In 2005 neuroscientist Henry Markram embarked on a mission to create a supercomputer simulation of the human brain, known as the Blue Brain Project. In 2013 that project became the Human Brain Project (HBP), a billion-euro, 10-year initiative supported in part by the European Commission. The HBP polarized the neuroscience community, culminating in an open letter last July signed by nearly 800 neuroscientists, including Nobel Prize–winners, calling the HBP’s science into question. Last month the critics were vindicated, as a mediation committee called for a total overhaul of the HBP’s scientific goals.
“We weren’t generating discontent,” says Zachary Mainen, a neuroscientist at the Champalimaud Centre for the Unknown, who co-authored the open letter with Alexandre Pouget of the University of Geneva. “We tapped into it.”
So, what was wrong with the Human Brain Project? And what are the implications for how we study and understand the brain? The HBP, along with the U.S.’s multibillion-dollar BRAIN Initiative, are often compared to other “big science” endeavors, such as the Human Genome Project, the Large Hadron Collider at CERN, or even NASA’s moon landing. But given how much of the brain’s workings remain mysterious, is big science the right way to unlock its mysteries and cure its diseases?
The HBP’s main approach to brain simulation was “bottom up,” meaning researchers would start with as much detailed data as possible, plug it all into a computer, and then observe what emerges out of the simulation. The idea was that scientists from all over the world would book time on the simulated brain to run virtual experiments. HBP co-director Henry Markram made sweeping claims, saying that scientists would be able to run “computer-based drug trials” to shed light on possible treatments for psychiatric and neurological disease.
But this massive bottom-up approach to brain simulation, many researchers argue, is premature at best, and fundamentally flawed at worst. A bottom-up simulation requires that researchers enter a huge amount of detailed data, so a reasonable question to ask is, where to start? Which level of detail should we use for the simulation?
Surely we don’t need to simulate every elementary and subatomic particle, and probably not every atom. Do we need to simulate each molecule? After all, DNA is a molecule, and the genes it contains profoundly influence brain structure and function. Proteins are molecules, and besides carrying out most cellular functions, they can misfold and cause diseases such as fatal familial insomnia and mad cow disease. And brain cells communicate using molecules called neurotransmitters, and they are affected by molecules such as hormones. So do we need to simulate each molecule of DNA, every protein, every neurotransmitter, and every hormone? Or do we ignore the molecules and just focus on cells? Neurons are often called “the building blocks of the brain,” but the electrical signals that travel along brain cells and allow them to communicate are carried by ions—which are single atoms. So maybe we should simulate atoms after all. In other words, we’re pretty much back where we started with no clue how to get going.
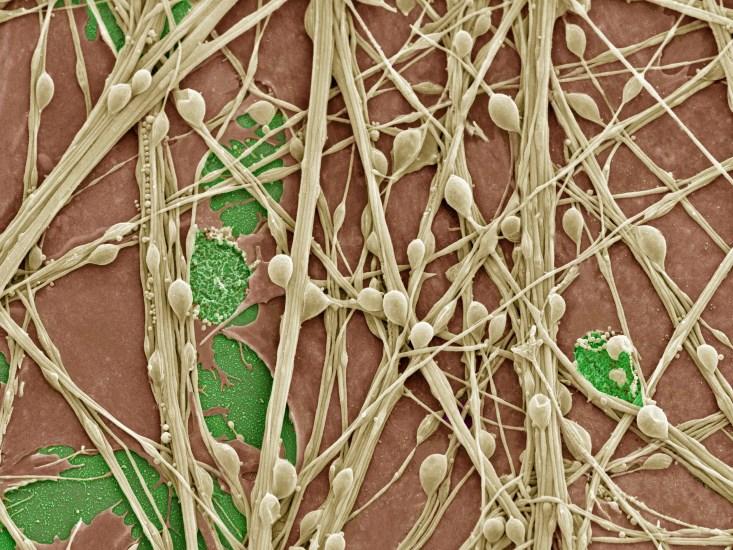
Arguing over the level of detail in the simulation might seem like a detail itself, but it actually reveals something important about the state of neuroscience. The fundamental units in the simulation depend upon the hypothesis being tested. For example, if you want to study a drug that affects neurotransmitters, you might have to simulate at the level of molecules. If you were interested in how the electrical activity of neural networks gives rise to cognition, you would simulate at the cellular level or higher. In either case, to generate a hypothesis, you should first have a well-established theoretical framework that explains how the brain generally works.
Such a framework would help connect the levels of detail by telling you which components actually matter in cognition. When forecasting the weather, for example, meteorologists don’t begin with every atom in the atmosphere—they use theories from fluid mechanics and thermodynamics to model macroscopic properties like pressure and temperature, which arise from the collective activity of molecules. Neuroscience, in contrast, currently lacks anything close to such comprehensive theories, even in the simplest of cases.
As Christof Koch, Chief Scientific Officer at the Allen Institute for Brain Science puts it, “The dirty secret is that we don’t even understand the nematode C. Elegans, which only has 302 neurons [in contrast with the nearly 100 billion in the human brain]. We don’t have a complete model of this tiny organism.”
Edvard Moser, who shared the Nobel Prize last year for his research into brain function and signed the open letter, agrees that starting a huge simulation without clear hypotheses is the project’s major flaw. “As I understand it, tons of data will be put into a supercomputer and this will somehow lead to a global understanding of how the brain works.” But, he went on, “To simulate the brain, or a part of the brain, one has to start with some hypothesis about how it works.” Until we at least have some well-grounded theoretical framework, building a huge simulation is putting the cart before the horse.
The larger point is that neuroscientists are mostly in the dark about how our brains operate. Forget the “hard problem” of consciousness: We don’t even understand the code that neurons use to communicate, except in rare cases. The way that many neurotransmitters affect the activity of neural networks is largely unknown. Whether it matters that we know the exact way that neurons are wired up to each other is up for debate. Many of the specifics of how we form and recall memories are not, for the most part, understood. We don’t really know how people make predictions or imagine the future. Emotions remain mysterious. We don’t even know why we sleep—in other words, how we spend about a third of our lives is an enigma.
Building a huge model of the brain might seem like a way forward. But according to Eve Marder of Brandeis University, a member of the advisory committee to the BRAIN Initiative, such a project is based on a fundamental misunderstanding of the role of models in science. “The purpose of building a model should never be to attempt to replicate the fullness of biological complexity, but to provide a simplified version that reveals general principles,” she says. She adds that even the most ambitious attempt to collect all of the data you’d need to make a bottom-up simulation will necessarily be incomplete, and scientists will be “forced to estimate, or just ‘make up’ many details. In that case, the model is destined to fail to produce new understanding because it will be as complex as the biological system, but wrong.”
This is not to say there is no role for brain simulations in neuroscience. In fact, the growing field of computational neuroscience is increasingly influencing how neuroscientists think about the brain. But as we just saw, bottom-up whole-brain simulation is hugely problematic, and Markram’s computer-based drug trials—presumably run on virtual brains that have virtual diseases—necessitate a level of complexity that would require, for one thing, knowing how to model diseases we barely understand.
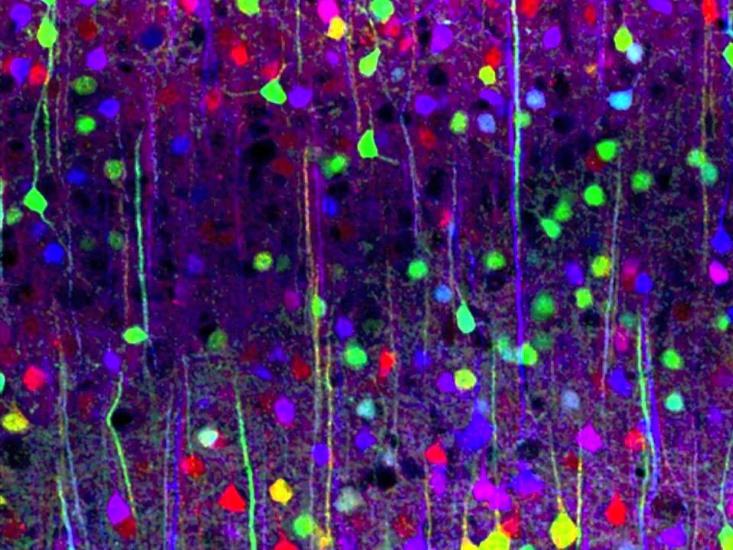
So should the HBP be scrapped? The mediation committee that recently overhauled the project plan doesn’t think so, and not just because it would be politically difficult. What saves the HBP is that it is more than just a brain simulation: It has several sub-projects that may very well lead to good science or useful technologies. There is a neuroinformatics group developing tools for data analysis and storage. There is a high-performance computing group developing cutting-edge computing technologies to support analysis of the large data sets common in neuroscience research. There is even a group trying to develop the overarching theories that neuroscience currently lacks.
Putting all neuroscience data in one place, for example, could accelerate discovery and spark collaborations. “It makes sense to have a database,” says Peter Dayan of University College London, a vocal opponent of the HBP and a member of the mediation committee. “Henry [Markram] makes an excellent point. You go to the Society for Neuroscience meeting and you see 30,000 different posters, and it would be lovely to have a mechanism to find out what’s known.”
In fact, the HBP leadership has backpedaled a bit (pdf), saying the simulation is simply a tool to get the world’s neuroscience data organized, and that the uproar is just a misunderstanding of what was always meant to be a technology project, not just a neuroscience project. But that rhetorical shift is difficult to reconcile with the brain-simulation publicity that sold the idea. And besides, according to the mediation report, the simulation still “represents much of the core of the HBP.” But at this point, it’s pretty much been discredited. Dayan went on: “We all think it’s a scientifically misguided endeavor.”
In contrast, the U.S.’s BRAIN Initiative is a collection of many different projects that share a very different goal: to develop better tools to measure activity in the brain. And while the HBP was run almost autocratically, the BRAIN Initiative was conceived by a diverse group of top scientists. As co-director Cori Bargmann says, “A lot of people had a chance to weigh in and contribute ideas about priorities and plans.”
Creating new tools, while it may not sound quite as sexy as simulating the human brain, is hugely important, because current technologies—especially those we use in humans—have major limitations. For example, we have no way to track the activity of the brain’s billions of neurons at the millisecond-by-millisecond speed of thinking. The better we can observe the brain, the better we can understand it.
But here may be the real conclusion from looking at the HBP and the BRAIN Initiative: Neither should be called “big science.” That’s just rhetoric. If you think about it, building a particle accelerator, sequencing the human genome, or putting a man on the moon are actually not science projects: They are engineering projects. Building a particle accelerator, for example, involves a set of engineering issues—a huge series of very complicated engineering issues. But while the accelerator may help discover lots of new particle physics, there is no new physics needed to build it. Likewise with the Human Genome Project: Determine the sequence of about a three billion base pairs in DNA and you’re done. This requires a lot of clever approaches for reading gene sequences and time-intensive bench work but no new molecular biology. And putting a man on the moon, likewise, involved new rockets, navigation, and life-support systems but no new physics.
In fact, there’s actually no such thing as big science; we should really be calling it big engineering. Scientific disciplines are ready for big engineering once they have a solid, well-established theoretical structure. At the time of the Human Genome Project, scientists agreed about the theory, the “central dogma,” of how genetic information is contained in the sequence of letters in our DNA and how that information is turned into action. Then they joined in a massive engineering effort to unravel the specific sequence of letters inside human cells.
But as of now, “the brain is too complex, and neuroscience is too young, for all funding to be put into a single-aimed project,” according to Moser.
So until we have some solid ideas about how the nearly 100 billion cells function together as a human brain, it’s probably best to concentrate research efforts on relatively small grants to diverse laboratories around the world1. Despite the big-science rhetoric surrounding the BRAIN initiative, that’s essentially the direction the project—and maybe the HBP—is going. And that’s a good thing. Fundamental discovery is fueled by letting researchers follow their noses to what’s important. The structure of DNA wasn’t discovered by a huge, expensive project; it was discovered by a few young researchers obsessed with molecules.
Footnote:
1. To be fair, it’s not a total dichotomy: Big and small approaches can be complementary, and in fact there are a few medium-scale engineering projects in neuroscience. The Allen Institute for Brain Science, a nonprofit organization launched by Microsoft founder Paul Allen, created a widely used “atlas” of gene expression patterns in the mouse brain. Again, no new science was needed, just a large effort by a small army of scientists and technicians. And at the end of this March, the Allen Institute launched a new initiative, called BigNeuron, which aims to classify the types of neurons in the brain (interestingly, this project will receive funding from the HBP).
In addition, there are a few connectome projects underway that seek to map all the connections among neurons, or at least brain areas, in animal and human brains. Whether connectomes can live up to the hype is unclear, but working out a connectome is an engineering project, and in fact was already completed for one animal, the nematode C. Elegans, in 1986. Like the Human Genome Project and the gene-expression atlas, this is a matter of exhaustively mapping a reasonably well-understood system, rather than finding the core principles of how that system works.
None of these mid-size projects have put forth the breathless comparisons to moon landings, etc., and none of them have price tags in the billions, as the HBP or BRAIN Initiative have. There’s a real concern of generating public backlash as these mega-projects hint at cures for, say, Alzheimer’s, when the chances of that happening any time soon, regardless of how we spend money, are, sadly, exceedingly slim.
Tim Requarth is a freelance journalist in New York City and director of Neuwrite. He tweets at @timrequarth.