Reprinted with permission from Quanta Magazine‘s Abstractions blog.
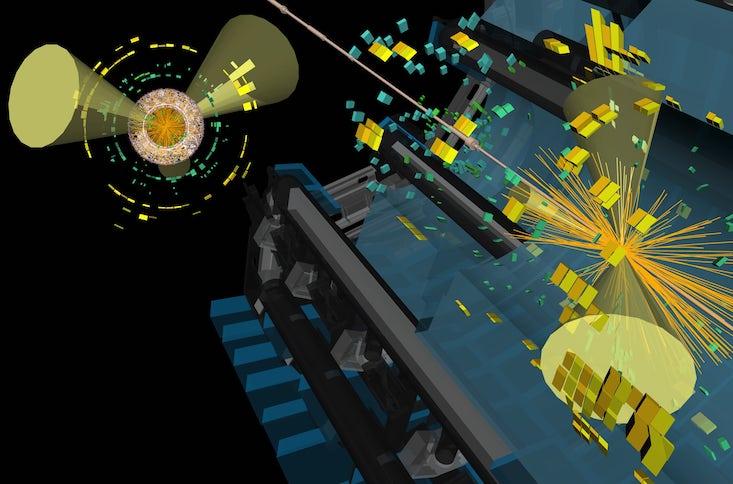
The Large Hadron Collider (LHC) smashes a billion pairs of protons together each second. Occasionally the machine may rattle reality enough to have a few of those collisions generate something that’s never been seen before. But because these events are by their nature a surprise, physicists don’t know exactly what to look for. They worry that in the process of winnowing their data from those billions of collisions to a more manageable number, they may be inadvertently deleting evidence for new physics. “We’re always afraid we’re throwing the baby away with the bathwater,” said Kyle Cranmer, a particle physicist at New York University who works with the ATLAS experiment at CERN.
Faced with the challenge of intelligent data reduction, some physicists are trying to use a machine learning technique called a “deep neural network” to dredge the sea of familiar events for new physics phenomena.
In the prototypical use case, a deep neural network learns to tell cats from dogs by studying a stack of photos labeled “cat” and a stack labeled “dog.” But that approach won’t work when hunting for new particles, since physicists can’t feed the machine pictures of something they’ve never seen. So they turn to “weakly supervised learning,” where machines start with known particles and then look for rare events using less granular information, such as how often they might take place overall.
In a paper posted on the scientific preprint site arxiv.org in May, three researchers proposed applying a related strategy to extend “bump hunting,” the classic particle-hunting technique that found the Higgs boson. The general idea, according to one of the authors, Ben Nachman, a researcher at the Lawrence Berkeley National Laboratory, is to train the machine to seek out rare variations in a data set.
Consider, as a toy example in the spirit of cats and dogs, a problem of trying to discover a new species of animal in a data set filled with observations of forests across North America. Assuming that any new animals might tend to cluster in certain geographical areas (a notion that corresponds with a new particle that clusters around a certain mass), the algorithm should be able to pick them out by systematically comparing neighboring regions. If British Columbia happens to contain 113 caribous to Washington state’s 19 (even against a background of millions of squirrels), the program will learn to sort caribous from squirrels, all without ever studying caribous directly. “It’s not magic but it feels like magic,” said Tim Cohen, a theoretical particle physicist at the University of Oregon who also studies weak supervision.
By contrast, traditional searches in particle physics usually require researchers to make an assumption about what the new phenomena will look like. They create a model of how the new particles will behave—for example, a new particle might tend to decay into particular constellations of known particles. Only after they define what they’re looking for can they engineer a custom search strategy. It’s a task that generally takes a Ph.D. student at least a year, and one that Nachman thinks could be done much faster, and more thoroughly.
The proposed CWoLa algorithm, which stands for Classification Without Labels, can search existing data for any unknown particle that decays into either two lighter unknown particles of the same type, or two known particles of the same or different type. Using ordinary search methods, it would take the LHC collaborations at least 20 years to scour the possibilities for the latter, and no searches currently exist for the former. Nachman, who works on the ATLAS project, says CWoLa could do them all in one go.
Other experimental particle physicists agree it could be a worthwhile project. “We’ve looked in a lot of the predictable pockets, so starting to fill in the corners we haven’t looked in is an important direction for us to go in next,” said Kate Pachal, a physicist who searches for new particle bumps with the ATLAS project. She batted around the idea of trying to design flexible software that could deal with a range of particle masses last year with some colleagues, but no one knew enough about machine learning. “Now I think it might be the time to try this,” she said.
The hope is that neural networks could pick up on subtle correlations in the data that resist current modeling efforts. Other machine learning techniques have successfully boosted the efficiency of certain tasks at the LHC, such as identifying “jets” made by bottom-quark particles. The work has left no doubt that some signals are escaping physicists’ notice. “They’re leaving information on the table, and when you spend $10 billion on a machine, you don’t want to leave information on the table,” said Daniel Whiteson, a particle physicist at the University of California, Irvine.
Yet machine learning is rife with cautionary tales of programs that confused arms with dumbbells (or worse). At the LHC, some worry that the shortcuts will end up reflecting gremlins in the machine itself, which experimental physicists take great pains to intentionally overlook. “Once you find an anomaly, is it new physics or is it something funny that went on with the detector?” asked Till Eifert, a physicist on ATLAS.
Charlie Wood is a journalist covering developments in the physical sciences both on and off the planet. His work has appeared in Scientific American, The Christian Science Monitor and LiveScience, among other publications. Previously, he taught physics and English in Mozambique and Japan, and he has a bachelor’s in physics from Brown University.