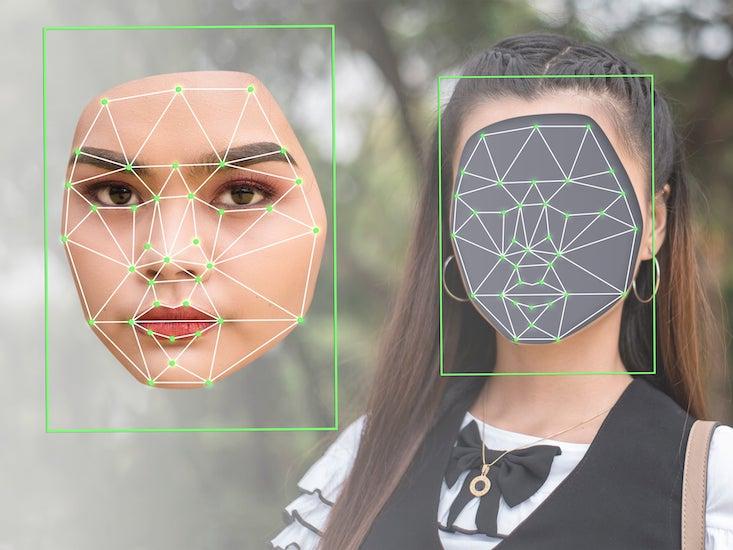
For many of us over the last year and more, our waking experience has, you might say, lost a bit of its variety. We spend more time with the same people, in our homes, and go to fewer places. Our stimuli these days, in other words, aren’t very stimulating. Too much day-to-day routine, too much familiarity, too much predictability. At the same time, our dreams have gotten more bizarre. More transformations, more unrealistic narratives. As a cognitive scientist who studies dreaming and the imagination, this intrigued me. Why might this be? Could the strangeness serve some purpose?
Maybe our brains are serving up weird dreams to, in a way, fight the tide of monotony. To break up bland regimented experiences with novelty. This has an adaptive logic: Animals that model patterns in their environment in too stringent a manner sacrifice the ability to generalize, to make sense of new experiences, to learn. AI researchers call this “overfitting,” fitting too well to a given dataset. A face-recognition algorithm, for example, trained too long on a dataset of pictures might start identifying individuals based on trees and other objects in the background. This is overfitting the data. One way to look at it is that, rather than learning the general rules that it should be learning—the various contours of the face regardless of expression or background information—it simply memorizes its experiences in the training set. Could it be that our minds are working harder, churning out stranger dreams, to stave off overfitting that might otherwise result from the learning we do about the world every day?
Yet how often do you dream of being at a computer?
Erik Hoel, a Tufts University neuroscientist and author of The Revelations, a cerebral novel about consciousness (excerpted in Nautilus), thinks it’s plausible. He recently published a paper, “The overfitted brain: Dreams evolved to assist generalization,” laying out his reasoning. “Mammals are learning all the time. There’s no shut-off switch,” Hoel told me. “So it becomes very natural to assume that mammals would face the problem of overlearning, or learning too well, and would need to combat that with some sort of cognitive homeostasis. And that’s the overfitted brain hypothesis: that there is homeostasis going on wherein the effects of the learning of the organism is constantly trending in one direction, and biology needs to fight it to bring it back to a more optimal setpoint.”
What’s distinctive about Hoel’s idea in the field of dream research is that it provides not only a cause of the weirdness of dreams, but a purpose, too. Other accounts of dreaming don’t really address why dreams get weird, or just write them off as a kind of by-product of other processes. They get away with this by noting that truly weird dreams are rare: It is easy to overestimate how weird our dreams really are. Although we tend to remember better the weird dreams, careful studies show that around 80 percent of our dreams reflect normal activity, and can be downright boring.
The “continuity hypothesis,” emphasizing this, suggests that dreams are just replays of plausible versions of waking life. To its credit, most of our dreams, though not most of the dreams we remember, fall into this category. But the continuity hypothesis doesn’t explain why we dream more about some things than others. For example, many if not most of us spend an enormous amount of time in front of screens—working, playing, watching movies, reading. Yet how often do you dream of being at a computer? The continuity hypothesis would suggest that the proportion of activities in dreams would reflect their proportions in waking life, and this clearly doesn’t happen.
Another set of theories holds that dreams are there to help you practice for real-world events. These theories are generally supported by the findings that sleep, and dreams in particular, seem to be important for learning and memory. Antti Revonsuo, a cognitive neuroscientist at the University of Skövde, in Sweden, came up with two theories of this nature. The threat simulation theory accounts for why 70 percent of our dreams are distressing. It holds that the function of dreams is to practice for dangerous situations. Later he broadened this to suggest that dreams are for practicing social situations in general. These learning theories also provide an explanation for why we believe that what we see in dreams is really happening: If we didn’t, we might not take them seriously, and our ability to learn from them would be diminished.1
Another theory accounts for the weirdness as being a side-effect of the brain activity. The “random activation theory” suggests that dreams are our forebrains trying to make sense of the random activity sent to it by the chaotic and meaningless information coming from the back of the brain during sleep. On this view, the weirdness has no function. On the other hand, the brain stem’s random activity might not be meaningless. McGill University neuroscientist Barbara Jones has noted that this part of the brain is used for programmed movements, like having sex and running, and these activities are frequently represented in dreams.
Hoel faces the weirdness of dreams head on. His hypothesis doesn’t deal with it indirectly but gives the weirdness significance. It helps keep the brain from doing something that plagues machine-learning researchers: overfitting. Stopping learning is one way to deal with overfitting—paying too much attention to insignificant details of a training set. But there are others, and many of the main ways to combat it introduce noise, often with distorted versions of the input. This, in effect, makes the “deep learning” neural network not so sure about the importance of the idiosyncrasies of the training set, and more likely to focus on generalities that will end up working better in the real world. So, for Hoel, dreams are weird because they’re serving the same function: They provide distorted input to keep the brain from overfitting to the “training set” of its waking experiences.
Interestingly, overfitting has been shown to happen in people in laboratory experiments—and sleep removes the overfitting. In short, dreams are weird because they need to be. If they were too similar to waking life they would exacerbate overfitting, not eliminate it. Even the dreams that are realistic usually don’t exactly match the episodes that happened to us—they’re different takes on the activities we do in life.
Like other learning accounts of dreaming, Hoel’s hypothesis holds that sleep is the perfect time to do “offline” learning. Experiencing warped or distorted inputs would be distracting and dangerous if they happened while we were awake. And perhaps the function of forgetting so many of our dreams is so that we don’t mistake them for things that actually happened. The mind wants to train its neural net parameters, not create new episodic memories for us to confuse with reality.
I asked Hoel whether we might look to machine learning to get hypotheses about how weird dreams would optimally be in a human being. “Possibly, but I would very much like to go the other way,” he said. “Maybe there’s something from neuroscience that deep learning should pay attention to. You want incoming data that is different enough that it is out of distribution in the classic sense, but it is not so different or wild that you don’t know what to do with it.”
This all suggests that there’s some optimal level of weirdness that dreams should have. Unfortunately, weirdness is not an easy thing to measure. “It’s almost like art or literature,” Hoel said. “A good poem is not completely nonsense, but also not just I saw the flower / the flower was blue. It’s occupying some critical space where things morph and change with the use of metaphor, but not so much so that it’s totally unrecognizable or alien.” He went on, “Maybe that Lynchian distance is precisely what helps big, complex minds the most when it comes to these serial problems of overlearning and over-memorization and overfitting.”
Neural networks were inspired by brain architecture, but since the deep-learning movement, these AIs have mostly been used to simply create smarter machines, not to model and understand human thought. But more and more, findings in deep learning are inspiring new theories of how our brains work. Neural networks need to “dream” of weird, senseless examples to learn well.
Maybe we do, too.
Jim Davies is a professor at the Department of Cognitive Science at Carleton University. He is co-host of the award-winning podcast Minding the Brain. His new book is Being the Person Your Dog Thinks You Are: The Science of a Better You.
Footnote
1. A brain explanation for why we accept the reality of our dreams is that our dorsolateral prefrontal cortex (DLPFC) is (relatively) deactivated during dreaming. This part of the brain is used, in part, to detect anomalies in the world. This theory is strengthened by the fact that the DLPFC is more active during lucid dreaming, which is characterized by an awareness of being in a dream.