Tell it to me in Star Wars,” Tracy Jordan demands of Frank Rossitano in an episode of 30 Rock, when Frank tries to explain the “uncanny valley.” As Frank puts it, people like droids like C-3PO (vaguely human-like) and scoundrels with hearts of gold like Han Solo (a real person), but are creeped out by weirdly unnatural CGI stormtroopers (or Tom Hanks in The Polar Express). More than a decade after that scene aired, Star Wars is still clambering out of the uncanny valley: Most fans were thrilled to see a digital cameo from a young Luke Skywalker in The Mandalorian. But he—along with recreations of young Princess Leia and Grand Moff Tarkin from the original Star Wars film—didn’t look real, which for some was unnerving.
These are all examples of what have come to be known as deepfakes, a catchall term for synthetically manipulated or generated images or videos of people or just about anything. The name derives from the fact that most methods for creating deepfakes rely on deep neural networks, the same basic architecture that underlies most of the AI we encounter on a daily basis, like face and voice recognition. And because the technology is constantly and rapidly improving, so are the deepfakes. Deepfake Luke recently appeared again in The Book of Boba Fett, just about a year later, looking impressively realistic.
Human and computer perception is fundamentally different.
Currently, one of the most advanced methods for creating deepfakes is called a generative adversarial network. It’s actually two separate neural networks paired together: one network might generate fake faces (the generative part) and the other tries to discriminate the fake faces generated by the first network from a set of real faces in a database. Like training with a partner, over time, both networks get better together at their respective tasks (the adversarial part). The discriminator network gradually gets better at telling the fake faces from the real faces. And the generator network gradually gets better at fooling the discriminator by generating more realistic fake faces.
Great for the scrappy little generator network. Good for Star Wars fans and the now immortal Luke Skywalker. Possibly bad for society.
Creating digital actors for TV shows is relatively harmless. But the power to create convincing deepfakes of world leaders, for example, could severely destabilize society. An inflammatory and well-timed deepfake video of a politician could hypothetically swing an election, spark riots, or raise tensions between two countries to volatile levels.
Whether this is a serious concern yet depends on how good current deepfake technology is and how good people are at discerning fakes from the real thing. In two recent studies, researchers asked thousands of people to judge the authenticity of real and fake images and videos to try to answer this question.
The most recent study, by psychologist Sophie Nightingale of Lancaster University and computer scientist Hany Farid of the University of California, Berkeley, focused on deepfake images.1 In one online experiment, they asked 315 people to classify images of faces, balanced for gender and race, as real or fake. Shockingly, overall average accuracy was near chance at 48 percent, although individuals’ accuracy varied considerably, from a low of around 25 percent to a high of around 80 percent.
In a follow-up experiment, 219 different people did the same task but first learned about features to look for that can suggest an image is a deepfake, including earrings that don’t quite match, differently shaped ears, or absurdly thin glasses. People also received corrective feedback after submitting each answer. “The training did help, but it didn’t do a lot,” Nightingale told me. “They still weren’t performing much better than chance.” Average accuracy increased to only 59 percent.
And those bizarre telltale deepfake features that people learned to spot? As technology improves and deepfakes become ever more flawless, “these artifacts are going to get sparser,” Nightingale said. “And we’re going to be back to that initial [accuracy] level, in not too long.”
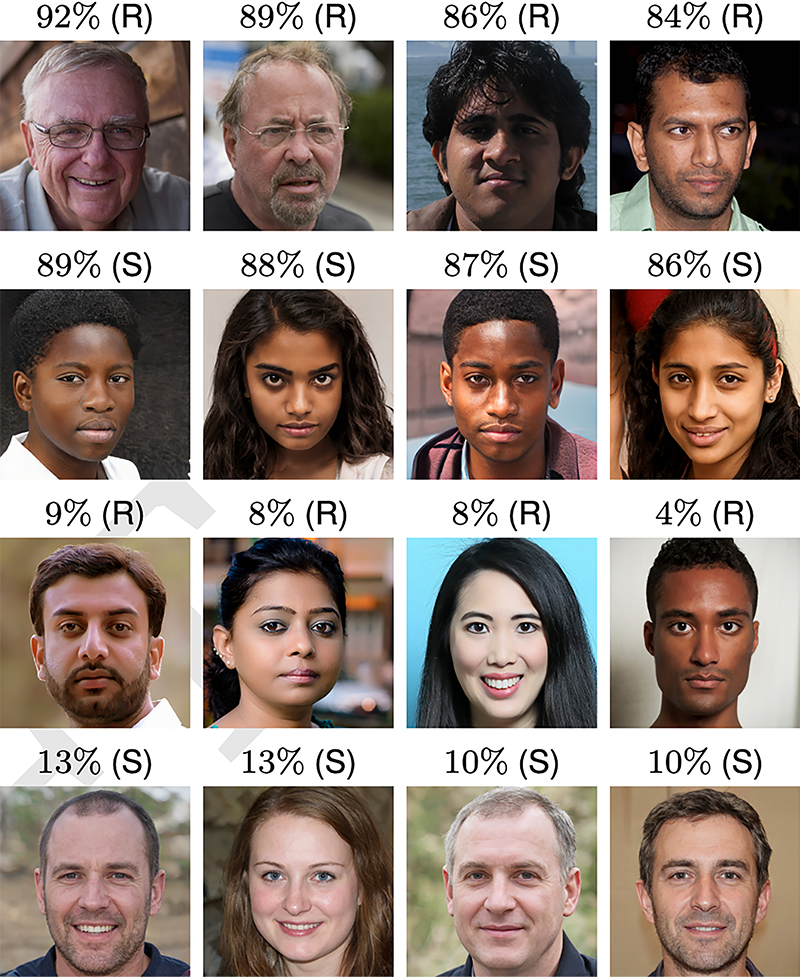
But there’s a difference between an image and a video. Part of the reason it remains so difficult to make a believably realistic recreation of young Luke is because he has to emote and speak. Even in The Book of Boba Fett, producers clearly recognized the limits of their illusion, frequently cutting away from Luke’s face whenever he had extended dialogue.
In December 2021, a team of researchers from MIT and Johns Hopkins, led by Ph.D. student Matt Groh, ran a similar study of people’s accuracy at identifying deepfakes, this one focusing on videos.2 They set up a website where people could rate the authenticity of real and deepfake videos randomly selected from Meta’s Deepfake Detection Challenge dataset. Like in the study of images, people watched videos, one at a time, judged whether they were real or fake, and received corrective feedback. More than 9,000 people around the world found the website on their own and collectively completed more than 67,000 trials. What’s more, the researchers recruited 304 nationally representative online participants who collectively completed about 6,400 trials.
Overall, average accuracy (weighted by people’s confidence judgments) was between 66 percent and 72 percent, depending on the participant group—slightly higher than in the study of images, but the stimuli in the two studies varied in multiple ways, so it’s hard to directly compare them. To get some sense of whether these accuracy numbers were any good, the researchers compared people’s judgments to those of one of the best performing computer models for detecting deepfakes. After feeding the same videos that people saw into the model, it beat most people, achieving an accuracy of 80 percent.
The best performer of all, though? One that incorporated human and computer judgments. To accomplish this, the researchers asked people to make a second rating of each video. After their initial rating, the researchers showed people the likelihood assigned by the computer model that the video was a deepfake and asked people if they wanted to change their ratings; they often did. The average of these updated ratings slightly out-performed both the model and people’s original ratings. This result suggests that both people and the computer model brought unique strengths to the task of detecting deepfakes.
At this point, you might be thinking that people are just irredeemably bad at this task. But many researchers believe that we are basically expert face detectors. Some neuroscientists have argued that we even have a brain region specialized for face processing. At the very least, it’s clear that people process faces differently than other types of visual information. We’re such expert face detectors that we can’t help but see faces everywhere: in rock formations on Mars, in the headlights and grilles of cars, and in misshapen vegetables.
The computer-generated faces tend to be more symmetrical, which we are biased to trust.
Groh, the study’s lead researcher, told me that these observations are exactly what motivated them: Because people seem to process faces in a specialized way, they might not be as easily fooled by deepfakes involving faces compared to other kinds of misinformation. And the strengths that the computer model brings? For one, it doesn’t suffer from the same limitations as our visual systems: “On videos that are super dark or blurry, that’s not really so much of an issue for the computer,” Groh explained.This is just one example of how human and computer perception is fundamentally different. Clearly people make mistakes that computers don’t. And yet sometimes computers make mistakes people would never make. AI mistakes are even an active area of research. For example, some people work on so-called adversarial images for computer vision systems: specially designed images that break image-recognition models. An adversarial image might be a photo of a lizard that an image-recognition model is 99 percent sure is a lizard. Then, after tweaking a handful of carefully chosen pixels in the image that are virtually imperceptible to any human, the model is suddenly 99 percent sure it’s a snail.
People are also excellent at incorporating context and background knowledge into their judgments in ways that computers mostly aren’t, and in ways that the sorts of tasks in these studies mostly don’t assess. One exception is a pair of deepfake videos of Vladimir Putin and Kim Jong-un included in the video study. People were much better than the model at classifying these videos. Whereas a majority of participants correctly spotted these fakes, the model was way off, highly confident that they were real.
Here, people may have taken into account factors like their memories of previous statements made by these leaders, or knowledge of what their voices sound like, or the likelihood that they would say the sorts of things shown in the video: all things not included in the model at all.
People’s sensitivity to these contextual factors underscores why even a flawless deepfake Luke in a future season of The Mandalorian wouldn’t really fool anyone: Everyone knows that Mark Hamill doesn’t look like that anymore.
Even if people have some advantages when it comes to processing faces, we have some liabilities, too. Nightingale and Farid conducted one more experiment to see if people might intuitively recognize the difference between deepfakes and real faces. They asked 223 people to rate the trustworthiness of 128 faces from the same set of images as in their previous experiments. People rated the deepfakes about half a point more trustworthy on a seven-point scale, on average, than the real faces.
Nightingale speculated the reason for the difference has to do with our well-documented preference for more average and symmetrical faces: “Think about how synthetic faces are generated: They’re basically an averaging process,” she said. “You take lots of images, lots of real images, you train a GAN [generative adversarial network], and then it uses that to produce synthetic faces.” The resulting faces tend to be more average-like and symmetrical, which we are biased to trust.
Arguably, both studies overstate and understate the seriousness of the problem. On one hand, unlike in these studies, far less than half the faces we see online are deepfakes, so our real-world accuracy is bound to be much higher, even if we just assume everything is real. But, unlike in these studies, Groh pointed out to me that under normal circumstances, we’re rarely so concerned with judging the accuracy of the media we encounter. This could explain why some people have already lost money to scammers using deepfakes, even though the stakes of these scams are much higher than in an online experiment.
Even worse, our ability to accurately judge real from fake may not even matter. For example, while people are reasonably good at judging the accuracy of news headlines, what people share on social media depends more on whether it agrees with their partisan beliefs than on whether it’s actually true.3 When it comes to deepfake misinformation, Groh told me, “if it’s emotionally charged, and it’s motivating, it’s likely that people might share stuff without even thinking about whether they believe in it or not.”
Is there anything that engineers and policy makers can do about the potential threat that deepfakes pose? Nightingale suggested developing ethical guidelines for using powerful AI technology or building watermarks or embedded fingerprints into the deepfake algorithms so they’re easier to identify. However, she thinks there are likely other solutions. “That’s why I’d like to almost get that idea out there that collaboration on what we do next is quite important,” she said. “Because I want people to be aware of the problems.”
“Have we passed through the uncanny valley?” Nightingale asked me. It was a rhetorical question. She said, “It turns out we’re pretty much on the other side.”
Alan Jern is a cognitive scientist and an associate professor of psychology at Rose-Hulman Institute of Technology, where he studies social cognition. Follow him on Twitter @alanjern.
Lead image: Massimo Todaro / Shutterstock
References
1. Nightingale, S.J. & Farid, H. AI-synthesized faces are indistinguishable from real faces and more trustworthy. Proceedings of the National Academy of Sciences 119, e2120481119 (2022).
2. Groh, M. , Epstein, Z., Firestone, C., & Picard, R. Deepfake detection by human crowds, machines, and machine-informed crowds. Proceedings of the National Academy of Sciences 119, e2110013119 (2021).
3. Pennycook, G., et al. Shifting attention to accuracy can reduce misinformation online. Nature 592, 590-595 (2021).