Memory doesn’t represent a single scientific mystery; it’s many of them. Neuroscientists and psychologists have come to recognize varied types of memory that coexist in our brain: episodic memories of past experiences, semantic memories of facts, short- and long-term memories, and more. These often have different characteristics and even seem to be located in different parts of the brain. But it’s never been clear what feature of a memory determines how or why it should be sorted in this way.
Now, a new theory backed by experiments using artificial neural networks proposes that the brain may be sorting memories by evaluating how likely they are to be useful as guides in the future. In particular, it suggests that many memories of predictable things, ranging from facts to useful recurring experiences—like what you regularly eat for breakfast or your walk to work—are saved in the brain’s neocortex, where they can contribute to generalizations about the world. Memories less likely to be useful—like the taste of that unique drink you had at that one party—are kept in the seahorse-shaped memory bank called the hippocampus. Actively segregating memories this way on the basis of their usefulness and generalizability may optimize the reliability of memories for helping us navigate novel situations.
If the package contains a Spider-Man costume, there is no need to clutter the house with it.
The authors of the new theory—the neuroscientists Weinan Sun and James Fitzgerald of the Janelia Research Campus of the Howard Hughes Medical Institute, Andrew Saxe of University College London, and their colleagues—described it in a recent paper in Nature Neuroscience. It updates and expands on the well-established idea that the brain has two linked, complementary learning systems: the hippocampus, which rapidly encodes new information, and the neocortex, which gradually integrates it for long-term storage.
James McClelland, a cognitive neuroscientist at Stanford University who pioneered the idea of complementary learning systems in memory but was not part of the new study, remarked that it “addresses aspects of generalization” that his own group had not thought about when they proposed the theory in the mid 1990s.
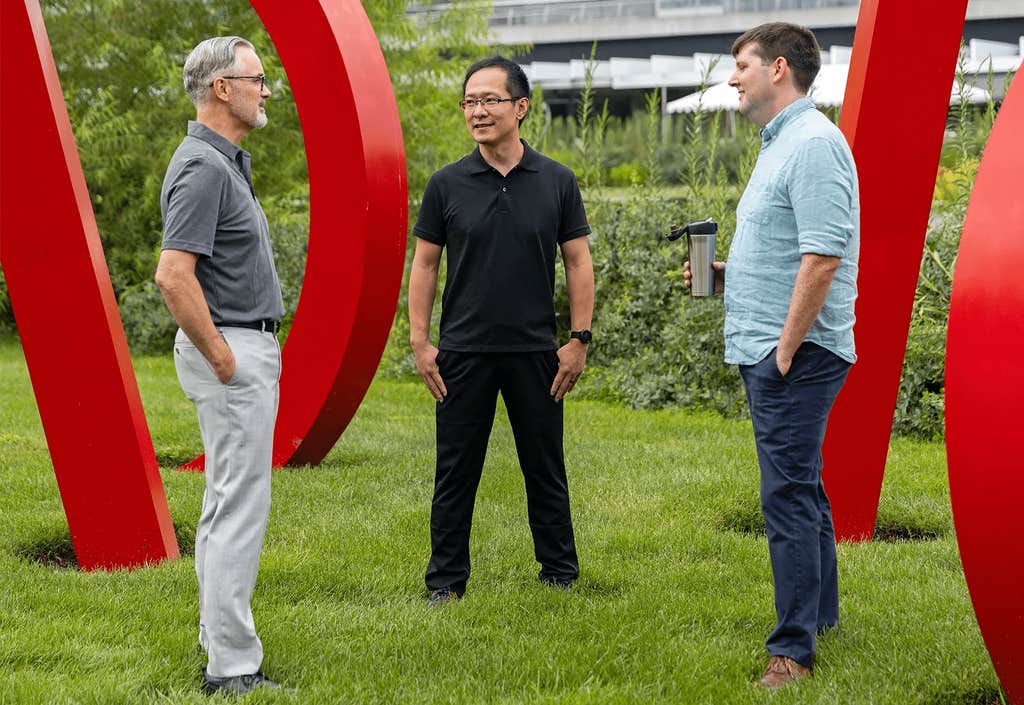
Scientists have recognized that memory formation is a multistage process since at least the early 1950s, in part from their studies of a patient named Henry Molaison—known for decades in the scientific literature only as H.M. Because he suffered from uncontrollable seizures that originated in his hippocampus, surgeons treated him by removing most of that brain structure. Afterward, the patient appeared quite normal in most respects: His vocabulary was intact; he retained his childhood memories and he remembered other details of his life from before the surgery. However, he always forgot the nurse taking care of him. During the decade that she cared for him, she had to introduce herself anew every morning. He had completely lost the ability to create new long-term memories.
Molaison’s symptoms helped scientists discover that new memories first formed in the hippocampus and then were gradually transferred to the neocortex. For a while, it was widely assumed that this happened for all persistent memories. However, once researchers started seeing a growing number of examples of memories that remained dependent on the hippocampus in the long term, it became clear that something else was going on.
To understand the reason behind this anomaly, the authors of the new paper turned to artificial neural networks, since the function of millions of intertwined neurons in the brain is unfathomably complicated. These networks are “an approximate idealization of biological neurons” but are far simpler than the real thing, Saxe said. Like living neurons, they have layers of nodes that receive data, process it, and then provide weighted outputs to other layers of the network. Just as neurons influence each other through their synapses, the nodes in artificial neural networks adjust their activity levels based on inputs from other nodes.
It can be vitally important to remember exceptional experiences.
The team linked three neural networks with different functions to develop a computational framework they called the teacher-notebook-student model. The teacher network represented the environment that an organism might find itself in; it provided inputs of experience. The notebook network represented the hippocampus, rapidly encoding all the details of every experience the teacher provided. The student network trained on the patterns from the teacher by consulting what was recorded in the notebook. “The goal of the student model is to find neurons—nodes—and learn connections [describing] how they could regenerate their pattern of activity,” Fitzgerald said.
The repeated replays of memories from the notebook network entrained the student network to a general pattern through error correction. But the researchers also noticed an exception to the rule: If the student was trained on too many unpredictable memories—noisy signals that deviated too much from the rest—it degraded the student’s ability to learn the generalized pattern.
From a logical standpoint, “this makes a lot of sense,” Sun said. Imagine receiving packages at your house, he explained: If the package contains something useful for the future, “such as coffee mugs and dishes,” it sounds reasonable to bring it into your home and keep it there permanently. But if the package contains a Spider-Man costume for a Halloween party or a brochure for a sale, there is no need to clutter the house with it. Those items can be stored separately or thrown away.
The study provides an interesting convergence between the systems used in artificial intelligence and those employed in modeling the brain. This is an instance where “the theory of those artificial systems gave some new conceptual ideas to think about memories in the brain,” Saxe said.
There are parallels, for example, to how computerized facial recognition systems work. They may begin by prompting users to upload high-definition images of themselves from different angles. Connections within the neural network can piece together a general conception of what the face looks like from different angles and with different expressions. But if you happen to upload a photo “containing the face of your friend in it, then the system is unable to identify a predictable facial mapping between the two,” Fitzgerald said. It damages generalization and makes the system less accurate at recognizing the normal face.
These images activate specific input neurons, and activity then flows through the network, adjusting connection weights. With more images, the model further adjusts the connection weights between nodes to minimize output errors.
But just because an experience is unusual and doesn’t fit into a generalization, that doesn’t mean that it should be discarded and forgotten. To the contrary, it can be vitally important to remember exceptional experiences. That seems to be why the brain sorts its memories into different categories that are stored separately, with the neocortex used for reliable generalizations and the hippocampus for exceptions.
This kind of research raises awareness about the “fallibility of human memory,” McClelland said. Memory is a finite resource, and biology has had to compromise to make the best use of the limited resources. Even the hippocampus does not contain a perfect record of experiences. Each time an experience is recalled, there are changes in the connection weights of the network, causing memory elements to get more averaged out. It raises questions about the circumstances under which “eyewitness testimony [could] be protected from bias and influence from repeated onslaughts of queries,” he said.
The model may also offer insights into more fundamental questions. “How do we build up reliable knowledge and make informed decisions?” said James Antony, a neuroscientist at California Polytechnic State University who was not involved in the study. It shows the importance of evaluating memories to make reliable predictions—a lot of noisy data or unreliable information may be as unsuitable for training humans as it is for training AI models.
This article was originally published on the Quanta Abstractions blog.
Lead illustration: Kristina Armitage/Quanta Magazine