinder, or even preclude, progress. Infrastructure is sticky and the window of opportunity is narrowing: Technology can improve in the future, but we’re making decisions about what tradeoffs to make now. It’s not clear how often, or even whether, we’ll get the opportunity to revisit those tradeoffs.
After all, the more pervasive algorithms become, the less likely they are to be replaced. While we might upgrade our phones every two years, there are strong impediments to renovating core software infrastructure. Consider how much dated technology already permeates our lives—air traffic control systems, for instance, run largely on software built in the 1970s. The recent “WannaCry” worm that crippled hospital systems across the United Kingdom exploited the fact that these systems ran on a decades-old version of Windows, which Microsoft wasn’t even bothering to maintain. A machine understanding of language, embedded in core services, could carry forward present prejudices for years or decades hence. In the words of artist Nicole Aptekar, “infrastructure beats intent.”
The greatest danger of the new digital infrastructure is not that it will decay, or be vulnerable to attack, but that its worst features will persist. It’s very hard to tear down a bridge once it’s up.
Aaron M. Bornstein is a researcher at the Princeton Neuroscience Institute. His research investigates how we use memories to make sense of the present and plan for the future.
Original Lead Image: Dmitriy Domino / Shutterstock