The first episode of Sesame Street in 1969 included a segment called “One of These Things Is Not Like the Other.” Viewers were asked to consider a poster that displayed three 2s and one W, and to decide—while singing along to the game’s eponymous jingle—which symbol didn’t belong. Dozens of episodes of Sesame Street repeated the game, comparing everything from abstract patterns to plates of vegetables. Kids never had to relearn the rules. Understanding the distinction between “same” and “different” was enough.
Machines have a much harder time. One of the most powerful classes of artificial intelligence systems, known as convolutional neural networks or CNNs, can be trained to perform a range of sophisticated tasks better than humans can, from recognizing cancer in medical imagery to choosing moves in a game of Go. But recent research has shown that CNNs can tell if two simple visual patterns are identical or not only under very limited conditions. Vary those conditions even slightly, and the network’s performance plunges.
These results have caused debate among deep-learning researchers and cognitive scientists. Will better engineering produce CNNs that understand sameness and difference in the generalizable way that children do? Or are CNNs’ abstract-reasoning powers fundamentally limited, no matter how cleverly they’re built and trained? Whatever the case, most researchers seem to agree that understanding same-different relations is a crucial hallmark of intelligence, artificial or otherwise.
“Not only do you and I succeed at the same-different task, but a bunch of nonhuman animals do, too—including ducklings and bees,” said Chaz Firestone, who studies visual cognition at Johns Hopkins University.
The ability to succeed at the task can be thought of as a foundation for all kinds of inferences that humans make. Adam Santoro, a researcher at DeepMind, said that the Google-owned AI lab is “studying same-different relations in a holistic way,” not just in visual scenes but also in natural language and physical interactions. “When I ask an [AI] agent to ‘pick up the toy car,’ it is implied that I am talking about the same car we have been playing with, and not some different toy car in the next room,” he explained. A February 2021 survey of research on same-different reasoning also stressed this point. “Without the ability to recognize sameness,” the authors wrote, “there would seem to be little hope of realizing the dream of creating truly intelligent visual reasoning machines.”
Same-different relations have dogged neural networks since at least 2013, when the pioneering AI researcher Yoshua Bengio and his co-author, Caglar Gulcehre, showed that a CNN could not tell if groups of blocky, Tetris-style shapes were identical or not. But this blind spot didn’t stop CNNs from dominating AI. By the end of the decade, convolutional networks had helped AlphaGo beat the world’s best Go player, and nearly 90% of deep-learning-enabled Android apps relied on them.
Getting any machine to learn same-different distinctions may require a breakthrough in the understanding of learning itself.
This explosion in capability reignited some researchers’ interest in exploring what these neural networks couldn’t do. CNNs learn by roughly mimicking the way mammalian brains process visual input. One layer of artificial neurons detects simple features in raw data, such as bright lines or differences in contrast. The network passes these features along to successive layers, which combine them into more complex, abstract categories. According to Matthew Ricci, a machine-learning researcher at Brown University, same-different relations seemed like a good test of CNNs’ limits because they are “the simplest thing you can ask about an image that has nothing to do with its features.” That is, whether two objects are the same doesn’t depend on whether they’re a pair of blue triangles or identical red circles. The relation between features matters, not the features themselves.
In 2018, Ricci and collaborators Junkyung Kim and Thomas Serre tested CNNs on images from the Synthetic Visual Reasoning Test (SVRT), a collection of simple patterns designed to probe neural networks’ abstract reasoning skills. The patterns consisted of pairs of irregular shapes drawn in black outline on a white square. If the pair was identical in shape, size and orientation, the image was classified “same”; otherwise, the pair was labeled “different.”
The researchers found that a CNN trained on many examples of these patterns could distinguish “same” from “different” with up to 75% accuracy when shown new examples from the SVRT image set. But modifying the shapes in two superficial ways—making them larger, or placing them farther apart from each other—made the CNNs’ accuracy go “down, down, down,” Ricci said. The researchers concluded that the neural networks were still fixated on features, instead of learning the relational concept of “sameness.”
In 2020, Christina Funke and Judy Borowski of the University of Tübingen showed that increasing the number of layers in a neural network from six to 50 raised its accuracy above 90% on the SVRT same-different task. However, they didn’t test how well this “deeper” CNN performed on examples outside the SVRT data set, as Ricci’s group had. So the study didn’t provide any evidence that deeper CNNs could generalize the concepts of same and different.
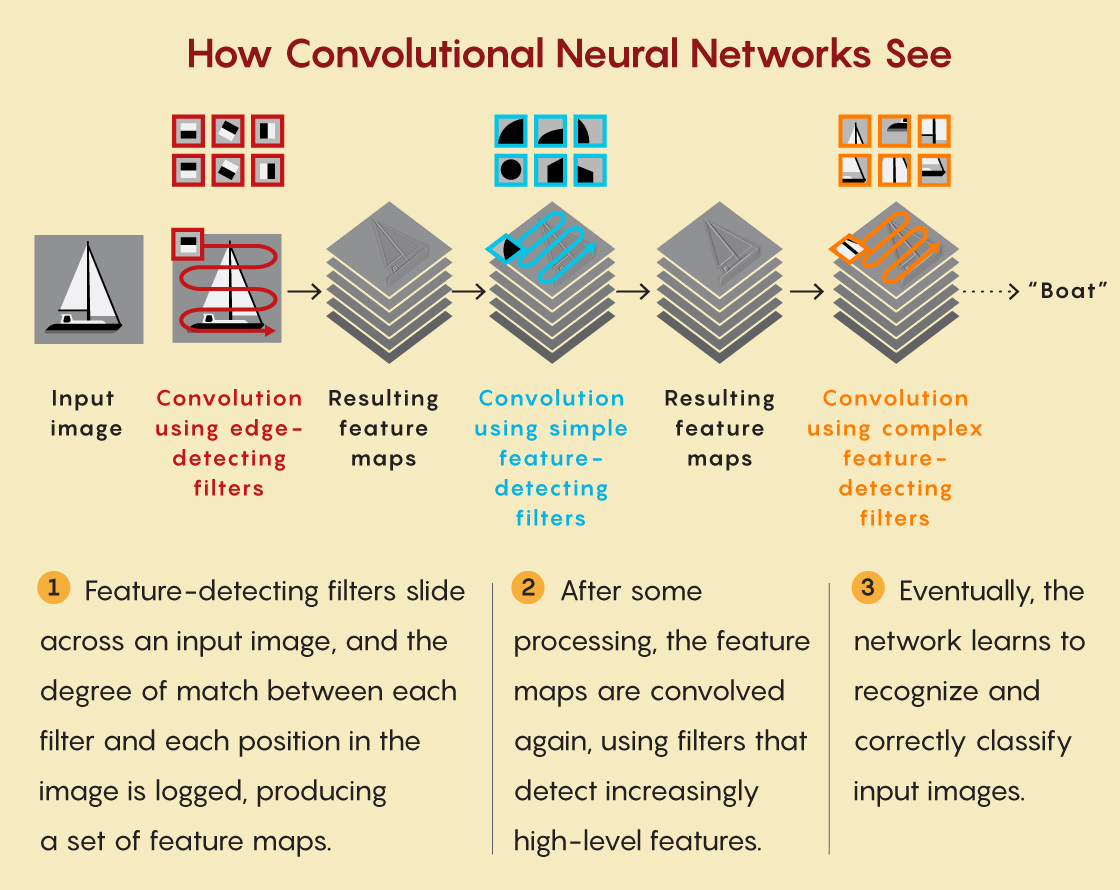
Guillermo Puebla and Jeffrey Bowers, cognitive scientists at the University of Bristol, investigated in a follow-up study in 2021. “Once you grasp a relation, you can apply it to whatever comes to you,” said Puebla. CNNs, he maintains, should be held to the same standard.
Puebla and Bowers trained four CNNs with various initial settings (including some of the same ones used by Funke and Borowski) on several variations of the SVRT same-different task. They found that subtle changes in the low-level features of the patterns—like changing the thickness of a shape’s outline from one pixel to two—was often enough to cut a CNN’s performance in half, from near perfect to barely above chance.
What this means for AI depends on whom you ask. Firestone and Puebla think the results offer empirical evidence that current CNNs lack a fundamental reasoning capability that can’t be shored up with more data or cleverer training. Despite their ever-expanding powers, “it’s very unlikely that CNNs are going to solve this problem” of discriminating same from different, Puebla said. “They might be part of the solution if you add something else. But by themselves? It doesn’t look like it.”
Funke agrees that Puebla’s results suggest that CNNs are still not generalizing the concept of same-different. “However,” she said, “I recommend being very careful when claiming that deep convolutional neural networks in general cannot learn the concept.” Santoro, the DeepMind researcher, agrees: “Absence of evidence is not necessarily evidence of absence, and this has historically been true of neural networks.” He noted that neural networks have been mathematically proved to be capable, in principle, of approximating any function. “It is a researcher’s job to determine the conditions under which a desired function is learned in practice,” Santoro said.
Ricci thinks that getting any machine to learn same-different distinctions will require a breakthrough in the understanding of learning itself. Kids understand the rules of “One of These Things Is Not Like the Other” after a single Sesame Street episode, not extensive training. Birds, bees and people can all learn that way—not just when learning to tell “same” from “different,” but for a variety of cognitive tasks. “I think that until we figure out how you can learn from a few examples and novel objects, we’re pretty much screwed,” Ricci said.
Lead image Credit: Samuel Velasco/Quanta Magazine