After the fall of the Berlin Wall, East German citizens were offered the chance to read the files kept on them by the Stasi, the much-feared Communist-era secret police service. To date, it is estimated that only 10 percent have taken the opportunity.
In 2007, James Watson, the co-discoverer of the structure of DNA, asked that he not be given any information about his APOE gene, one allele of which is a known risk factor for Alzheimer’s disease.
Most people tell pollsters that, given the choice, they would prefer not to know the date of their own death—or even the future dates of happy events.
Each of these is an example of willful ignorance. Socrates may have made the case that the unexamined life is not worth living, and Hobbes may have argued that curiosity is mankind’s primary passion, but many of our oldest stories actually describe the dangers of knowing too much. From Adam and Eve and the tree of knowledge to Prometheus stealing the secret of fire, they teach us that real-life decisions need to strike a delicate balance between choosing to know, and choosing not to.
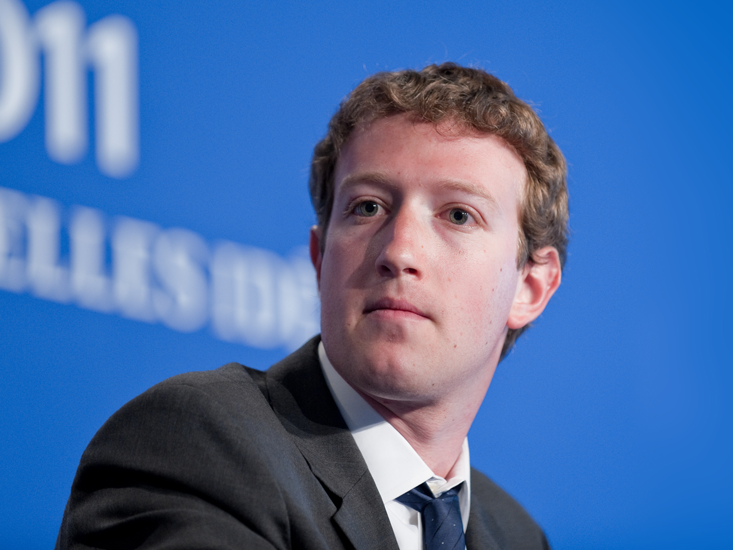
But what if a technology came along that shifted this balance unpredictably, complicating how we make decisions about when to remain ignorant? That technology is here: It’s called artificial intelligence.
AI can find patterns and make inferences using relatively little data. Only a handful of Facebook likes are necessary to predict your personality, race, and gender, for example. Another computer algorithm claims it can distinguish between homosexual and heterosexual men with 81 percent accuracy, and homosexual and heterosexual women with 71 percent accuracy, based on their picture alone.1 An algorithm named COMPAS (Correctional Offender Management Profiling for Alternative Sanctions) can predict criminal recidivism from data like juvenile arrests, criminal records in the family, education, social isolation, and leisure activities with 65 percent accuracy.2
Knowledge can sometimes corrupt judgment, and we often choose to remain deliberately ignorant in response.
In each of these cases, the nature of the conclusion can represent a surprising departure from the nature of the data used (even if the validity of some of the results continues to be debated). That makes it hard to control what we know. There is also little to no regulation in place to help us remain ignorant: There is no protected “right not to know.”
This creates an atmosphere where, in the words of Facebook’s old motto, we are prone to “move fast and break things.” But when it comes to details about our private lives, is breaking things really what we want to be doing?
Governments and lawmakers have known for decades that Pandora’s box is sometimes best left closed. There have been laws on the books protecting the individual’s right to ignorance stretching back to at least the 1990s. The 1997 European Convention on Human Rights and Biomedicine, for example, states that “Everyone is entitled to know any information collected about his or her health. However, the wishes of individuals not to be so informed shall be observed.” Similarly, the 1995 World Medical Association’s Declaration on the Rights of the Patient states that “the patient has the right not to be informed [of medical data] on his/her explicit request, unless required for the protection of another person’s life.”
Writing right-to-ignorance laws for AI, though, is a very different matter. While medical data is strongly regulated, data used by AI is often in the hands of the notoriously unregulated for-profit tech sector. The types of data that AI deals with are also much broader, so that any corresponding laws require a broader scope of understanding of what a right to ignorance means. Research into the psychology of deliberate ignorance would help with designing right-to-ignorance laws for AI. But, surprisingly, the topic has long been ignored as a topic of rigorous scientific inquiry, perhaps because of the implicit assumption that deliberately avoiding information is irrational.
Recently, though, the psychologist Ralph Hertwig and legal scholar Christoph Engel have published an extensive taxonomy of motives for deliberate ignorance. They identified two sets of motives, in particular, that have a particular relevance to the need for ignorance in the face of AI.
The first set of motives revolves around impartiality and fairness. Simply put, knowledge can sometimes corrupt judgment, and we often choose to remain deliberately ignorant in response. For example, peer reviews of academic papers are usually anonymous. Insurance companies in most countries are not permitted to know all the details of their client’s health before they enroll; they only know general risk factors. This type of consideration is particularly relevant to AI, because AI can produce highly prejudicial information.
The second relevant motives are emotional regulation and regret avoidance. Deliberate ignorance, Hertwig and Engel write, can help people to maintain “cherished beliefs,” and avoid “mental discomfort, fear, and cognitive dissonance.”3 The prevalence of deliberate ignorance is high. About 90 percent of surveyed Germans want to avoid negative feelings that may arise from “foreknowledge of negative events, such as death and divorce,” and 40 to 70 percent also do not want to know about positive events, to help maintain “positive feelings of surprise and suspense” that come from, for example, not knowing the sex of an unborn child.4
We’ve been giving our data away for so long that we’ve forgotten it’s ours in the first place.
These sets of motives can help us understand the need to protect ignorance in the face of AI. The AI “gaydar” algorithm, for example, appears to have close to zero potential benefits, but great potential costs when it comes to impartiality and fairness. As The Economist put it, “in parts of the world where being gay is socially unacceptable, or illegal, such an algorithm could pose a serious threat to safety.” Similarly, the proposed benefits of an ethnicity detector currently under development at NtechLab seem to pale in comparison to the negative impact on impartiality and fairness. The use of the COMPAS recidivism prediction software has a higher accuracy than a human but, as Dressel and Farid write, is “not as accurate as we might want, particularly from the point of view of a defendant whose future lies in the balance.”2 Algorithms that predict individual life expectancy, like those being developed by Aspire Health, are not necessarily making emotional regulation any easier.
These examples illustrate the utility of identifying individual motives for ignorance, and show how complex questions of knowledge and ignorance can be, especially when AI is involved. There is no ready-made answer to the question of when collective ignorance is beneficial or ethically appropriate. The ideal approach would be to consider each case individually, performing a risk-benefit analysis. Ideally, given the complexity of the debate and the weight of its consequences, this analysis would be public, include diverse stakeholder and expert opinions, and consider all possible future outcomes, including worst-case scenarios.
That’s a lot to ask—in fact, it is probably infeasible in most cases. So how do we handle in broad strokes something that calls for fine shading?
One approach is to control and restrict the kinds of inferences we allow machines to make from data that they have already collected. We could “forbid” judicial algorithms from using race as a predictor variable, for example, or exclude gender from the predictive analyses of potential job candidates. But there are problems with this approach.
First of all, restricting the information used by big companies is costly and technically difficult. It would require those companies to open-source their algorithms, and large governmental agencies to constantly audit them. Plus once big data sets have been collected, there are many ways to infer “forbidden knowledge” in circuitous ways. Suppose that using gender information to predict academic success was declared illegal. It would be straightforward to use the variables “type of car owned” and “favorite music genre” as a proxy for gender, performing a second-order inference and resting the prediction on proxies of gender after all. Inferences about gender may even be accidentally built into an algorithm despite a company’s best intentions. These second-order inferences make the auditing of algorithms even more daunting. The more variables that are included in an analysis, the higher the chances that second-order inferences will occur.
The more radical—and potentially more effective—approach to protecting the right to ignorance is to prevent data from being gathered in the first place. In a pioneering move in 2017, for example, Germany passed legislation that prohibits self-driving cars from identifying people on the street by their race, age, and gender. This means that the car will never be able to inform its driving decisions—and especially the decisions it needs to take when an accident is unavoidable—with data from these categories.
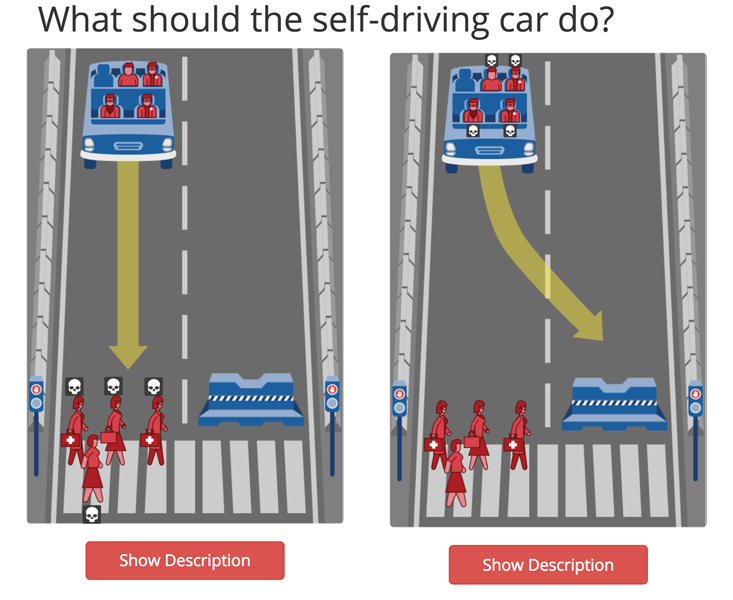
In line with this way of thinking, the European Union’s new General Data Protection Regulation (GDPR), which became effective in May 2018, states that companies are permitted to collect and store only the minimum amount of user data needed to provide a specific, stated service, and to get customers’ consent for how their data will be used. Such a restriction on data capture may also prevent second-order inferences. One important limitation of the GDPR approach is that companies can give themselves very broad objectives. The now-shut Cambridge Analytica’s explicit objective, for example, was to assess your personality, so technically its controversial collection of Facebook data satisfied GPDR’s guidelines. Similarly, GPDR’s focus on the alignment between data and a given service does not exclude categories of data we find morally questionable, nor completely stop companies from buying excluded data from a data broker as long as the user has consented—and many people consent to sharing their data even with relatively meager incentives. Researchers found that some MIT students would share their friends’ contact data for a slice of pizza.5 Clearly, further restrictions are needed. But how many?
The American activist and programmer Richard Stallman gave this answer: “There are so many ways to use data to hurt people that the only safe database is the one that was never collected.” But restricting data collection too severely may impede progress and undermine the benefits we stand to gain from AI.
Who should decide on these tradeoffs? We should all do it ourselves.
In most cases we are actually talking about data that is owned by you and me. We have been careless in giving it away for shiny apps without considering the consequences. In fact, we’ve been giving our data away for so long that we’ve forgotten it’s ours in the first place. Taking it back allows us to individually decide whether there is something we want or don’t want to know. Restoring data to its rightful owners—us—neatly solves many of the hard challenges we’ve discussed. It avoids the need to develop universal, prescient guidelines about data. Instead, millions of individuals will guide their own data usage according to their sense of what is right and wrong. We can all react in real time to evolving uses of data by companies, punishing or rewarding companies according to how their data is treated.
The computer science philosopher Jaron Lanier has suggested an additional, economic argument for placing data back into the hands of people. We should all be able to profit from our private data, he reasons, by selling it to big companies. The problem with this approach is twofold. First, it muddles the ethics of data use and ownership. The willingness to give data away for free is a good litmus test for the ethical integrity of the questions that data will be used to answer. How many individuals from a minority group would freely give away their data in order to create a facial recognition app like the gaydar? And how many would agree to be paid to do so? On the other hand, a majority of the population would gladly contribute their data to finding a cure for cancer. Second, putting (high) economic value on personal data may coerce people to share their data and make data privacy a privilege of the rich.
This isn’t to say that individual action alone will be sufficient. Collective action by society’s institutions will also be required. Even if only a small portion of the population shares their sensitive data, the result may be a high predictive accuracy opposed by the majority. Not all of us are aware of this. To prevent unwanted consequences we would need additional laws and public debates.
The Economist has written that the world’s most valuable resource is no longer oil—it’s data. But data is very different from oil. Data is an unlimited resource, it’s owned by individuals, and it’s best exchanged without any transactional economic value. Taking the profit out of oil kills the oil market. As a first step, taking profit out of data provides the space we need to create and maintain ethical standards that can survive the coming of AI, and pave the way for managing collective ignorance. In other words, as data becomes one of the most useful commodities of the modern world, it also needs to become one of the cheapest.
Christina Leuker is a pre-doctoral fellow at the Max Planck Institute for Human Development.
Wouter van den Bos is a research scientist at the Max Planck Institute for Human Development.
References
1. Wang, Y. & Kosinski, M. Deep neural networks are more accurate than humans at detecting sexual orientation from facial images. Journal of Personality and Social Psychology 114, 246-257 (2018).
2. Dressel, J. & Farid, H. The accuracy, fairness, and limits of predicting recidivism. Science Advances 4, eaao5580 (2018).
3. Hertwig, R. & Engel, C. Homo ignorans: Deliberately choosing not to know. Perspectives on Psychological Science 11, 359-372 (2016).
4. Gigerenzer, G. & Garcia-Retamero, R. Cassandra’s regret: The psychology of not wanting to know. Psychological Review 124, 179-196 (2017).
5. Athey, S. Catalini, C., & Tucker, C.E. The digital privacy paradox: Small money, small costs, small talk. Stanford University Graduate School of Business Research Paper No. 17-14 (2018).
Additional Reading
Stallman, R. A radical proposal to keep your personal data safe. The Guardian (2018).
Staff writers. The world’s most valuable resource is no longer oil, but data. The Economist (2017).
Lead photo collage credit: Oliver Burston / Getty Images; Pixabay