In 1975, the British economist Charles Goodhart pointed out that when a measure becomes a target, it ceases to be a good measure. Goodhart’s Law, as it came to be known, is a ubiquitous phenomenon in regulatory affairs, like healthcare. Making healthcare better requires metrics which can be measured and assessed. But measuring the right metric is sometimes the wrong choice.
In order to quantify and characterize health and healthcare, hospitals and government agencies collect massive amounts of data. Typically, this data is gathered by patient surveys, such as the Hospital Consumer Assessment of Healthcare Providers and Systems Survey (HCAHPS), or by the hospital itself (for instance regarding in-hospital mortality rate). The metrics present in these surveys are usually easy to measure—they’re partly used exactly for that reason. Government agencies, in turn, demand improvement in metrics like mortality rate or hospital readmission rate. Hospitals focus on these scores, which can be coupled to financial penalties and loss of patients. This procedure is supposed to financially incentivize hospitals to improve the healthcare system. And this is exactly where the trouble starts.
One problem is the potential for “gaming the system.” By nominally fulfilling requirements set by the regulators, the regulated actors can pursue their own aims and effectively weaken the connection between the metric and the regulator’s goal (improved healthcare). One classic example comes from 18th century Britain. At that time, taxation was proportional to the number of windows in a given house. Initially, this was a reasonable choice, as the number of windows tended to increase with house size and was easily measurable. But when people started bricking up some of their windows (you can still see the bricks today) in order to pay lower taxes, the metric (number of windows) and the goal (to tax people according to the size of their house) became misaligned. Goodhart’s Law was rearing its head.
Like Heisenberg’s uncertainty principle in quantum mechanics, the assessment of performance metrics as regulatory targets changes their statistical relationship with health.
Does Goodhart’s Law constitute a problem in healthcare, too? Unfortunately, the answer is a resounding “yes.” Two intuitive metrics—in-hospital mortality and readmission rate—serve as an ideal illustration. Both of these measures correlate with health (intuitively as well as statistically, once rates are adjusted for socioeconomic status) and are therefore regularly chosen as performance metrics to assess hospitals. But then hospital administrators quickly found roundabout ways to satisfy regulators.
One approach was refusing to admit the elderly or the very sick, so that mortality rates would drop. Risky procedures were avoided for the same reason, even if they were necessary. As a result, some populations of patients either received reduced care or no care at all, defeating the purpose of the policy. Another popular performance metric is “readmission rate 30 days after hospital discharge.” Again, ideally this metric would be a stand-in for the quality of care, as a lower rate of readmission could indicate that the health issue was solved during the initial hospital stay. But this does not hold true for patients with chronic diseases, as they usually require multiple readmissions. Yet when regulators tried to enforce this measure as a performance metric, hospitals simply delayed readmissions to day 31 after hospital discharge or even discouraged readmission altogether. Perversely, this resulted in a higher mortality rate (which incidentally also results in a lower readmission rate) and a lower standard of care, especially for patients with chronic diseases.
The list of misapplied performance metrics could go on and on. Recently, studies discovered a link between patient satisfaction (captured by patient surveys and Yelp ratings) and health metrics such as mortality rate and readmission rate. Patient satisfaction is also one of the factors positively influencing insurance payments, creating another incentive for hospitals. But maximizing patient satisfaction as a regulatory target will not necessarily result in an improved healthcare system—unless you think that HD television, a lobby with free drinks, or a jacuzzi will improve your health. Likewise, directives to reduce waiting times in U.K. emergency rooms to four hours did not reduce overall mortality. The ERs favored younger patients over older patients, redefined what counts as waiting time and redistributed hospital staff from other departments (whose waiting times increased).
Forcing the abstract concept of health into the straitjacket of easy-to-measure attributes leads to a loss of information. Like Heisenberg’s uncertainty principle in quantum mechanics, the assessment of performance metrics as regulatory targets changes their statistical relationship with health. One fix might be calculating a score from a plethora of performance metrics—this increases the chances of a continuous correlation with health, as the score will remain useful even if one or two metrics are corrupted by cheating.
However, this would only work if the importance of each metric is roughly similar: A group of metrics completely dominated by a single metric is not more robust to cheating than one metric by itself. Metrics could also be kept secret from the entity being evaluated, though this lack of transparency would create its own problems.
The least that can be done is simply this: Be aware of Goodhart’s Law, and cast a sceptical eye at that latest healthcare metric.
Daniel Bojar is a PhD student at the Swiss Federal Institute of Technology in Zurich, in the Department of Biosystems Science and Engineering. Follow him on Twitter @daniel_bojar.
WATCH: The moral boundary between using genetics to prevent disease and enhance ability is constantly shifting.
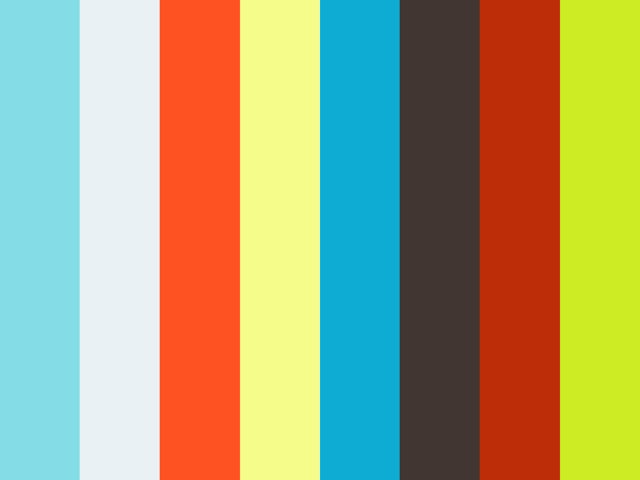