What if physiologists were the only people who study human behavior at all scales: from how the human body functions, to how social norms emerge, to how the stock market functions, to how we create, share, and consume culture? What if neuroscientists were the only people tasked with studying criminal behavior, designing educational curricula, and devising policies to fight tax evasion?
Despite their growing influence on our lives, our study of AI agents is conducted this way—by a very specific group of people. Those scientists who create AI agents—namely, computer scientists and roboticists—are almost exclusively the same scientists who study the behavior of AI agents.
We cannot certify that an AI agent is ethical by looking at its source code, any more than we can certify that humans are good by scanning their brains.
As these computer scientists and roboticists create their agents to solve particular tasks—no small feat—they most often focus on ensuring that their agents fulfill their intended function. For this, they use a variety of benchmark datasets and tasks that make it possible to compare different algorithms objectively and consistently. For example, email classification programs should meet a benchmark of accuracy in classifying email to spam or non-spam using some “ground truth” that is labeled by humans. Computer vision algorithms must correctly detect objects in human-annotated image datasets like ImageNet. Autonomous cars must navigate successfully from A to B in a variety of weather conditions. Game playing agents must defeat state-of-the-art algorithms, or humans who won a particular honor—such as being the world champion in chess, Go, or poker.
This task-specific study of the behavior of AI agents, while narrow, is extremely useful for the progress of the fields of AI and robotics. It enables rapid comparison between algorithms based on objective, widely-accepted criteria. But is it enough for society?
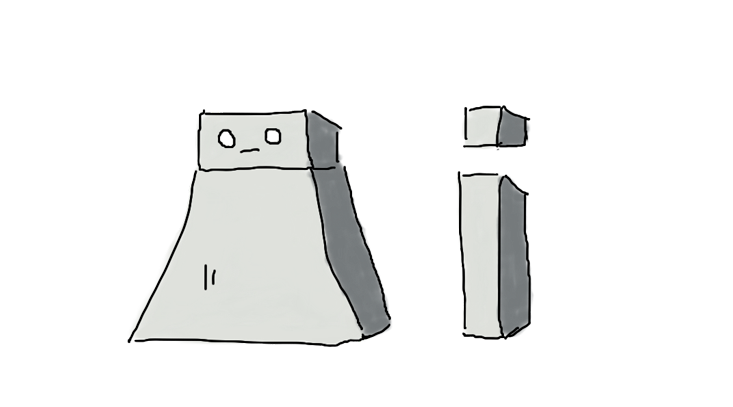
The answer is no: The study of the behavior of an intelligent agent (human or artificial) must be conducted at different levels of abstraction, in order to properly diagnose problems and devise solutions. This is why we have a variety of disciplines concerned with the study of human behavior at different scales. From physiology to sociology, from psychology to political science, and from game theory to macroeconomics, we obtain complementary perspectives on how humans function individually and collectively.
In his landmark 1969 book, Sciences of the Artificial, Nobel Laureate Herbert Simon wrote: “Natural science is knowledge about natural objects and phenomena. We ask whether there cannot also be ‘artificial’ science—knowledge about artificial objects and phenomena.” In line with Simon’s vision, we advocate the need for a new, distinct scientific discipline of Machine Behavior: the scientific study of behavior exhibited by intelligent machines.
This new discipline is concerned with the scientific study of machines, not as engineering artifacts, but as a new class of actors with their unique behavioral patterns and ecology. Crucially, this field overlaps with, but is distinct from computer science and robotics, as it treats machine behavior observationally and experimentally, without necessarily appealing to the machine’s internal mechanisms. Machine Behavior is akin to how the fields of animal behavior—also known as ethology—and behavioral ecology study the behavior of animals without necessarily focusing on physiology or biochemistry.
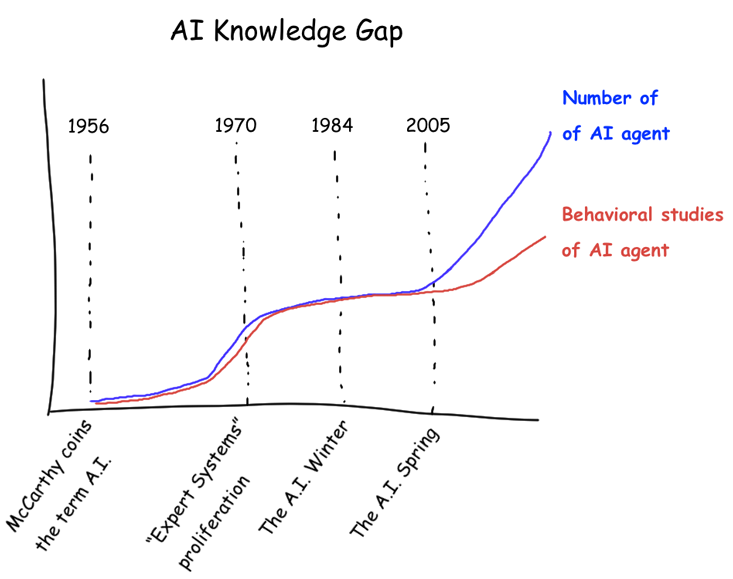
Our definition of the new field of Machine Behavior comes with some caveats. Studying machine behavior does not imply that AI algorithms have agency—in the sense that they are socially responsible for their actions. If someone’s dog bites a bystander, it is the owner of the dog who is held responsible. Nonetheless, it is useful to study—and therefore effectively predict—dog behavior. Similarly, machines are embedded in a larger socio-technical fabric, with human stakeholders who are responsible for deploying them, and for the harm they may cause to others.
Complex AI agents often exhibit inherent unpredictability: they demonstrate emergent behaviors that are impossible to predict with precision—even by their own programmers.
A second caveat is that machines exhibit behaviors that are fundamentally different from animals and humans, so we must avoid any tendency to excessively anthropomorphize or zoomorphize machines. Even if borrowing scientific methods from the study of human and animal behavior may prove useful for the study of machines, machines may exhibit forms of intelligence and behavioral patterns that are qualitatively different—even alien.
With those caveats out of the way, we argue that a distinct new discipline concerned with studying machine behavior will be both novel and useful. There are many reasons we cannot study machines by simply looking at their source code or internal architecture. First is opacity caused by complexity: Many new AI architectures, like Deep Neural Networks, can exhibit internal states that are not easily interpretable. We often cannot certify that an AI agent is optimal or ethical by looking at its source code, any more than we can certify that humans are good by scanning their brains. Furthermore, what we can consider ethical and good for society today can gradually change over time, something that social scientists are able to track but the AI community may fail to keep up with, since it is not how their achievements are measured.
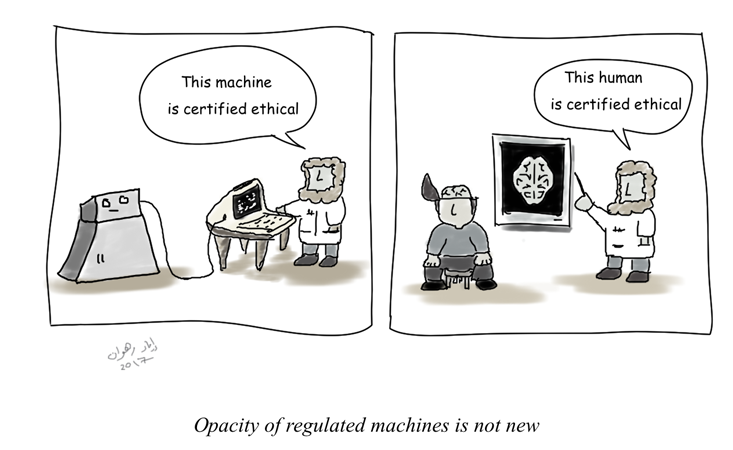
Another motivation for the study of machine behavior without appeal to its internal architecture is opacity caused by intellectual property protection. Many algorithms in common use—such as news filtering and product recommendation algorithms—are black boxes simply due to industrial secrecy. This means that the internal mechanisms driving these algorithms are opaque to anyone outside of the corporations that own and operate them.
Third, complex AI agents often exhibit inherent unpredictability: they demonstrate emergent behaviors that are impossible to predict with precision—even by their own programmers. These behaviors manifest themselves only through interaction with the world and with other agents in the environment. This is certainly the case for algorithmic trading programs, which can exhibit previously unseen behavioral regimes through complex market dynamics. In fact, Alan Turing and Alonzo Church showed the fundamental impossibility of ensuring an algorithm fulfills certain properties without actually running said algorithm. There are fundamental theoretical limits to our ability to verify that a particular piece of code will always satisfy desirable properties, unless we execute the code, and observe its behavior.
But most importantly, the scientific study of Machine Behavior by those outside of computer science and robotics provides new perspectives on important economic, social, and political phenomena that machines influence. Computer scientists and roboticists are some of the most talented people on earth. But they simply do not receive formal training in the study of phenomena like racial discrimination, ethical dilemmas, stock market stability, or the spread of rumors in social networks. While computer scientists have studied such phenomena, there are capable scientists from other disciplines, with important skills, methods, and perspectives to bring to the table. In our own work—being computer scientists ourselves—we’ve been frequently humbled by our social and behavioral science collaborators. They often highlighted how our initial research questions were ill-formed, or how our application of particular social science methods was inappropriate—e.g. missing important caveats or drawing conclusions that are too strong. We have learned—the hard way—to slow down our impulse to just crunch big data or build machine learning models.
Under the status quo, contributions from commentators and scholars outside of computer science and robotics are often poorly integrated. Many of these scholars have been raising alarms. They are concerned about the broad, unintended consequences of AI agents that can act, adapt, and exhibit emergent behaviors that often defy their creators’ original intent—behaviors that may be fundamentally unpredictable.
In tandem with this lack of predictability, there is fear of potential loss of human oversight over intelligent machines. Some have documented many cases in which seemingly benign algorithms can cause harm to individuals or communities. Others have raised concerns about AI systems being black boxes whose decision-making rationales are opaque to those affected, undermining their ability to question such decisions.
Artificially intelligent machines increasingly mediate our social, economic, and political interactions—and this is the tip of the iceberg of what is to come.
While these important voices have made great strides in educating the public about the potential adverse effects of AI systems, they still operate from the sidelines—and tend to generate internal backlash at the AI community. Furthermore, much of this contemporary discussion relies on anecdotal evidence gathered by specialists, and limited to specific cases. We still lack a consolidated, scalable, and scientific approach to the behavioral study of artificial intelligence agents in which social scientists and computer scientists can collaborate seamlessly. As Cathy O’Neil wrote in The New York Times: “There is essentially no distinct field of academic study that takes seriously the responsibility of understanding and critiquing the role of technology—and specifically, the algorithms that are responsible for so many decisions—in our lives.” And given the pace at which new AI algorithms are proliferating, this gap—between the number of deployed algorithms and our understanding of those algorithms—is only likely to increase over time.
Finally, we anticipate that substantial economic value can be unlocked by studying machine behavior. For example, if we can certify that a given algorithm satisfies certain ethical, cultural, or economic standards of behavior, we may be able to market it as such. Consequently, consumers and responsible corporations may start demanding such certification. This is akin to the way consumers have started demanding certain ethical and environmental standards be met in the supply chains that produce the goods and services they consume. A science of machine behavior can lay the foundation for such objective certification for AI agents.
Artificially intelligent machines increasingly mediate our social, economic, and political interactions: Credit scoring algorithms determine who can get a loan; algorithmic trading programs buy and sell financial assets on the stock market; algorithms optimize dispatch in local policing; programs for algorithmic sentencing now influence who is given parole; autonomous cars drive multi-ton boxes of metal in our urban environments; robots map our homes and perform regular household cleaning; algorithms influence who gets matched with whom in online dating; and soon, lethal autonomous weapons may determine who lives and who dies in armed conflict. And this is the tip of the iceberg of what is to come. In the near future, software and hardware agents driven by artificial intelligence (AI) will permeate every aspect of society.
Whether or not they have anthropomorphic features, these machines are a new class of agents—some even say, species—that inhabit our world. We must use every tool at our disposal to understand and regulate their impact on the human race. If we do this right, perhaps, humans and machines can thrive together in a healthy, mutualistic relationship. To make that possibility a reality, we need to tackle machine behavior head on with a new scientific discipline.
Iyad Rahwan is an associate professor of media arts and sciences at the MIT Media Lab, and the director and principal investigator of its Scalable Cooperation group.
Manuel Cebrian is a research scientist with the MIT Media Lab, and the research manager of its Scalable Cooperation group.