Tanya Berger-Wolf didn’t expect to become an environmentalist. After falling in love with math at 5 years old, she started a doctorate in computer science in her early 20s, attracting attention for her cutting-edge theoretical research. But just as she was about to graduate, she became obsessed with a topic that surprised her professors and even herself: zebras.
While still an undergraduate, Berger-Wolf began working as a research assistant at the ecology department, building computer simulations of wildlife populations. She was intrigued by the fact that digital technologies and biodiversity were following exponential trends, but in opposite directions. While the digital sector was burgeoning, endangered species populations were crashing. And in contrast to the deluge of data she had experienced in computer science, Berger-Wolf was shocked at how little data existed about the world’s most endangered species.
The animal that caught Berger-Wolf’s attention, the Grevy’s zebra, was known in antiquity as the imperial zebra. Used by Romans in their circuses, the largest of the wild equines, renowned for their elegant stripes and striking gait, the Grevy’s zebras once roamed large expanses of East Africa in giant herds. Today, fewer than 1,000 zebras remain, crowded out by farmers’ fields and cattle ranges, and still hunted for their skins and meat. By the time Berger-Wolf learned about their plight, scientists were predicting that the iconic species might die out within two decades.
How, exactly, could computer science help save endangered species?
Maybe, Berger-Wolf thought, she could apply her digital skills in a way that could help save the zebras.
But the computer scientists she consulted were discouraging. As one told her: “You’re smart enough to do theory; why do you want to do this applied crap?” Others advised her to transfer to computational biology. Berger-Wolf tried it out, only to realize that she didn’t want to spend her time sequencing the human genome or creating more accurate models of the human brain. She wanted to do something that would combine ecology and computer science to help save the planet.
The field of research she imagined had no name, nor precedent. Undaunted, Berger-Wolf asked around: “Who is the best ecologist in the world?” She was given the name of Simon Levin, at Princeton University. Berger-Wolf wrote to Levin out of the blue with an unorthodox idea: They would invent a new field, called computational ecology—a research agenda as ambitious as computational biology, but applied to ecological issues. Levin accepted, and Berger-Wolf moved to Princeton.
Even there, it was an uphill battle. Most ecologists saw computer scientists as mere coders. And most computer scientists saw ecologists as mere sources of data. Berger-Wolf couldn’t convince her fellow computer scientists of her vision’s potential. And the ecologists, although intrigued, were dubious: How, exactly, could computer science help save endangered species?
Zebras were Berger-Wolf’s breakthrough. Shortly after arriving at Princeton, she began chatting with Daniel Rubenstein, one of the world’s leading behavioral ecologists. She explained the problem to him. Ecologists wanted the Kenyan government to adopt stronger protections, including a national park. But the Kenyan government demanded an accurate census before proceeding with regulations that would likely incite strong resistance from local communities.
Why didn’t I think of it before? All we need is a bar code reader for zebras!
Here, the ecologists faced a catch-22. The usual census methods (catching the zebras and painting numbers on them or shooting them with anesthetic darts in order to embed electronic tracking devices) were expensive, traumatizing, and put the zebras at risk of infection. Given how endangered the zebras were, these conventional methods couldn’t be used. But visual surveys were slow, expensive, and inaccurate; it could take up to half an hour to identify a zebra from a photo, and the people-shy zebras were notoriously hard to track. The herd’s babies were dying at an alarming rate, yet without a census the government would not act. Unless something changed, the zebras were doomed.
How could computer science help? Berger-Wolf’s epiphany emerged during a field computational ecology course that she and Rubenstein co-taught in Kenya. By then, she had met and married her husband—an ecologist with an interest in zebras. One night, Berger-Wolf overheard her husband joking with some local field biologists as they gathered to identify zebras from individual photos, a tedious task that would take many days. All they needed, they mused, was an automated method to help them identify and catalog individual zebras. It had been yet another frustrating day tracking the elusive animals, and Berger-Wolf overheard her husband say: “Why didn’t I think of it before? All we need is a bar code reader for zebras!”
A light bulb went off for Berger-Wolf. Zebras did indeed have unique stripe patterns. Although a bar code reader probably wouldn’t work, something like a fingerprint scanner potentially could.
Theoretically, zebra stripes pose a similar conceptual problem to the identification of human fingerprints, as each individual zebra has its own unique markings. While biologists had long used these patterns (which they sometimes refer to as “bodyprints”) to help identify individual zebras in the wild, the process had never been automated.
Berger-Wolf began collaborating with her Ph.D. student Mayank Lahiri to develop an initial iteration: StripeSpotter. Their goal was to create a free, open-source program to which anyone could upload a photo of a zebra’s flank, for automatic identification. The algorithm is fairly straightforward: The zebra is assigned a “stripe code,” which is then checked against the database. If the zebra is already in the database, it is matched to earlier photos; if not, it is assigned a new, unique identity. The AI-powered algorithm can identify individual zebras from a simple photo and has no problem handling images of different sizes, oblique angles, and over- or underexposed photographs. After testing it against thousands of zebra photos that she took from various angles, including hundreds of photos she took personally during several super-light airplane flights over northern Kenya, Berger-Wolf verified the accuracy of the algorithm. With enough photos, a complete census of the zebra population was now feasible.
Berger-Wolf then teamed up with Chuck Stewart, a computer scientist at Rensselaer Polytechnic; together with his student Jon Crall, they decided to develop a more comprehensive computer vision method for identifying patterned animals, which they called HotSpotter. The near-impossible task of identifying and tracking individual animals, which used to take weeks or months, now takes mere milliseconds. As reliable as facial recognition technology, computers could now identify zebras as individuals.
Berger-Wolf knew that her idea was highly scalable. In theory, the HotSpotter algorithm could be adapted and applied to many of the nearly 9 million known species on the planet. Moreover, manual photos could be augmented with photos by satellites or drones and crowdsourced from social media. In other words, it could become a low-cost face recognition reader for the world’s wildlife. She was just missing one key component: a robust data management system.
A chance encounter led her to the solution. At around the same time Berger-Wolf had dreamed up StripeSpotter, Princeton-trained physicist Zaven Arzoumanian developed an unexpected passion for endangered whale sharks.
Whale sharks are mysterious creatures: Although they are the largest living fish in the world (with adults longer than 40 feet weighing in over 20 tons), little is known about them—except that global populations have halved in the past few decades. Arzoumanian’s interest was sparked when a friend, software programmer Jason Holmberg, had a numinous encounter with a whale shark while scuba diving. Holmberg began wondering about how the highly elusive fish might be tracked, and asked Arzoumanian—by then working at NASA’s Goddard Space Center—for help.
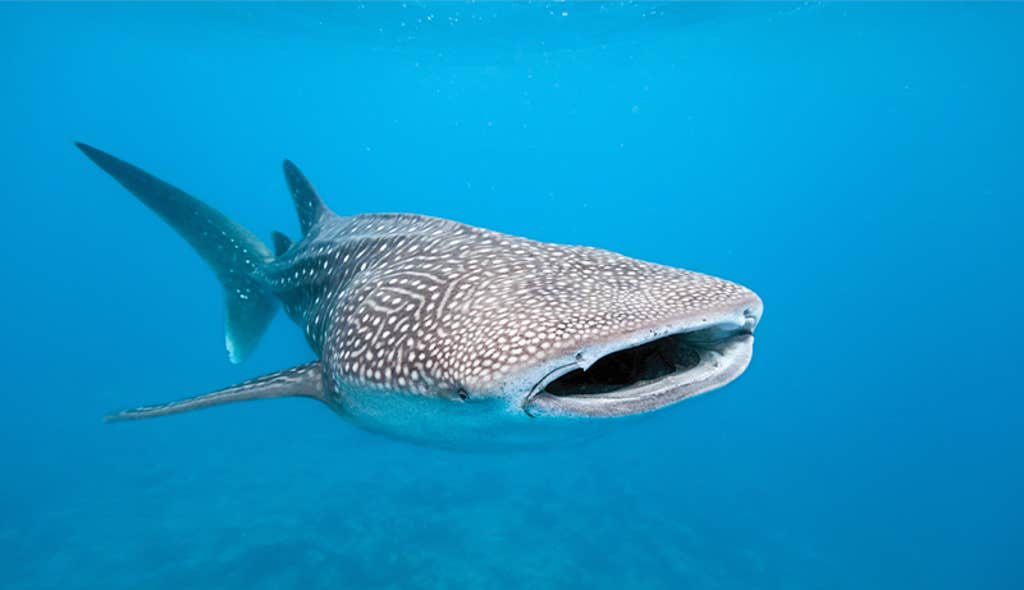
How could they automate identification of whale sharks? The creatures have hundreds or even thousands of distinctive white spots on their backs, as unique as human fingerprints, but the subtle variation is hard for human eyes to parse and differentiate.
After searching through the literature, Arzoumanian homed in on an innovative stellar pattern-matching algorithm that had been developed decades earlier for use by astrophysicists. When Princeton scientist Edward Groth first created the algorithm, he was trying to automate the analysis of the billions of stars revealed by the newly launched Hubble Space Telescope.
The observable universe as a whole contains somewhere between 30 billion trillion and 70 billion trillion stars; the sheer volume of information overwhelmed astronomers. Groth’s algorithms solved this challenge; Arzoumanian realized that the algorithm, which analyzes patterns of pinpricks of light in the sky, could be used to identify individual whale sharks, whose dappled skins have unique patterns that are as intricate and unique as stellar constellations.1
Holmberg and Arzoumanian tweaked the algorithm, and then reached out to whale shark biologist Brad Norman, who immediately saw the enormous potential in citizen science outreach: Norman’s large network of amateur whale shark spotters could upload photographs, and the whale spotter algorithm could identify individual sharks in seconds.
For decades, Norman had been photographing and identifying each whale shark by eye, a tedious process that could take hours or even days; he was eager to try an automated method. He soon launched a global campaign. A year later, with the contributions of more than 5,000 citizen scientists who reported tens of thousands of whale shark encounters in dozens of countries, more than 6,000 individual whale sharks were identified, with a successful match rate of over 90 percent.
The number of known whale shark gathering sites doubled within a few years—a finding that would not have been possible using manual observation methods.2 Says Norman, “Whale sharks stopped being random animals . . . and became individuals with stories and histories and futures that are yet to be written. And that’s what makes it so seductive as a citizen science project.”3
With the help of over 8,000 citizen scientists, and by scraping YouTube videos, researchers were able to identify over 12,000 individual whale sharks from over 75,000 reported sightings. Those data led the International Union for Conservation of Nature (IUCN) Red List of Threatened Species to reclassify the whale shark from vulnerable to endangered and to determine that the population trend was declining, rather than stable as previously thought.
Based on their initial success with whale sharks, Arzoumanian and Jason Holmberg founded a nonprofit organization, Wild Me. But they hit a stumbling block: just like StripeSpotter, their original algorithm didn’t scale. However, Holmberg had created a robust data management layer, just what Berger-Wolf needed. The three innovators teamed up, and the constellation and StripeSpotter algorithms were replaced with the HotSpotter-based approach, linked with the Wild Me data management layer. They now had a system that could potentially catalog any living thing (anything, that is, with colors, stripes, spots, wrinkles, scars, or notch patterns that do not change as the animal ages).
Then, they embarked on an ambitious mission: cataloging the world’s wildlife. The pent-up need for automated animal identification systems was staggering. Berger-Wolf’s email was jammed with requests. Researchers sent in massive datasets of photos from all over the world. The huge amounts of data now available were both blessing and curse: The Wild Me team now had a massive cataloging problem, and it still wasn’t obvious how conservationists could use the information. So the team, which had by this point attracted half a dozen engineers, created Wildbook, a version of Facebook for animals.
The founders’ vision was ambitious: blend wildlife research with citizen science and computer vision to accelerate zebra monitoring. They billed it as extinction-combating software. Their ultimate goal was to create a universal animal recognition algorithm that could identify unique individuals in every species on the planet, like a low-cost facial recognition reader for the world’s wildlife. With such an algorithm, ecologists would be able to easily and automatically identify and track any individual living creature on the planet, from birth to death.
Several years later, Wildbook encompasses hundreds of species. (If you’re a fan of whales or dolphins, check out Flukebook.) The social media side is the visible part of the website, where members of the public can visit and perhaps fall in love with some charismatic megafauna (or microfauna). At the back end, out of sight, is a set of algorithms that deploy computer vision, combined with citizen science, as a way to put a face, name, and story to each and every individual animal on the planet.
Whale sharks stopped being random animals and became individuals with stories and futures.
The platform is now being used to track a Noah’s ark-like list, including whales, giraffes, manta rays, humpback whales, Hector’s dolphins, sea bass, flapper skates, turtles, sharks, jaguars, lynx, seals, polar bears, and sea dragons. With funding from the Moore Foundation, Berger-Wolf is expanding Wildbook to thousands of species. In the future, she hopes to develop systematic assessments of populations for every species on the IUCN Red List, a global inventory of endangered species.
Another funder of Wildbook has been Microsoft’s AI for Earth program. Why did Microsoft decide to get involved? In the words of Josh Henretig, who oversaw the $50 million fund Microsoft is investing in AI for Earth: “What’s really worrying to us and to many scientists around the world is that we have only discovered/described about 1.5 million species of an estimated 10 million on our planet, and less than 5 percent of that 1.5 million species have ever been analyzed in any detail. There are species that are disappearing off our planet that we’ve never even known about.”
Many researchers are now following in Wildbook’s footsteps, developing machine learning algorithms to track specific species of wildlife (chimps, dolphins, badgers, birds, koalas, kangaroos), and even track the exotic pet trade. These efforts are not enough to stop extinction, but they are one important piece in the larger puzzle: accurate documentation of species decline.
In the meantime, the Grevy’s zebras now have a fighting chance at survival.
Soon after the launch of HotSpotter, Dan Rubenstein came up with a controversial suggestion: convince the Kenya Wildlife Service to incorporate HotSpotter into a biannual zebra population census. As Berger-Wolf remembers, “The computer scientists on our team nearly had a heart attack when Dan proposed a census.” But Rubinstein insisted. They needed to convince the government to do a census, and they also needed to win over the public by engaging ordinary Kenyans in the process.
Although dubious, Berger-Wolf approached the Kenyan government, and to her surprise found some interest. After two years of negotiations and planning, the first two-day Great Grevy’s Rally was held across Kenya in 2016. The rally was cast as a blend of citizen science and public relations, mobilizing hundreds of Kenyans, from the prime minister to children from the slums of Kibera, to snap photos of the elusive zebras in a two-day national campaign. The rally produced an unprecedented result: a verifiable, accurate census (rather than estimate) of the entire Grevy’s population.
Over subsequent years, its accuracy was confirmed; meanwhile, the political popularity of the zebra grew. As Berger-Wolf recounts, the youngest participant in the most recent rally was 3 years old, while the oldest was over 90 years old. The Kenyan government was able to enact reforms that were previously thought to be too unpopular to be feasible: passing a new Grevy’s Zebra Endangered Species Management Plan (committing land, resources, and funding) and strategically limiting lion populations (a major zebra predator) with contraception.
A government census in 2020 confirmed that—for the first time in years—the Grevy’s zebra population in Kenya had stabilized rather than continuing to decline.
This excerpt is taken from Gaia’s Web: How Digital Environmentalism Can Combat Climate Change, Restore Biodiversity, Cultivate Empathy, and Regenerate the Earth by Karen Bakker, published with permission from The MIT Press.
Lead image: slowmotiongli / Shutterstock
References
1. Arzoumanian, Z., Holmberg, J., & Norman, B. An astronomical pattern-matching algorithm for computer-aided identification of whale sharks Rhincodon typus. Journal of Applied Ecology 42, 999–1011 (2005).
2. Norman, B.M. et al., Undersea constellations: The global biology of an endangered marine megavertebrate further informed through citizen science. BioScience 67, 1029–1043 (2017).
3. Annie Sneed, A. Astronomy Tool Helps ID Sharks. Scientific American ( 2018).