You’ve probably heard the myth that the average person uses only 10 percent of their brain. It’s a seductive lie because it suggests that we could be more than we are. Sci-fi movies like Limitless and Lucy, whose protagonists gain super-human abilities by accessing latent mental capacities, have exploited the myth. Neuroscientists, on the other hand, have long loathed it. Eighty years of studies confirm that every part of the brain is active throughout the course of a day. Save those who have suffered serious brain injury, we use all of our brains, all of the time.
But, like many legends, the 10 percent myth also carries a grain of truth. In the last 20 years, scientists have discovered that our cortex follows a strangely familiar pattern: A small minority of neurons output the vast majority of activity. It’s not that we don’t use 90 percent of our brain, but that many neurons remain eerily quiet even during use. The story behind this silence is more profound than the boosted IQs and temporary clairvoyance from the movies. It speaks to the basic principles of how our minds represent reality in the first place.
Neurons communicate with electrical impulses called spikes. In the 1930s, scientists began to record spikes from individual neurons using small metal electrodes inserted into the brain. They observed neurons with activity rates of tens to hundreds of spikes per second, with each spike lasting a few milliseconds.1 The brain seemed to be buzzing with communication. Then in a 1968 review of microelectrode technology, the biomedical engineer David Robinson brought an important discrepancy to light. As electrodes are lowered into the brain, they should detect activity from any cell they come close to. In a typical recoding, this would theoretically amount to about 200 cells. Yet researchers were lucky to record from five cells per electrode insertion. Where were the rest of the neurons?
Many brushed these concerns aside—the low discovery rate might be due to tissue damage from the electrode, or because the recordings were made while the animal subjects were anesthetized. But Robinson’s insight was vindicated 30 years later as researchers began to make painstaking recordings from the inside of cells.1-3 They found that the majority of neurons in the cortex spike much less than the commonly reported 10-100 spikes per second, and that these high rates describe only the most active 3 to 20 percent of cells. It turns out that scientists had long failed to record from the majority of neurons in the brain, simply because they lacked the methods to detect them.
A small population of neurons fire hundreds or thousands of times more often than others.
Today we know that a large population of cortical neurons are “silent.” They spike surprisingly rarely, and some do not spike at all. Since researchers can only take very limited recordings from inside human brains (for example, from patients in preparation for brain surgery), they have estimated activity rates based on the brain’s glucose consumption. The human brain, which accounts for less than 2 percent of the body’s mass, uses 20 percent of its calorie budget, or three bananas worth of energy a day. That’s remarkably low, given that spikes require a lot of energy. Considering the energetic cost of a single spike and the number of neurons in the brain, the average neuron must spike less than once per second.4 Yet the cells typically recorded in human patients fire tens to hundreds of times per second, indicating a small minority of neurons eats up the bulk of energy allocated to the brain. The remaining neurons may fire a few times per minute or less. This energy budget puts limits on how much of the cortex can be engaged at once: In total, no more than 1 percent of neurons can be active. This may explain why our attention is so limited in scope—our brains can only allocate so many spikes to any given perception.
Why does the brain keep around a huge number of relatively inactive cells? The question is a hard one because while we know that spikes translate into our perception of the world, we’re still far from knowing how.5 This translation is referred to as the neural code, and neuroscientists have been trying to crack it for the past 100 years.
There are two extremes of neural coding: Perceptions might be represented through the activity of ensembles of neurons, or they might be encoded by single neurons. The first strategy, called the dense code, would result in a huge storage capacity: Given N neurons in the brain, it could encode 2N items—an astronomical figure far greater than the number of atoms in the universe, and more than one could experience in many lifetimes. But it would also require high activity rates and a prohibitive energy budget, because many neurons would need to be active at the same time. The second strategy—called the grandmother code because it implies the existence of a cell that only spikes for your grandmother—is much simpler. Every object in experience would be represented by a neuron in the same way each key on a keyboard represents a single letter. This scheme is spike-efficient because, since the vast majority of known objects are not involved in a given thought or experience, most neurons would be dormant most of the time. But the brain would only be able to represent as many concepts as it had neurons.
Theoretical neuroscientists struck on a beautiful compromise between these ideas in the late ’90s.6,7 In this strategy, dubbed the sparse code, perceptions are encoded by the activity of several neurons at once, as with the dense code. But the sparse code puts a limit on how many neurons can be involved in coding a particular stimulus, similar to the grandmother code. It combines a large storage capacity with low activity levels and a conservative energy budget.
View Video
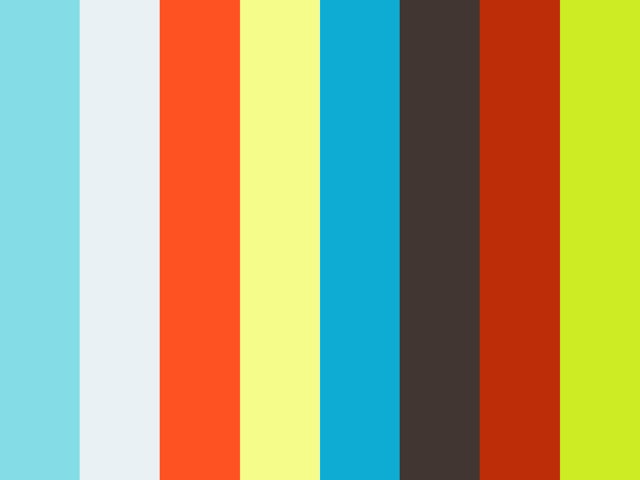
Sparse coding is considered one of the great triumphs of theoretical neuroscience, as its predictions are in stunning agreement with real data. In a 1996 paper, the neuroscientists Bruno Olshausen and David Field trained an artificial neural network to “learn” images. Their network was constrained to a sparse code by limiting the number of neurons active at any given time, and requiring that the information each neuron encoded be maximally independent from that encoded by other neurons. Under these constraints, the artificial neurons extracted information from images in the exact way that real neurons in the visual cortex do: by spiking for local edges with a particular form. In the brain, neuroscientists believe these edges first reassemble into shapes and textures, then eventually into the perceptions of specific concepts like faces, bowties, and boats. The visual world gets ripped apart into its primary elements before meaning is layered on as information rises through increasingly specialized brain regions.
The sparse coding strategy explains why some neurons spike extremely rarely: They encode very specific information. But it doesn’t explain why a small population of neurons fire hundreds or thousands of times more often than others. This can partially, but not fully, be explained by the diversity of cell types—some neuron classes simply spike more than others. But even among excitatory cells, the most common neuron class, there is a dramatic activity imbalance. This disparity might represent two separate coding strategies: A small, active population might make a rapid “best guess” for enabling more immediate responses, while the quiet population refines this into a specific perception. Think of all the times you thought you caught an ominous figure out of the corner of your eye, only to realize it was a coat rack.
The quiet neurons might be doing more than refining perceptions. Though they spike infrequently, we know from intracellular recordings that they still receive inputs from other neurons, which causes their membrane voltages to fluctuate. The sum of these fluctuations and spikes constitute what’s commonly known as brain waves. Over the past 15 years scientists have begun to amass evidence that these brain waves play an active role in information processing, shunting some neural inputs while enhancing others, for example, or altering the timing of spikes. This suggests that spikes are not the sole information-carrying signal in the brain, and that, in turn, the “inactive” neurons are doing much more than it seems.
In addition to shaping the output of high-spiking neurons, these quiet neurons could be a kind of “reserve pool” for learning and recovery. A mouse that loses one whisker becomes more sensitive to his remaining whiskers, because neurons in the touch-related cortical region rewire to receive inputs from the spared whiskers. When scientists recorded neural activity before and after trimming a mouse whisker, they found that it was the less active neurons that became more sensitive to spared whiskers.8
If this is true, we really do have latent mental capabilities, as suggested by the 10 percent myth. They are more prosaically referred to as learning.
Kelly Clancy studies neuroscience as a postdoctoral fellow at the University of Basel, in Switzerland. Previously, she roamed the world as an astronomer and served with the Peace Corps in Turkmenistan. She won the 2014 Regeneron Prize for Creative Innovation for her work designing drug-free brain therapies.
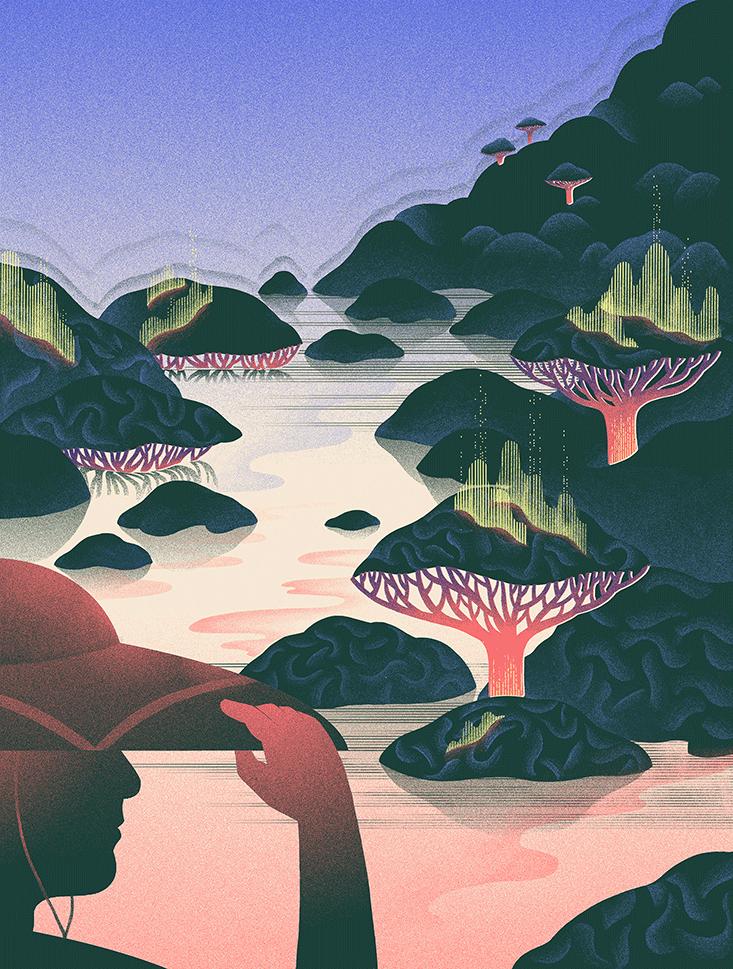
References
1. Shoham, S., O’Connor, D.H., & Segev, R. How silent is the brain: Is there a “dark matter” problem in neuroscience? Journal of Comparative Physiology A 192, 777–784 (2006).
2. Henze, D.A., et al. Intracellular features predicted by extracellular recordings in the hippocampus in vivo. Journal of Neurophysiology 84, 390–400 (2000).
3. de Kock, C.P., Bruno, R.M., Spors, H., & Sakmann, B. Layer- and cell-type-specific suprathreshold stimulus representation in rat primary somatosensory cortex. The Journal of Physiology 581, 139–154 (2007).
4. Lennie, P. The cost of cortical computation. Current Biology 13, 493–497 (2003).
5. Olshausen, B.A. & Field, D.J. How close are we to understanding v1? Neural Computation 17, 1665–1699 (2005).
6. Olshausen, B.A. & Field, D.J. Emergence of simple-cell receptive field properties by learning a sparse code for natural images. Nature 381, 607–609 (1996).
7. Bell, A. J. & Sejnowski, T. J. The “independent components” of natural scenes are edge filters. Vision Research 37, 3327–3338 (1997).
8. Margolis, D.J. et al. Reorganization of cortical population activity imaged throughout long-term sensory deprivation. Nature Neuroscience 15, 1539–1546 (2012).