Sex is ubiquitous. The vast majority of animals and plants reproduce sexually at least some of the time. Some, such as humans, can reproduce no other way. Figuring out why sex is so common, though, has been a longstanding challenge for evolutionary biologists.
The problem is that, as a reproductive strategy, sex seems wasteful. The mere fact that you have survived to adulthood means that you are reasonably well adapted to your environment, and it is not at all clear that reshuffling your genes with those of someone else will lead to anything as good, let alone better. Furthermore, a female who reproduces asexually by making diploid eggs passes roughly twice as much of her genetic material on to the next generation as does one who reproduces sexually. Overall, cloning yourself would seem to be the way to go.
Sex and recombination generate new variation faster than mutation alone, and this might facilitate adaptation to an unpredictable environment. Is this sufficient, though, to overcome the short-term costs of sex to an individual? Through the middle and late 20th century, the answer seemed to be “no.” A number of population geneticists studied models in which a “modifier gene” determines whether or not the genome that contains it undergoes recombination (one allele, or variant, of the modifier gene allows for recombination; the other prevents it). Under most conditions, recombination declined in frequency and ultimately disappeared in these models. Furthermore, sex and recombination often reduced the rate of adaptive evolution. John Maynard Smith, surveying these models, concluded that while it was possible for recombination to be favored in an unpredictable environment, the environment would have to be “unpredictable in a special and somewhat implausible sense.”
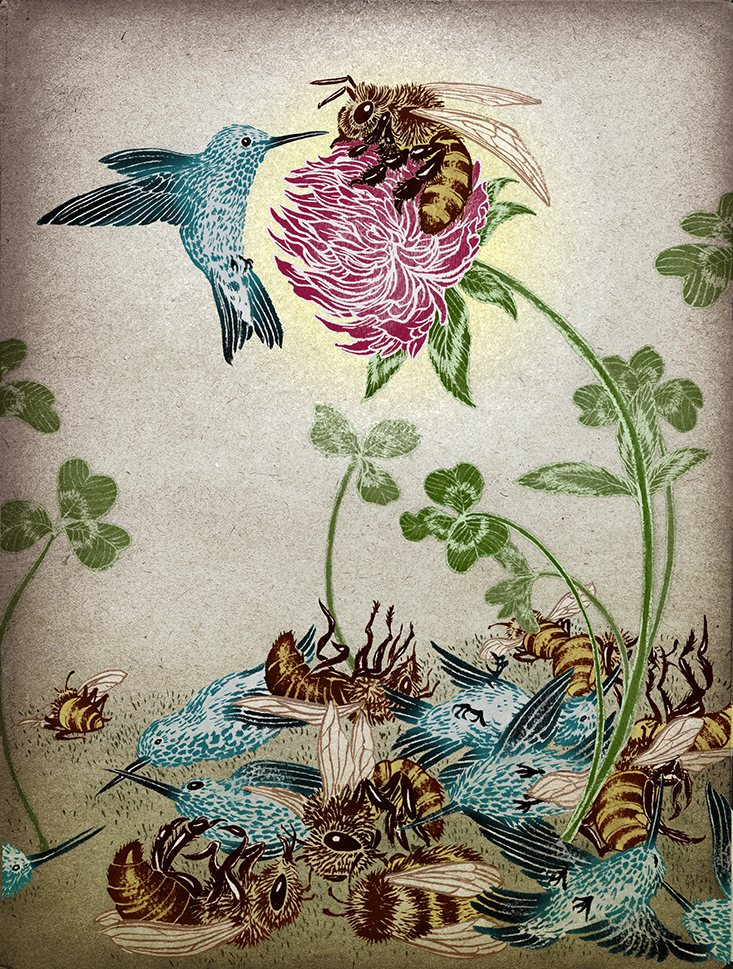
In fact, these models contained a simplifying assumption that, though seemingly innocuous, actually hid some key processes that make sex more likely to be adaptive. That assumption was that populations are infinitely large. In an infinite population with mutation, all possible combinations of alleles (variants of a particular gene) exist at any given time. If the environment changes, the optimal genotype will immediately start increasing in frequency—recombination just gets in the way.
In a finite population, though, not all combinations of alleles will be present at any given time. Furthermore, because of drift (see Understanding Drift), those combinations that are present will include some that are maladaptive. In recent years, researchers have focused increasingly on modeling finite populations in unpredictable environments. In such cases, most possible combinations of alleles are not initially present. Sex and recombination can create advantageous combinations by bringing together alleles that initially appeared in different individuals. When we further allow organisms to reproduce asexually or sexually depending on their circumstances, the value of recombination as a way to produce adaptive variants in an unpredictable environment reemerges as a viable explanation for the maintenance of sex.
This example illustrates a common mistake that we all make when thinking about things like waste and efficiency. We tend to intuitively treat the world as infinite and deterministic. When confronted with a world that we know is finite and sometimes random, we often uncritically think of it as a sloppy approximation to some “optimum” world; one that would exist were it not for pesky imperfections.
This modern remnant of Platonic essentialism—the idea that to gain true insight we should study an ideal world of perfect forms—sometimes prevents us from seeing that finite systems are not just fuzzy approximations to infinite systems (see Understanding Infinity). Rather, they behave in fundamentally different ways that we would never dream of if we thought only about idealized infinite worlds.
In evolutionary biology, this means that we discover entirely new kinds of processes when we study finite populations. The best studied of these probabilistic evolutionary processes is genetic drift; random fluctuations in allele frequencies. Drift is often visualized as a “random walk” in which, each generation, the frequency of a variant may take a step up (towards fixation), a step down (towards extinction), or remain the same (see Understanding Drift). The size of the step up or down is inversely proportional to the size of the population. In a large population, the size of the steps is small; in a small population, the step size is large (though this does not mean that drift is unimportant in large populations).
Adaptation thus involves multiple attempts, many unsuccessful, and even some steps in the wrong direction. In fact, adaptive evolution of finite populations requires error-prone exploration. The reason is that similar genomes can produce very different phenotypes with very different fitnesses. A useful analogy is the mountain climber searching for the highest peak of a jagged mountain range, but not able to look beyond his immediate surroundings. The simple approach of always stepping uphill will just get him stuck on a minor peak. The way around this is to occasionally step downhill—to sometimes accept solutions that are worse than the current one.
In fact, this is exactly the methodology behind a variety of modern computer algorithms, including genetic algorithms and simulated annealing. These methods find the optimal solution to a complex problem by sometimes accepting worse solutions than the current one. If the acceptance of inferior solutions is gradually reduced (to take fewer steps down as we climb higher), the algorithm eventually settles near the optimum. A computer with unlimited processing power and memory would not need to use genetic algorithms to solve complex problems. It could just compare all possible solutions side by side—just as an infinitely large population of humans would not need to rely on sexual reproduction to adapt to environmental changes. A computer with finite resources, on the other hand, must sometimes take one step back before it takes two steps forward.
We can recognize the same properties of finiteness in creative human endeavors such as art and science. The goal of science is to increase our knowledge and understanding of the objective qualities of the universe. The goal of art is to explore and communicate the subjective quality of individual experience. Because the universe—and our experience of living in it—is immensely complex, it is not surprising that scientists and artists both engage in a lot of exploration that leads to dead ends. An omniscient scientist or artist would not have to waste time on unsolvable equations, uninformative experiments, or failed metaphors.
If we think of unsuccessful variants as purely wasteful, it is because we are tacitly imagining infinite, idealized systems. In the real world of finite systems that must respond to complex challenges, error-prone exploration, combined with some form of selection, becomes not only a viable option, but a surprisingly efficient one—for everything from an egg to an abstract expressionist.
Sean Rice grew up in California and is now a professor of biological sciences at Texas Tech University. He is the author of Evolutionary Theory: Mathematical and Conceptual Foundations.