Having the sense to take a shortcut, the most direct route from point A to point B, doesn’t sound like a very impressive test of intelligence. Yet according to a new report appearing today in Nature, in which researchers describe the performance of their new navigational artificial intelligence, the system’s ability to explore complex simulated environments and find the shortest route to a goal put it in a class previously reserved for humans and other living things.
The surprising key to the system’s performance was that while learning how to navigate, the neural net spontaneously developed the equivalent of “grid cells,” sets of brain cells that enable at least some mammals to track their location in space.
For neuroscientists, the new work seems to offer important clues about how grid cells in living brains make us better navigators. It also shows how neural nets could contribute greatly to future neuroscience studies: Neil Burgess, a cognitive neuroscientist at University College London who was not involved with the study, suggested that the systems should “provide fertile ground for understanding how and why the human brain works as it does.”
Meanwhile, for AI researchers, the work’s relevance to improving automated navigation systems is obvious. But its greater importance might eventually lie in suggesting a more general way to enhance the intelligence of machines.
According to the researchers Andrea Banino at the British AI company DeepMind and Caswell Barry at University College London, who were lead authors on the new Nature paper, the project evolved out of questions they had about the function of the brain’s grid cells. Grid cells are often called “the brain’s GPS” because of their importance to navigation in many animal species. (Edvard Moser and May-Britt Moser shared a 2014 Nobel Prize for their discovery of grid cells nine years earlier.) These clusters of neurons, which are arranged in roughly hexagonal arrays, collectively work like the inertial guidance systems on ships, aircraft, and missiles: They keep track of how the body is moving through space, even in total darkness. “It’s basically updating your belief about where you are based on how you’re moving,” Barry said.
“It really is a demonstration that the benefits of using deep learning can extend to tasks that are more like higher cognitive functions.”
Neuroscientists have therefore credited grid cells with performing the function called “path integration”—the dead-reckoning form of navigation that doesn’t involve external cues: for example, “Take five steps forward, turn 90 degrees to the left, then walk straight ahead for another 15 steps. …” But various experiments have also hinted that grid cells perform other functions, including some that might go beyond navigation. For example, some studies implicate grid cells in measuring time and distance during travel. As Barry noted, if grid cells provide a spatial reference for objects and locations, “then in principle you can use them to calculate the direct route between those places”—that is, what’s called “vector-based navigation.”
The researchers decided to use deep-learning neural networks to investigate the role of grid cells in these navigational functions. As a first step, they set up a neural net to learn how to do path integration for a simulated agent moving through a small space. “We wanted to see whether we could set up an artificial network with an appropriate task so that it would actually develop grid cells,” Barry said.
The neural net obliged, and, according to Barry, “what was surprising was how well it worked.” The “grid units” that spontaneously emerged in the network were remarkably similar to what’s seen in animals’ brains, right down to the hexagonal grid.
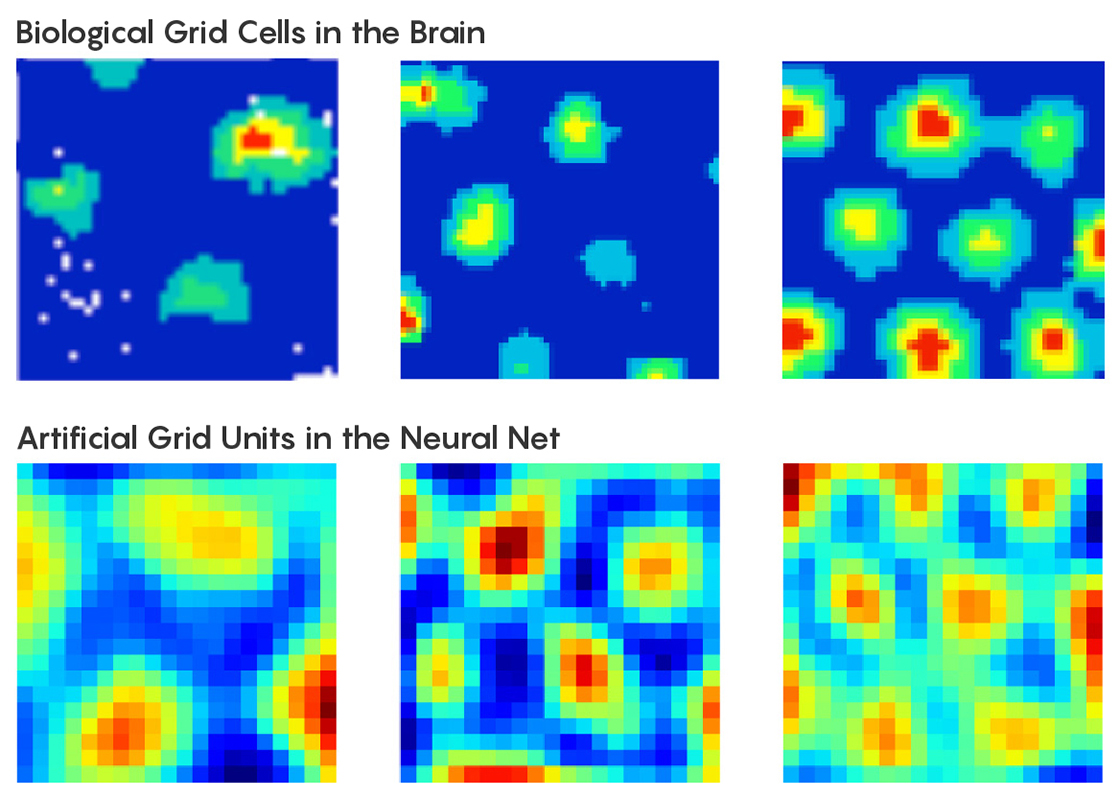
The research team then joined the neural network’s abilities to systems that helped simulated agents find their way through mazelike virtual environments to goals. The system with grid units was far superior to systems without: For example, the system could tell if a previously closed door offered a shortcut to the goal, and it would take that route preferentially. According to Banino, this knack demonstrated that the grid units in the neural net were performing vector-based navigation because they were identifying a shorter, more direct route based on knowledge of the goal’s position.
“I think with this work, we were able to give a proof of principle that grid cells are used for taking shortcuts,” Banino said. The results therefore supported theories that grid cells in the brain are capable of both path integration and vector-based navigation. Comparable experimental proof with studies on living animals, he added, would be much more difficult to obtain.
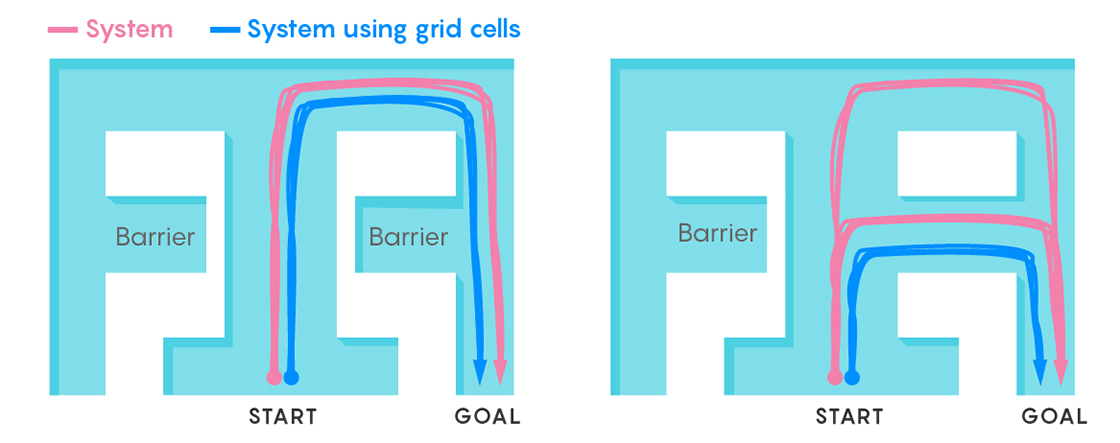
“The interesting implication is that this same approach could be used for different sorts of neuroscience questions,” Barry said. Researchers interested in limb control, for example, could train a neural network to control a robotic arm the way that the brain controls a living arm, and then run experiments on the artificial system to generate further insights into the living one. “It’s a potentially very exciting general-purpose neuroscience tool.”
“It’s quite spectacular,” said Stefan Leutgeb, a professor of neurobiology at the University of California, San Diego. “I think they make a very good case for vector navigation being a possible function [of grid cells]. This has long been proposed, but I don’t think anyone has come as close to finding a possible mechanism.”
But at the same time, he notes, “It has the limits of any computational study. This is a way in which it could work, but it doesn’t prove that it’s the way it works in animals.”
“The brain is the only example we have of a general-purpose algorithm. So why not source from it?”
Francesco Savelli, a neuroscientist at Johns Hopkins University who co-authored a commentary accompanying the new Nature paper, offered a similar view. He considers it very interesting that “you somehow get these [grid] cells without programming them. … And still they come out, as emergent properties.” But at the same time, “because it’s not a biologically realistic system, then you might be a little less clear on exactly what information it gives you.
“Unless you’re able to break into the black box of this network, and make this network a little more biologically realistic, then at some point you hit the limit in how you can inform further neurobiological studies,” he said.
On the other hand, what’s encouraging from a technological perspective is that “these deep learning systems, more and more, can tackle tasks that are more similar to higher cognitive function of the brain,” Savelli said. “It really is a demonstration that the benefits of using deep learning can extend to tasks that are more like higher cognitive functions.”
One might imagine that the DeepMind researchers would be looking to use this better navigational network to improve, say, the capabilities of exploratory robots or future self-piloting drones. But according to Banino, their plans are less focused—and more ambitious. “We think navigation is a fundamental aspect of our intelligence,” he said. “Personally, we’re not thinking of any application other than creating a general-purpose algorithm.
“The brain is the only example we have of a general-purpose algorithm,” he continued. “So why not source from it?”
Jordana Cepelewicz contributed reporting to this story.
Lead image credit: kmarfu