When Nate Soares psychoanalyzes himself, he sounds less Freudian than Spockian. As a boy, he’d see people acting in ways he never would “unless I was acting maliciously,” the former Google software engineer, who now heads the non-profit Machine Intelligence Research Institute, reflected in a blog post last year. “I would automatically, on a gut level, assume that the other person must be malicious.” It’s a habit anyone who’s read or heard David Foster Wallace’s “This is Water” speech will recognize.
Later Soares realized this folly when his “models of other people” became “sufficiently diverse”—which isn’t to say they’re foolproof, he wrote in the same post. “I’m probably still prone to occasionally believing someone is malicious when they’re merely different than me, especially in cases where they act similarly to me most of the time (thereby fooling my gut-level person-modeler into modeling them too much like myself).” He suspected that this “failure mode” links up with the “typical mind” fallacy, and is “therefore,” he concluded, “difficult to beat in general.”
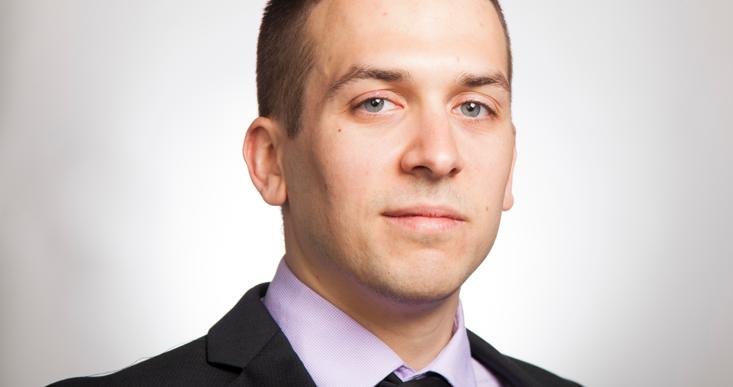
Beating biases is one of Soares’ core concerns—“I care a lot about having accurate beliefs,” he says—and one of them is the bias that artificial intelligence will just happen to be on our side, like a child born to loving parents. Soares disagrees: It’s up to us to make it so. This is the “alignment problem.” Aligning an AI’s behavior with our values and goals is no trivial task. In fact, it may be so challenging, and so consequential, that it defines our time, according to one of Soares’ employees, the decision theorist Eliezer Yudkowksy. Yudkowksy’s Twitter bio reads “Ours is the era of inadequate AI alignment theory. Any other facts about this era are relatively unimportant …” He recently convinced Neil deGrasse Tyson that keeping a super intelligent machine “in a box,” disconnected from the Internet, was a lousy safeguard for a misaligned AI and bemoaned Steven Pinker’s misconceptions about AI safety. Donors find the Institute’s mission worthwhile: In December 2017, 341 of them gifted it $2.5 million.
Over the last few months, I’ve been corresponding with Soares over email about why, among other things, a misaligned AI doesn’t need to be malicious to do us harm.
How could AI harm us?
When laypeople imagine AI going wrong, they often imagine the Terminator movies, but I think the “Sorcerer’s Apprentice” short from the 1940 Disney film Fantasia is a much better fictional illustration. The problem Mickey faces when he enchants a broom to help him fill a cauldron isn’t that the broom rebels or acquires a will of its own, but that it fulfills the task it was given all too well.1 The task is in a complex physical environment that makes it hard to fully specify everything Mickey really cares about. He wants the cauldron full, and overflowing the workshop is a great way to be extra confident that the cauldron is full (and stays full). Mickey successfully “aimed” his AI system but things still went poorly for Mickey.
Could today’s AI systems, which work in narrow domains, endanger us?
Not today, but as AI systems get better at finding clever strategies, and as they work in more complicated situations, it gets harder to find directions we could aim them in such that the results are good. Even if we did know which directions to aim very clever/capable systems such that their objectives align with the outcomes we actually want, there’s the remaining problem that we don’t yet have a good understanding of how to point a highly capable optimization process in a particular direction. This problem is more abstract, but also more important, in my estimation.
There’s no danger of your self-driving car or your Go engine “waking up.”
How would you describe a highly capable optimization process?
We can think of an “effective” or “capable” AI system as one that’s very good at identifying sequences of actions which, when executed, produce a certain outcome. To do this in complicated real-world scenarios (such as when coming up with an idea for a scientific experiment or a blueprint for a futuristic technological device), the AI system needs to be able to build and manipulate accurate models of the world. The world is a complicated place, and the first AI systems capable of modeling and managing that complexity are likely to be complicated systems in and of themselves. If we aren’t careful, we’ll end up in a situation where we have a highly capable system working for reasons no one quite understands.
Why should we worry about not understanding how an AI works?
We wouldn’t be able to direct the system’s efforts by, for example, knowing how those efforts come about and reaching in to ensure that every computation the machine carries out is done in service of a particular objective. We would instead be relegated to using more indirect and surface-level methods for directing problem-solving work in desired ways, where for example we reward the system for behavior that we consider good and punish it for behavior we consider bad. I largely expect surface-level methods to fail.
Why do you expect indirect methods of controlling AI to fail?
Consider the case of natural selection, which aggressively rewards genomes that promote fitness in the organism and penalizes genomes that decrease fitness. This eventually resulted in genomes that coded for a highly capable generally intelligent reasoning system (the human brain), but the result is an enormous and complicated kludge, endowed with hopes and fears and dreams that, in today’s world, often have nothing to do with genetic fitness, or even actively conflict with fitness (in, for example, the case of birth control). Which is to say, it’s quite possible to design a training regime that rewards an AI system for the desired behavior and punishes it for undesirable behavior, only to have it end up pursuing goals only loosely correlated with the desired behavior. Engineers can take these worries into account and deliberately design systems to avert these concerns, but it’s likely to take a fair amount of extra work. If we drop the ball on these problems the likely outcome is disaster, though it’s hard to predict the shape of the disaster without knowing the details of how and when we’ll get to smarter-than-human AI.
Are you worried that narrow-intelligence AI will gradually blur into general-intelligence AI?
I have been impressed by some of DeepMind’s results, including AlphaZero. Which is not to say that AlphaZero has the seeds of general intelligence, necessarily. Though it’s definitely quite a bit more general than DeepBlue was, for what it’s worth. Still I’m not too worried about narrow AI blurring into general AI: I expect the latter to involve architectural challenges the solutions to which I don’t expect narrow systems can stumble into during the course of everyday operation. There’s no danger of your self-driving car or your Go engine “waking up” and being an artificial general intelligence one day. I strongly expect that if one wants DeepMind’s system to be a general reasoner, they have to build it to be a general reasoner from the get-go. That said, predicting what the AI systems of tomorrow will look like is a notoriously difficult task, so I retain a lot of uncertainty in this area.
If we aren’t careful, we’ll end up in a situation where we have a highly capable system working for reasons no one quite understands.
Why do you say you’re not involved in AI ethics?
“AI ethics,” in my mind, covers things like making sure that self-driving cars can solve trolley problems, or coming up with social policies, like basic income, to deal with automation, or thinking about what legal rights you should give to machines if you build conscious machines. Those aren’t the sorts of questions I focus on. My focus is roughly around the question: “If you handed me algorithms for automating scientific research and technological innovation, how could I safely use those algorithms to complete important tasks?” If conventional capabilities research is about developing systems that can solve increasingly hard problems, alignment research is about ensuring that we can reliably aim those capabilities at the problems we want solved—even when our AI algorithms have vastly more scientific and technological capabilities than we do.
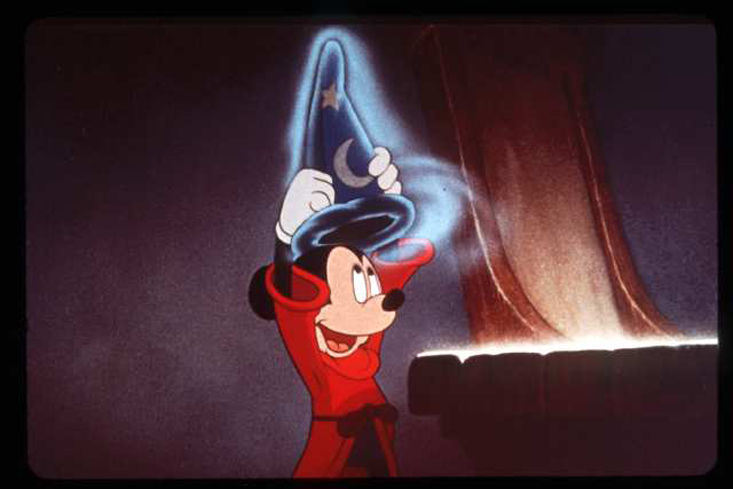
How did your work at Google lead you here today?
Around 2012, while employed at Google, I came across arguments claiming that AI—specifically the kind of AI system that could do novel science and engineering work—could accelerate technological progress enormously and produce an enormous amount of good, if well-aligned. I spent some time examining these arguments, and (to my surprise) found them to be solid. I also found that there weren’t as many researchers working on the alignment parts of the puzzle as I had expected. And, of course, I found the topic fascinating: Intelligence is still a mystery in many respects, and AI is one of those exciting scientific frontiers, and I enjoy the way that studying AI helps me refine my understanding of my own mind. The Machine Intelligence Research Institute was one of very few organizations working AI alignment. I got in touch in mid-2013 and asked MIRI what sort of resources they needed to accelerate their alignment research. Six months later—after some very intensive study into mathematics and AI—they hired me as a researcher. A little over a year after that, I was made MIRI’s executive director.
Was Google not receptive to alignment research?
No. They weren’t particularly unreceptive, either. In 2012, the current wave of hype about general artificial intelligence hadn’t gotten started yet, and there just wasn’t much discussion about AI’s long-term technological trajectory and impact. That’s changed somewhat. For example, these days, high-profile groups such as Google DeepMind, OpenAI, and FAIR (which stands for Facebook AI Research) have explicit goals to develop things like “general AI” or “human-level AI.” But Google didn’t acquire DeepMind until 2014, and back in 2012, the research community tended to focus more on narrower applications. There’s still a surprisingly small amount of work going into alignment, not just at Google but in general. I would say that Google’s work in alignment research properly began in 2016, with a few important milestones: DeepMind launched their alignment research team, and researchers at Google Brain, Open AI, and Stanford released a solid research agenda, “Concrete Problems in AI Safety.”
You’re hiring a Machine Learning Living Librarian. What is that?
We have a bunch of researchers working in the machine-learning space, and it often seems that their work could be sped up if there was an expert who was deeply familiar with a large swath of the field (who can quickly answer questions, point people to the relevant parts of the literature, and so on). I’m a fan of specializing labor, and this seems like an area ripe for gains from specialization. Sometimes we’re looking to hire researchers who have their own ideas about how to pursue AI alignment, and other times we’re looking to hire researchers and engineers who can dramatically speed up our ability to pursue promising areas of research that we’ve already picked out, and I think it’s important to be clear about which positions are which.
Brian Gallagher is the blog editor at Nautilus. Follow him on Twitter @BSGallagher.
Lead image: Screenshot from YouTube / Disney