In January, Robert Williams, an African-American man, was wrongfully arrested due to an inaccurate facial recognition algorithm, a computerized approach that analyzes human faces and identifies them by comparison to database images of known people. He was handcuffed and arrested in front of his family by Detroit police without being told why, then jailed overnight after the police took mugshots, fingerprints, and a DNA sample.
The next day, detectives showed Williams a surveillance video image of an African-American man standing in a store that sells watches. It immediately became clear that he was not Williams. Detailing his arrest in the Washington Post, Williams wrote, “The cops looked at each other. I heard one say that ‘the computer must have gotten it wrong.’” Williams learned that in investigating a theft from the store, a facial recognition system had tagged his driver’s license photo as matching the surveillance image. But the next steps, where investigators first confirm the match, then seek more evidence for an arrest, were poorly done and Williams was brought in. He had to spend 30 hours in jail and post a $1,000 bond before he was freed.
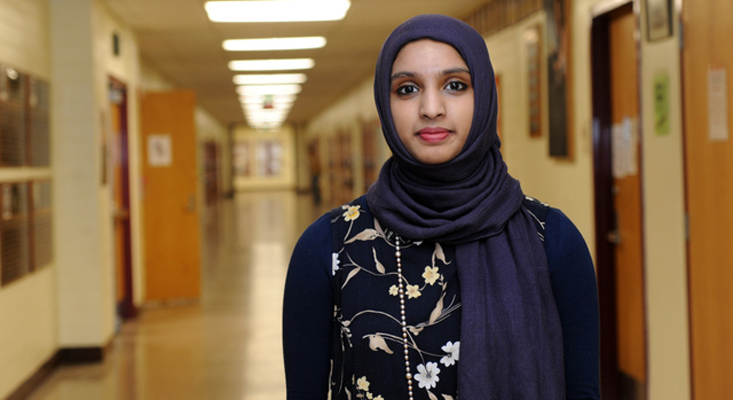
What makes the Williams arrest unique is that it received public attention, reports the American Civil Liberties Union.1 With over 4,000 police departments using facial recognition, it is virtually certain that other people have been wrongly implicated in crimes. In 2019, Amara Majeed, a Brown University student, was falsely identified by facial recognition as a suspect in a terrorist bombing in Sri Lanka. Sri Lankan police retracted the mistake, but not before Majeed received death threats. Even if a person goes free, his or her personal data remains listed among criminal records unless special steps are taken to expunge it.
Recent studies from the National Institute of Standards and Technology and the Massachusetts Institute of Technology2 have confirmed that computer facial recognition is less accurate at matching African-American faces than Caucasian ones. One reason for the discrepancy is the lack of non-Caucasian faces in datasets from which computer algorithms form a match. The poor representation of people of color from around the world, and their range of facial features and skin shades, creates what researchers have called a “demographic bias” built into the technology.
Facial recognition technology has widespread effects through its association with broad surveillance and massive stores of photographs. In the 1920s, investigators began wiretapping telephones to trace criminal activities. In the 1970s, analog closed-circuit television added remote visual monitoring of people. But digital methods vastly expand the power and scale of surveillance through cameras linked to the Internet and police departments. Ubiquitous in homes, businesses, and public spaces, a billion cameras are projected to be placed in over 50 countries by 2021, one for every eight people on Earth.
To identify suspects, the FBI and police compare images from surveillance cameras and other sources to photo databases. These contain some criminal mugshots, but the bulk of the images comes from non-criminal sources such as passports and state driver’s license compilations; that is, the databases mostly expose ordinary, generally innocent citizens to criminal investigation. This approach grew after 9/11, when the United States government proposed Total Information Awareness, a global program to collect data about people and identify them by various means, including facial recognition. Georgetown University’s Center on Privacy and Technology asserts that half of American adults, 117 million people, appear in databases accessible to police.3 In 2019, testimony before the U.S. House Oversight Committee revealed that the FBI can scan 640 million photos for facial matching.4
“The cops looked at each other. I heard one say that ‘the computer must have gotten it wrong.’ ”
The FBI and police scan these masses of photos through computer programs that digitize them for identification. An important thread in developing this technology began with the American mathematician and AI pioneer Woodrow Wilson “Woody” Bledsoe. In 1959, he and a colleague invented a machine to recognize alphanumeric characters, then went on to facial recognition.
Their first idea was to analyze a character, say the letter “A,” by overlaying it onto a rectangular array of pixels. Each pixel received a binary 1 or 0 depending on whether or not it contained part of the image. The pixels were sampled in adjacent groups called “n-tuples” to account for the spatial relations among them. Further manipulation produced a set of binary digits embodying the letter “A.” This process found and stored the bits and a resulting unique score for every character; then an unknown character was identified by comparing its score to the values in memory. The method worked, correctly recognizing up to 95 percent of handwritten and printed numerals.
N-tuples, however, did poorly for the intricacies of a face, whose appearance also varies with illumination, tilt of the head, facial expression, and the subject’s age. Bledsoe’s team turned instead to human operators who measured characteristic parameters from photographs of faces, such as the distance between the pupils of the eyes or from top to bottom of an ear.5 In 1967, the researchers showed that a computer using stored facial measurements from several thousand photographs reduced by 99 percent the number of images a person would have to sift through to match a new photo. Then in 1973, Japanese computer scientist Takeo Kanade automated the entire process with a computer program that extracted eyes, mouth, and so on, from an image of a face without human intervention.
Bledsoe’s foundational facial recognition work was funded by the Department of Defense, or according to some evidence, the CIA, either of which would have limited his freedom to publish his results.5 But early this year, writer Shaun Raviv described in Wired what he learned from examining Bledsoe’s life and an archive of his work given to the University of Texas after Bledsoe’s death in 1995.6 The recognition experiments, Raviv reported, began with a database of photos of 400 male Caucasians. In the archive, Raviv saw no references to women or people or color, or images of them in dozens of marked-up photos that must represent Bledsoe’s facial measurements.
Since Bledsoe’s original research, other techniques have arisen, supported by more powerful computers and bigger databases to develop and test algorithms. Now the introduction of AI methods is bringing about the latest changes; but the bias that comes from the lack of diversity in Bledsoe’s formative datasets still appears, and for much the same reason, in these advanced methods.
For years, the U.S. National Institute of Standards and Technology (NIST) has invited producers of facial recognition algorithms to submit them for testing. In 2019, NIST presented its analysis of 189 algorithms from 99 mostly commercial developers.7 These were checked against federal databases with 18 million images of 8.5 million people for general accuracy and across different demographic groups, in two applications: 1:1 matching, where a face is compared to a stored image for verification, as in confirming the validity of a passport, and 1:n matching, where a face is compared to a whole dataset, typically to find a criminal suspect. For each algorithm, the researchers determined the number of false negatives, where a face that should be matched to one in the database is not, and false positives, where a face is matched to the wrong one.
The data show that facial recognition has improved significantly. The rate of failing to match a submitted face to one in the database dropped from 4 percent in 2014 to only 0.2 percent by 2018. Newer algorithms were also less sensitive to the variations in facial appearance that plagued early efforts. The NIST researchers ascribe these gains to an “industrial revolution” in facial recognition, the adoption of deep convolutional neural networks (CNN).
One test yielded a false positive rate 63 times higher for African faces than for European ones.
A neural network is a computing system that can be taught to carry out certain tasks, somewhat like the connected neurons in a biological brain. A CNN mimics human visual perception. In our brains, neurons in specialized regions of the visual cortex register certain general elements in what the eyes see, such as the edges of objects, lines tilted at particular angles, and color. The brain assembles these results into a meaningful whole that allows a person, for example, to quickly recognize a friend even under obscured or varied conditions.
As in the n-tuple method, in a CNN the pixels forming an image are analyzed in spatially adjacent clumps, but succeeding stages provide deeper analysis. Like the regions in the brain, each stage seeks different types of general pictorial elements like those the brain finds, rather than seeking the eyes, nose, and so on. The mathematically manipulated results are passed on and augmented through the stages, finally producing an integrated representation of a face. Crucially, this is achieved by first exposing the CNN to a large dataset of varied facial images. This “trains” the system to develop a comprehensive approach to analyzing faces.
Within NIST’s testing, CNN-based algorithms performed best; but overall, the algorithms differed in how well they identified people of different races, sexes, and ages. These results echo earlier studies of 1:1 matching and are the first to explore demographic effects in 1:n matching. Errors in each application yield different undesirable outcomes. A false positive in a 1:1 search can allow unauthorized access; a false positive in a 1:n search for a criminal suspect puts the subject at risk for unwarranted accusations.
In 1:n matching, the NIST data show that the most accurate algorithms are also the most reliable across demographic groups. Less proficient ones give higher rates of false positives for African-American females compared to African-American males, and to white males and females, in an FBI database of 1.6 million mugshots. For 1:1 matching, some algorithms falsely matched African-American and Asian faces 10 to 100 times more often than Caucasian ones. Notably, however, some algorithms from Asian countries gave fewer false positives for Asians than for Caucasians. This, the report notes, shows that the degree of diversity in a training dataset may strongly affect the demographic performance of a CNN.
“Facial recognition should not be used to deprive people of liberty.”
Other research has more fully explored how lack of diversity affects the training of a neural network. In 2012, B.F. Klare and A.K. Jain at Michigan State University and colleagues tested 1:1 facial matching against police mugshots.8 Different types of algorithms they examined were all less accurate for African-American faces than white or Hispanic ones. One algorithm studied was a neural network defined by its training dataset. The researchers found that the resulting fits to African-Americans improved when this dataset was limited to African-American faces, and in a nod to diversity, also improved when the training dataset had equal numbers of African-American, Hispanic and white faces.
This suggests how to make biased training databases more equitable. In one recent demonstration, researchers at the biometrics company Onfido made a demographically unbalanced dataset less biased.9 Its facial images came from different continents in varying proportions, such as 0.5 percent from Africa compared to 61 percent from Europe. This yielded a false positive rate 63 times higher for African faces than for European ones. But when the researchers used statistical methods to train with more African faces than their small numbers alone would provide, the discrepancy was reduced to a factor of 2.5, a sign of future possibilities.
But according to biometrician Patrick Grother, lead scientist for the NIST report, serious police action should require more than just a match from an algorithm. He explained that an algorithm actually returns a list of likely candidates. In the ideal next step, an investigator seeking suspects must confirm that there is a good match within this list. Only then would the detective seek other evidence like eyewitnesses or forensic data to justify arresting and charging the subject. The fact that a “no match” from a human investigator can overturn a wrong machine identification should be reassuring, but that came too late to save Williams from false arrest and its repercussions.
Andrew Guthrie Ferguson is a professor at American University Washington College of Law who studies technology and civil rights. Responding to my query, he wrote that “facial recognition should not be used to deprive people of liberty.” It is “too dangerous a tool to be used in an unregulated way. Williams’ case is a signal to stop the ad hoc adoption of facial recognition before an injustice occurs that cannot be undone.”
Repairing the flaws in facial recognition technology will not be easy within a complex landscape that includes dozens of producers of the software with varying levels of bias, and thousands of law enforcement agencies that can choose any of these algorithms. Maybe only a federal effort to establish standards and regulate compliance to them would be necessary before we no longer have a Robert Williams, a member of any minority group, or any citizen unjustly experience a night in jail or worse.
Sidney Perkowitz, Candler Professor of Physics Emeritus at Emory University, has written about police algorithms and is working on a book about them. His latest books are Physics: A Very Short Introduction and Real Scientists Don’t Wear Ties.
References
1. Garvie, C. The untold number of people implicated in crimes they didn’t commit because of face recognition. aclu.org/news (2020).
2. Buolamwini, J. & Gebru, T. Gender shades: Intersectional accuracy disparities in commercial gender classification. Proceedings of Machine Learning Research 81, 1-15 (2008).
3. Garvie, C., Bedoya, A., & Frankle, J. The perpetual line-up. Georgetown Law Center on Privacy & Technology (2016).
4. Melton, M. Government Watchdog Questions FBI On Its 640-Million-Photo Facial Recognition Database. Forbes (2019).
5. Boyer, R.S. (Ed.) Automated Reasoning: Essay in Honor of Woody Bledsoe Kluwer Academic Publishers, Dordrecht, Netherlands (1991).
6. Raviv, S. The Secret History of Facial Recognition. Wired (2020).
7. Grother, P., Ngan, M., & Hanaoka, K. Face recognition vendor test. National Institute of Standards and Technology (2018).
8. Klare, B.F., Burge, M.J., Klontz, J.C., Vorder Bruegge, R.W., & Jain, A.K. Face recognition performance: Role of demographic information. IEEE Transactions on Information Forensics and Security 7, 1789-1801 (2012).
9. Bruveris, M., Mortazavian, P., Gietema, J., & Mahadevan, M. Reducing geographic performance differentials for face recognition. arXiv (2020). Retrieved from DOI: 2002.12093
Lead image: greenbutterfly /Shutterstock