It’s Monday morning of some week in 2050 and you’re shuffling into your kitchen, drawn by the smell of fresh coffee C-3PO has brewed while he unloaded the dishwasher. “Here you go, Han Solo, I used the new flavor you bought yesterday,” C-3PO tells you as he hands you the cup. You chuckle. C-3PO arrived barely a month ago and already has developed a wonderful sense of humor and even some snark.
He isn’t the real C-3PO, of course—you just named him that because you are a vintage movie buff—but he’s the latest NeuroCyber model that comes closest to how people think, talk, and acquire knowledge. He’s no match to the original C-3PO’s fluency in 6 million forms of communication, but he’s got full linguistic mastery and can learn from humans like humans do—from observation and imitation, whether it’s using sarcasm or sticking dishes into slots. Unlike the early models of such assistants like Siri or Alexa who could recognize commands and act upon them, NeuroCybers can evolve into intuitive assistants and companions. You make a mental note to get one for Grandma for the upcoming holiday season. She’s been feeling lonely so she could use company.
Let’s make it clear—you’re not getting this NeuroCyber C-3PO for Grandma this holiday season. Or any holiday season soon. But building such intelligent helpers and buddies may be possible if we engineer their learning abilities to be similar to those of humans or other animals, argues Anthony Zador, a professor of neurosciences at Cold Spring Harbor Laboratory who studies how brain circuitry gives rise to complex behaviors.
Humans come with a lot of scripted behavioral wisdom. AI comes with none.
Currently, some AI models are making strides toward natural language processing using the so-called “deep learning approach,” which tries to mimic how humans acquire knowledge. One such language learning model, GPT-3 by San Francisco-based company OpenAI, “is like a supercharged version of the autocomplete version on your phone,” Raphael Milliere reported in Nautilus. “Given a definition, it can use a made-up word in a sentence. It can rewrite a paragraph in the style of a famous author. It can write creative fiction. Or generate code for a program based on a description of its function. It can even answer queries about general knowledge.”
But to say that GPT-3 thinks like humans is an overstatement because it still makes funny mistakes no human would. In one of its articles, GPT-3 wrote that if one drinks a glass of cranberry juice with a spoonful of grape juice, one will die. “I’m very impressed by what GPT-3 can do,” Zador says, but it still needs to be taught some rudimentary facts of life. “So it’s important to focus on what it’s still missing.” And what GPT-3 and other AI programs are missing is something important: a genome. And the millions of years of evolution it took for this genome to form.
Humans and all other living beings come with a set of pre-wired behaviors written into their genes, accumulated along the long and circuitous evolutionary journey. Animals have been around for about 100 million years and humans for about 100,000 years. That’s a lot of behavioral wisdom scripted in the genetic language of amino acids and nucleotides. On the contrary, AI comes with none. It has no genes to inherit and no genes to pass on. Humans and animals are products of both nature and nurture, but AI has only the former. Without the latter, it will never think like us.
That’s why AI and robots are superior to humans only in specific things, while failing at others. They beat world class players in chess and Go. They outperform us in math and everything else that relies on rules or calculations. They are indispensable when things are dangerous, dull, too heavy, or too repetitive. But they aren’t good at thinking on their feet, improvising, and interacting with the real world, especially when challenged by novel situations they weren’t trained to deal with.
“I would love to have a robot load up dishes into my dishwasher, and I’d love to have a robot clean my house,” says Zador, but we are far from making such helpful assistants. When it comes to household chores, we’re at the level of Roomba. “You’d think stocking up dishes and tidying up a room is an easier problem than playing Go. But not for robots, not for AI.” A child can learn to load up the dishwasher by watching their parent, but no Roomba can master the feat. In the famous match between 18-time world Go champion Lee Sedol and his AI opponent AlphaGo, the latter won by calculations, but couldn’t physically place the pieces for its moves. It had to rely on its human assistant to move the stones.
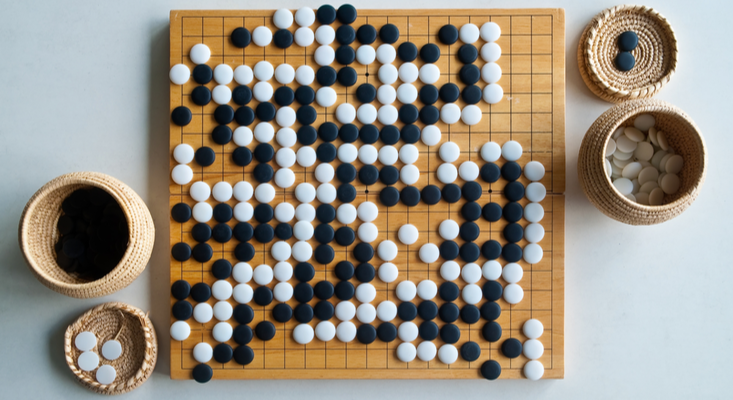
Even if you train a robot to load the dishwasher with a set of dishes and cups, but then add pots and pans into the mix, you’d confuse your cyborg helper, while a child would likely figure out how to re-arrange the objects. That limitation is true across the AI world. “Currently, AIs are very narrow,” says Blake Richards, an assistant professor at the School of Computer Science and the Montreal Neurological Institute at McGill University. Even the smart algorithms are limited in their tasks. “For example, a Facebook algorithm checks every image to make sure it’s not pornographic or violent,” says Richards—which is an impressive feat, but it can’t do much else. “AIs are optimized for one specific task, but if you give them a different task, they are not good.” They just don’t learn like living things do.
In neuroscience, the term “learning” refers to a long-lasting change in behavior that is the result of experience. However, most animals spend a small amount of time learning, yet they do well. “Most fish don’t spend a lot of time learning from their parents and neither do insects, who are phenomenally successful in their behaviors,” Zador points out. Bees are born knowing how to pollinate, flies expertly escape your swatter and roaches scutter away at the sound of your walk. “But the vast majority of these behaviors are pre-programmed into a bee, a fly, and a roach,” Zador says. “They don’t learn these behaviors, they come out of the box—or out of the egg—with the ability to do whatever they are supposed to do.” Where’s the instruction set that enables them to do that? In their genome, of course. “Animals are enabled by the innate structures they were born with—their DNA encodes the instructions needed for them to execute these behaviors,” Zador says.
It took evolution 3.2 billion years to create Einstein. How long would it take AI?
Mammals spend more time learning than insects, and humans devote a considerable span of years to acquiring knowledge and practicing their skills. “The amount of time we spend learning is at least an order of magnitude greater than other animals,” Zador says. But we also come with a lot of “preprogrammed” wisdom. “Our ability to learn language is greatly facilitated by the neural circuits that are primed and ready to learn language.” AI, on the contrary, isn’t primed for anything, so it must learn everything from scratch.
Consequently, AI creators always had to do a lot of schooling. Up until the late 1990s, AI developers tried to give AI a set of rules to follow, says Richards. For example, when teaching computers to see, they would program them to recognize certain shapes and features. “Here are the shapes of eyes or noses, and if you see two eyes and a nose in between, that’s likely a face,” Richards explains. That unfortunately didn’t work very well, because the world is simply too complex to fit into such rules. “We were not smart enough to hand-design these things for the messiness of the real world.”
Today AI developers rely on three different types of learning. One is called supervised learning, in which an AI scans hundreds of thousands of pictures of, let say, puppies or elephants that are labeled as such—and learns how puppies and elephants look. But because humans don’t stare at a stack of dog pictures to memorize what a dog looks like, it’s not an ideal way to teach AI to think like humans, Zador notes. He cites one curious example. An AI system was trained on 10 million labeled images, which is how many seconds are in a year. Human children would have to ask a question every second of their life to process a comparable volume of labeled data; plus, most images children encounter aren’t labeled. But children learn to recognize images just fine.
Another approach is unsupervised learning, in which AI trainers keep categories of elephants, puppies, cars, trees, and so on; the AI maps an image to a category and knows what it sees. That is more similar to how we do it—a human child playing with a little plastic toy dog and a big puffy stuffed one, will likely figure out they are the same animal.
And finally, the third way is reinforcement learning: The AI builders give it a goal—for example, find an object in a maze—and let it figure out how to do it. Rats are pretty good at finding cheeses in mazes. AIs still have a ways to go. That’s because finding food is wired into the rats’ genome, but AI does not have one. We are back to square one. To develop human-like intelligence—or rat-like intelligence to begin with—the AI needs a genome. But how do you give a set of genes to an algorithm?
Zador has an idea for that. Genomes encode blueprints for wiring up our nervous system. From that blueprint, arises a human brain of about 100 billion neurons, each of which talks to about a thousand of its neighbors. “A genome is a very compact, condensed, and compressed form of information,” Zador says. He likens a genome to CliffNotes—study guides that condense literary works into key plotlines and themes. “The genome has to compress all the key stuff into a form of biological CliffNotes,” Zador says—and that’s what we should try doing with the AI, too. We should give AI a bunch of behavioral CliffNotes, which it may then unfurl into a human-brain-like repository.
Despite their biological complexity, genomes contain simplified sets of rules on how to wire the brains, Zador says. Living beings retain only the most important features for the most useful behaviors. Bees don’t sing and flies don’t dance because they don’t need to. Humans can do both, but not fly. Zador is developing algorithms that function like a simple rule to generate behavior. “My algorithms would write these CliffNotes on how to solve a particular problem,” he explains. “And then, the neural networks will use them to figure out which ones are useful—and incorporate those into its behavior.” Later, more complex behaviors can be added—presumably all the way to the intelligent assistants who load dishwashers, pay bills, and converse with Grandma.
Another way to emulate learning, at least for simple organisms, is to equip an AI with their neuronal structure and let it advance by way of reinforcement learning. In one such effort, Nikhil Bhattasali, a student at Zador’s lab, outfitted an AI with a subset of a digital mimic of a neuronal structure from a simple worm called C. elegans and let it learn how to move faster. “We took the wiring diagrams from C. elegans and essentially taught it to swim,” Zador says. The worms perfected their squirming motions through millions of years of evolution. When equipped with only about two dozen neurons, the AI caught up with the swimming motions quickly. “With this built-in diagram, it learned to swim much faster than without.”
Richards adds that the best way to let this AI develop would be to essentially mimic evolution. “Evolution endows us with innate capabilities, but evolution itself is not an intelligent designer,” he notes. “Instead, evolution is a brute-force optimization algorithm. So rather than hardwiring any specific behavior into AI, we should optimize a system and then use that point of optimization for the next generation of AI—much as you do with evolution.”
If these ideas work, will that combination of human intelligence and computer speed instantly propel the cyborg to the singularity, intelligence that surpasses our own? If it took 3.2 billion years to create a human Albert Einstein, how long would it take to create an AI equivalent of him?
Richards doesn’t think AI will get to the Albert Einstein level, and here’s why: It will likely hit the energy bottleneck. Making an AI amass as much knowledge as Albert Einstein, or even an average human, might require such an enormous amount of electrical power that it would be too polluting to sustain. The GPT-3 produced the equivalent of 552 metric tons of carbon dioxide during its training—the equivalent of 120 cars in a year—only to think that grape juice is poison.
“I think we’re pretty far from the singularity,” Zador chuckles. He cites a statistical reason why it’s not worth worrying about. Suppose that machines do replace us as the next Einsteins,” he theorizes. “By definition, very, very few of us make outlier genius contributions like Einstein. So does it matter if the probability of making such a contribution drops from 1 in 100 million per generation to basically zero?” Not really.
So building an AI equivalent to Albert Einstein may be neither worth the energy nor statistically important. Besides, the genius theoretician was known to be messy, spacey, and forgetful. With an AI like that, you’d be cleaning up after your assistant.
Lina Zeldovich grew up in a family of Russian scientists, listening to bedtime stories about volcanoes, black holes, and intrepid explorers. She has written for The New York Times, Scientific American, Reader’s Digest, and Audubon Magazine, among other publications, and won four awards for covering the science of poop. Her book, The Other Dark Matter: The Science and Business of Turning Waste into Wealth, was just released by Chicago University Press. You can find her at LinaZeldovich.com and @LinaZeldovich.
Lead image: Production Perig / Shutterstock
Support for this article was provided by Cold Spring Harbor Laboratory. Read more on the Nautilus channel, Biology + Beyond.