Genetic material is the smoking gun of the modern crime scene. Juries in criminal trials are often encouraged to think of DNA profiling as an exact science, in which telltale traces of skin, hair, and blood identify perpetrators with pinpoint accuracy and rule out any likelihood of mistaken identity. The statistics, however, tell a different story.
From the tens of thousands of genes that make up the human genome, scientists have whittled down the list to about 13 pairs that vary most widely among different people. They use these pairs to make graphs called “electropherograms,” in which each set of genes produces a peak of a certain height. The chance that two individuals could have all 13 peak pairs in common has been estimated to be about 1 in 400 trillion, many times the number of people on earth. So if two profiles are found to match perfectly at every peak, it is extremely unlikely that they don’t come from the same person, or from an identical twin.
It can happen, however, that the profile is not completely clear—for example, the sample might contain a mixture of several people’s DNA, it could be degraded, or it might be very small. For this reason, a DNA match is always presented to the court accompanied by a probability figure called the “random match probability” (RMP). This represents the chance that a person picked off the street at random would match the DNA sample in question. The less clear the sample, the higher the RMP.
Bringing probability into the courtroom can turn the apparent obviousness of guilt into the possibility of innocence. Mathematics can shift the balance between an event that seems virtually impossible and one that is merely improbable, introducing an element of uncertainty that might avoid a conviction “beyond a reasonable doubt,” as the criminal standard demands. It can also correct the false friend of common sense. In the Amanda Knox case, in which a young Seattle woman defended herself against charges of murder concerning the death of her British housemate in the Italian town of Perugia in 2007, an appeal judge’s refusal to retest some of the victim’s disputed DNA that was found on a kitchen knife arguably rested on a failure to grasp the fact that multiple uncertain tests can yield a more definitive result.
Such mistakes are all too common, made worse by the fact that the language of statistics is everywhere, encouraging people to think that they understand it well—that they know what percentages mean, that they understand how probabilities work. But managing unlikelihood is, in fact, incredibly tricky, and there are many common and widespread misconceptions that could easily be put right with a bit of mathematical knowledge.
The language of statistics is everywhere, encouraging people to think that they understand it well.
One important tool for interpreting DNA profiles is known as Bayes’ theorem. It is an equation that permits conditional probabilistic reasoning, comparing the likelihood of an outcome given the existence of a state of affairs, versus the likelihood of an outcome in the absence of that state of affairs. Bayes can help jurors assess how much weight to attach to new evidence, measuring the chance of its appearance if the defendant is innocent against the chance of its appearance if the defendant is guilty.
Crucially, Bayesian theory shows that the RMP of a DNA test is not the same as the probability that a person who gets a match is, in fact, the source of the DNA. Conflating these two scenarios is an example of what has been called “the prosecutor’s fallacy”: failing to appreciate the difference between the probability that someone who actually fits the evidence (A) is innocent (B), versus the probability that an innocent person picked off the street would fit the evidence. In mathematical notation, this is represented by P(B|A) ≠ P(A|B).
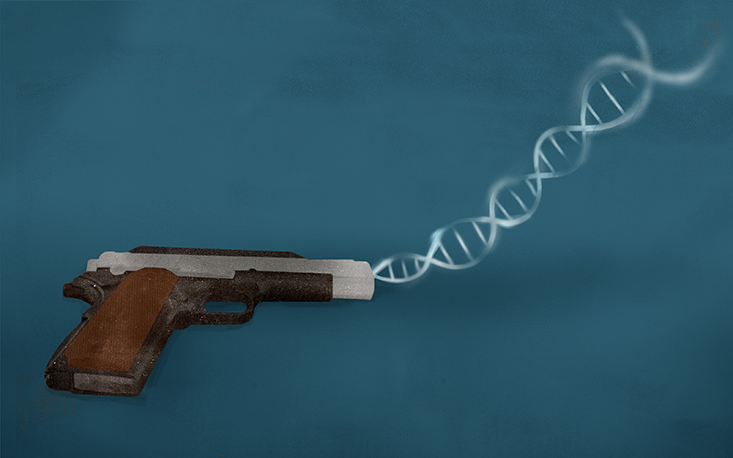
This distinction presented itself in a notable British case in the 1990s. Early on the morning of April 6, 1991, Miss M (as she was later identified in the court documents) was returning home from a night out with friends in London. A man approached her to ask for the time. As she looked down at her watch, he grabbed her suddenly from behind, threw her to the ground, and raped her before running away.
When Miss M reported the rape to the police, she was able to give them a rough description of her attacker. She said he was in his early 20s, Caucasian, and clean-shaven, with a local accent. In addition, the forensic scientists collected a semen sample of the attacker from Miss M’s body. This produced a DNA profile that was eventually found to match that of a local man, Andrew Dean (not his real name), whose profile was added to the DNA database after the crime for an unrelated sexual offense.
The RMP given in the Dean case was 1 in 200 million, which indicates a very good match. In the United Kingdom, with a population of 60 million, it would be conceivable but unlikely to find another person with the same DNA profile. But there were some problems with Dean’s identification. Miss M was unable to pick him out in a police lineup. When Dean was pointed out to her, she said that he could not be her attacker, who was “much older.” Dean was not in his 20s but was, in fact, 37 years old. Furthermore, he had an alibi, a girlfriend who said that she had spent that night together with him.
At Dean’s trial, the jury effectively had to weigh two different types of evidence against one another: the DNA match, which pointed toward guilt, and the non-scientific evidence, which pointed toward innocence—the alibi, and the fact that Miss M had been unable to recognize Dean.
The defense proposed balancing this evidence using Bayesian reasoning. It called Peter Donnelly, a professor of statistics at the University of Oxford, as an expert witness. Using Bayes, he said, one could assess how a new piece of evidence ought to affect the likelihood of guilt by multiplying the prior odds of guilt by the “likelihood ratio” corresponding to the new material. The likelihood ratio measures the relative chance of seeing a piece of evidence if the defendant is guilty, compared to the chances of seeing it if he is innocent. It can be calculated as follows:
(probability of seeing that evidence if the defendant is guilty)
÷
(probability of seeing that evidence if the defendant is innocent)
Donnelly then distributed a questionnaire in which the jurors were invited to use the above calculation in relation to the pieces of evidence. He also brought calculators for each of the jury members and the judge, and ran them through an illustrative example, though he encouraged the jurors to make their own individual estimates for the probabilities involved. (When he reached a point where the calculators “should now show the value of 31.6,” the judge cried out, “but mine just shows zero!”)
At Dean’s trial, the jury effectively had to weigh two different types of evidence against one another: the DNA match, which pointed toward guilt, and the non-scientific evidence, which pointed toward innocence.
At the beginning of the trial, Donnelly suggested, the presumption of innocence meant that they could assume that the defendant was no more likely to be guilty than any other male in the region. Given the number of local men in the area (approximately 150,000) and the probability that Dean was a local man (Donnelly put it at about 75 percent), Donnelly suggested that the probability of Dean’s guilt was approximately 1 in 200,000—or, 1 ÷ (150,000 ÷ 0.75).
Next, each of the pieces of evidence presented in court generated a likelihood ratio, which could be multiplied sequentially in order to “update” the assessment of guilt. Regarding the impact of Miss M’s failure to identify Dean, Donnelly gave an illustrative probability of such a failure, in the case of Dean’s guilt, of 10 percent (on the top of the fraction) and the probability of such as a failure in the case of Dean’s innocence as 90 percent (on the bottom of the fraction). This produced a likelihood ratio of Dean’s guilt of 1 in 9.
Then, performing the same calculations for Dean’s girlfriend’s assertion that they spent the night together, Donnelly estimated that a guilty defendant would have a 25 percent chance of adducing this evidence, while an innocent defendant would have a 50 percent chance. This put the likelihood ratio for Dean’s alibi as 25 in 50, or 1 in 2.
Thus, before the introduction of the DNA evidence, a juror might have estimated the chance of Dean’s guilt as the product of:
(1/200,000) x (1/9) x (1/2) = 1/3,600,000, or one in 3.6 million.
Now, calculating the weight that the jury should attach to the DNA match could be done in the same way. On the top of the fraction is the probability that the DNA sample would correspond to Dean if he were guilty, which is equal to 100 percent. On the bottom of the fraction is the probability of a match if Dean were innocent. This is the number measured by the RMP, namely 0.0000005 percent (or 1 in 200 million). This calculation produced a likelihood ratio for guilt associated with the DNA evidence equal to 200 million to 1 (or 1 in 200 million for Dean’s innocence).
So, the chance that Dean is guilty can be calculated by multiplying the likelihood ratios for these pieces of evidence together:
(1/3,600,000) x (200,000,000/1)
= 200 ÷ 3.6
= 55.55, or approximately 55 to 1 in favor of guilt.
In other words, using Donnelly’s figures and his Bayesian analysis, there would be a 1 in 55 chance that Dean was innocent, despite the good match for his DNA sample. This would have made it significantly more likely that he was innocent than if the jury had only considered the RMP, of 1 in 200 million.
Despite Donnelly’s testimony, the jury in the Dean case voted to convict. His conviction was quashed on appeal, however, and he was sent back for retrial—where he was convicted a second time, and his appeal rejected. In each case, the appeal judges expressed dislike of the Bayesian method, arguing that “Jurors evaluate evidence and reach a conclusion not only by means of a formula, mathematical or otherwise, but by the joint application of their individual common sense and knowledge of the world to the evidence before them.”
Courts in both the United States and Britain are leery of letting juries rely too heavily on probabilistic expert evidence. They seem to fear that math might put too much power into the hands of experts, and turn jurors into mechanical number-crunchers rather than feeling, reasoning, sensitive, and sensible adjudicators. This skepticism has a long tradition dating back to at least the jurist Laurence Tribe, whose 1971 Harvard Law Review article, “Trial by Mathematics: Precision and Ritual in the Legal Process” argued that the seeming “inexorability” of numbers may “intimidate” juries and erode the “sense of community values” that they are meant to bring to their decision-making.
Judges’ mistrust of mathematical reasoning is not the only obstacle to its use in the courtroom. Mathematicians themselves haven’t managed to reach an agreement on the appropriate form of Bayes’ theorem to use, or the best way to explain their calculations to juries. Even so, probabilistic reasoning remains a powerful tool to counter the shortcomings of human intuition about unlikelihood, and finding the right role for it is an urgent problem to be resolved—especially in a climate where DNA-match evidence is viewed as so damning that it often eclipses everything else.
Leila Schneps is a mystery writer and mathematician at the Institut de Mathématiques de Jussieu in Paris. Coralie Colmez is a math tutor in London. Colmez is Schneps’ daughter, and both are member of the Bayes and the Law Research Consortium. They are the authors of Math on Trial: How Numbers Get Used and Abused in the Courtroom, published in 2013.