If the rumors are true, 20th Century Fox will release a remake of the 1966 science-fiction film Fantastic Voyage in the next year or two. The conceit behind the film is that its protagonists are shrunk down and injected into the human body, through which they travel in a microscopic submarine. At that size, a swirl of blood turns into dangerous turbulence. White blood cells can engulf their ship. A droplet’s surface tension, formerly imperceptible, now forms an impenetrable barrier.
Changing scales disrupts our intuitive sense of what is significant, what is powerful, and what is dangerous. To survive, we must recalibrate our intuitions. Even if every effect at familiar scales is negligible, the slightly less negligible effect may become monstrously important at unfamiliar scales.
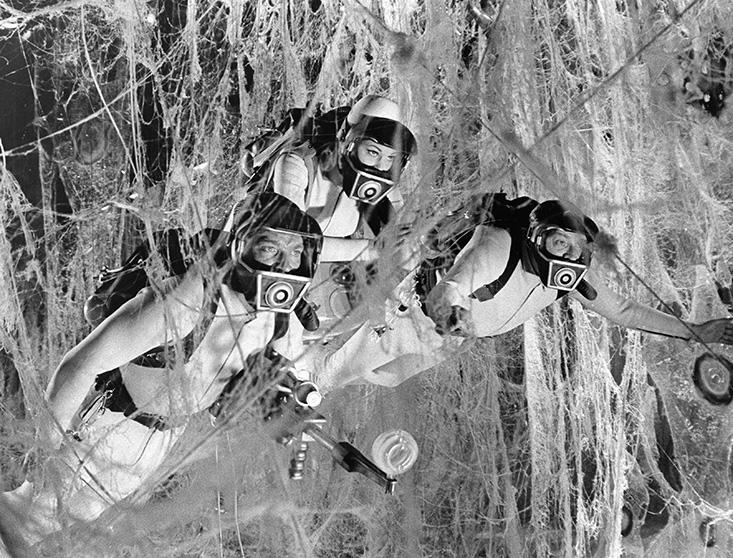
How can we understand what is important at unfamiliar scales? It turns out that there is a mathematical theory called large deviations theory that performs the same trick for probabilities as the shrink ray did for the crew of the Fantastic Voyage. Whereas classical probability theory concerns itself primarily with the likelihoods of ordinary events, large deviations focuses on the extreme rare events that emerge from the confluence of multiple slightly odd ones. It lets us zoom in our probability microscope to identify the least unlikely ways that the very, very unlikely could occur.
The theory, which has been the subject of intense study and development since its formulation 50 years ago by the mathematician S.R. Srinivasa Varadhan, shows us how average behavior of a random system may diverge from typical behavior. And by rigorously comparing all the rare possibilities, we see that often we underestimate the probabilities of unusual events when we confine our attention to the most superficially ordinary ways they could happen.
Microscope in hand, let’s go traveling.
The high-frequency trader
A high-frequency trader makes a long sequence of trades. On each one of them his fortune, which starts out at $1,000,000, increases by half a percent or decreases by half a percent, each with probability ½. How much is he likely to have after 1 million transactions?
He might reason: Each transaction goes up or down by the same amount, so on average it doesn’t change. On average he should still have $1 million.
Here is another argument: When he gets a winner his fortune is multiplied by 1.005; a loser multiplies it by 0.995. One of each multiplies it by 1.005 x 0.995 = 0.999975. In 1 million transactions there should be about 500,000 of each, so the original $1 million should turn into about $1,000,000 x (0.999975)500,000, which is about $3.73.
Which argument is correct? Surprisingly, both are, though the second is more relevant. The trader will almost certainly have nothing left, but if we zoom in among the set of very unlikely events where he comes out ahead, there are some outcomes in which he comes out ahead by a huge amount. The key is a function I(x), called the rate function, that tells you how the probability of getting an outcome like x declines as the number of trades increases. Here x is a number, but depending on the problem it might be a random life-course trajectory, a random network structure, or a random geometry of the universe. I(x)=0 corresponds to the typical case, whose probability is not tiny—in this case corresponding to the outcome where the fortune collapses exponentially fast. Larger values of I(x) correspond to exponentially more unlikely x.
It is the tradeoff between an exponentially decreasing probability and exponentially increasing fortune that determines the average. Some of the x are very big, even if the corresponding probability is small. Optimizing this tradeoff confirms the naïve intuition that the average outcome of the trading exercise is $1 million—even while we can be quite confident that any single trader will lose almost everything. If there are 1 million traders, though, all making their million trades with $1 million in capital, the average outcome will indeed be $1 million. This average turns out to be determined by the one or two traders who end up with several hundred billion dollars. Most of the money—hundreds of billions—will wind up in the accounts of a handful of random individuals, while the vast majority lose almost everything.
The chance of coming out ahead (or even) is less than 1 in 100.
The telephone exchange
A central problem for communications networks is to determine how likely they are to become overloaded. The data buffer connected to a telephone switchboard or Internet network may have a capacity sufficient for an average load level, but not sufficient to handle an unusual number of simultaneous users.
The Bell Labs mathematicians Alan Weiss and Adam Shwartz outlined the application of large-deviation theory to communication networks in 1995. The general theory tells us that the probability of a rare event tends to decline exponentially with the size of the system under consideration—in mathematical notation, the probability changes as e–n1(x), where n measures the size, x is a particular “pathway” to the rare event, and I is the rate function, giving the relative unlikelihood of that path. Rare events tend to happen in a predictable way—the one that minimizes the rate function—and to come in clusters, separated by long time intervals.
The challenge, in any given problem, is to identify (and successfully interpret) the rate function. The rate function gives the relative likelihoods of all sequences of flows, from which it is possible to find the overflow-producing combinations with the lowest rate function, meaning the highest probability. These combinations determine the frequency of overflows, and so also determine the nature of the overflow: How many sources will be active, which kinds of sources, and how quickly the overflow is likely to be resolved.
Rare events tend to happen in a predictable way, and to come in clusters, separated by long time intervals.
As a simple example, consider a telephone network where each of the large number of potential users—let us say, 1 million—connects at random times, so that on average they are connected about 1 percent of the time. (We assume they make their calls independently of each other, with equal chances at any time of day.) The network needs 10,000 communication lines to meet the average demand. The company calculates, using large-deviations, that if they make 10,500 lines available the network will be overloaded about 2 minutes per year.
Suppose now that in addition the network starts being used by half a million video gamers, who are connected about 1 percent of the time, but they need a huge bandwidth, taking up five communication lines each. The new users also require 10,000 lines on average, so the company decides to double its capacity, to 21,000. But the result is that the network is overloaded several minutes a week. Analyzing the rate function tells us as well that the video gamers—who on average use the same capacity as the others—will always be found to be using about 8 percent more lines at times of overload, and that an extra 250 lines will restore the smooth functioning of the network. If we plot the network load in the seconds before an overload, we will see that it almost always follows a certain pattern, curving gradually up before it crashes sharply into the upper bound—and this curve can also be calculated as the one that minimizes the rate function.
In modern, decentralized packet-switching networks the rate function can help to detect botnets, networks of virus-infected computers that criminal hackers deploy to send spam and to attack other systems. The idea is to identify the botnet master as a computer that communicates with an unusually large number of other computers, and then confirm the identification by finding unusual patterns of correlation among the computers it communicates with. In order to do that, researchers at Boston University needed to use a rate function that could describe, among all the ways an improbably large set of unrelated computers might happen to be communicating with the same distant server, what patterns of correlation among their communications are most likely.1
The dormant seed
Diapause is a delay in biological development, often at an early stage. Many plant species, for example, produce seeds that do not germinate immediately, but remain dormant for extended times, forming what are called persistent seed banks. Given that the struggle for survival, as in war, typically turns on who can “get there first with the most,” random dormancy is a bit of an ecological mystery.
To help clarify the issue, a paper by myself and Stanford biologist Shripad Tuljapurkar considered a very simple model: a species with a two-year life-cycle, in which the first year is spent growing from a seed to an adult, and the second year is spent producing seeds.2 We considered the question: What will be the effect on growth rates if a small fraction of the seeds remain dormant for one year?
In the case where growth, survival, and seed production are the same every year the answer is what you would expect: Delaying individual growth slows down population growth. Things look different, though, when environmental conditions are variable. Then even a tiny amount of delay will yield a substantial boost to population growth.
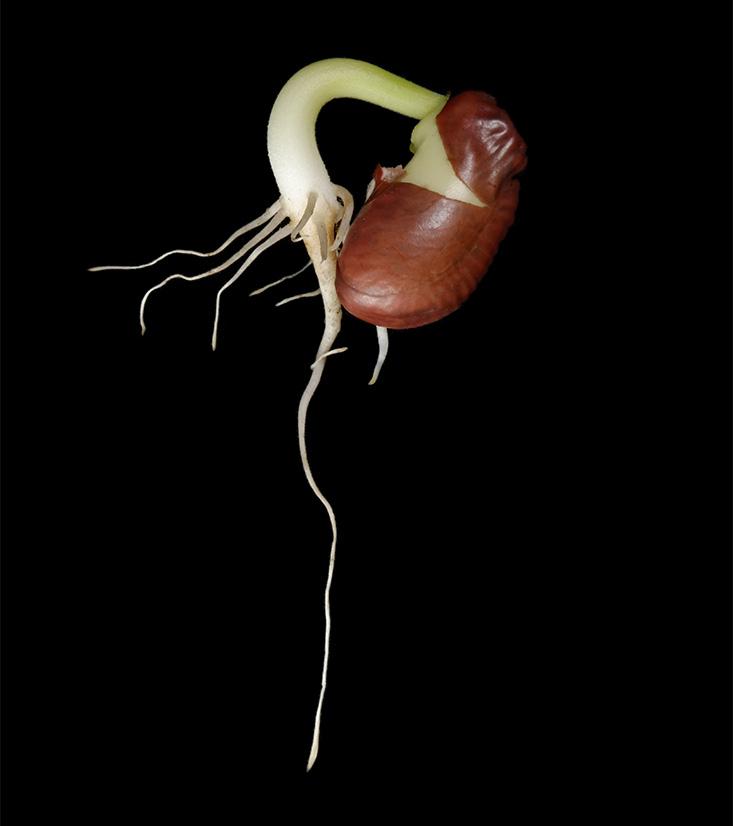
If 1 percent of seeds wait out the year, then we would expect that a typical genealogical trajectory will have about 1 delay per 100 years, and will experience fairly typical environmental conditions when they do mature. But there will be some extremely rare trajectories of successive generations of seed that delay more frequently, and happen to do so in just the worst years, when growing seedlings are almost sure to die, or produce no seeds as adults. These trajectories are large deviations—exponentially rare —but they produce exponentially more offspring over time. The population growth rate will ultimately be determined by these unlikely trajectories. To put it differently, if we look backward at the trajectory of an individual currently alive, it will look like a succession of lucky accidents.
The same mathematics applies to migration, supporting an important principle in habitat conservation: A species will benefit from the ability to wander between two equally good territories where environmental conditions vary randomly between them from year to year. Each individual, looking backward at its family history, will see ancestors who happened to flee one site, purely by chance, just before disaster struck, or who arrived in the other site just when food was particularly abundant. This is a special case of this bromide of evolution: The vast majority of organisms who ever lived died without reproducing, but you can trace your ancestry back for billions of generations without encountering a single one of them. Lucky you!
The centenarian
If you are past a certain age—an age that is younger than most people think, since the probability you’ll survive your next year of life peaks at age 12—your general fitness and likelihood to survive another year is trending downward day by day, even if you may sometimes improve over longer or shorter periods. Theoretical demographers have considered models of aging where an individual’s “vitality” is a random quantity that progresses by small steps, more likely to go down than up, with death more likely the lower the vitality sinks.
Unsurprisingly, when individuals follow this model, the average vitality of the population declines as a function of age … up to a point. At advanced ages we have only a small subset of the population still alive, and those are exceptional individuals. Maybe they started out exceptional, genetic lottery winners. Maybe they just became exceptional through the chance knocks of life happening to knock them in a relatively positive direction.
This suggests that the mortality rate increases with age through the adult years then levels off in extreme old age.
However it happens, the model predicts that the vitality of the survivors gradually stops declining. That is, each individual keeps declining, but those who decline are scythed off by the Grim Reaper. The overall vitality of the survivors settles into an equilibrium, called a quasistationary distribution, between the individual downward trajectory and the winnowing out of the low end of the vitality distribution through death.
Expressed in terms of large-deviations theory, there is a rate function I(x)—where x is now a record of vitality over a whole lifetime—that is zero for trajectories that stay close to the average. Those that deviate significantly from average have a positive rate function, meaning they are exponentially less likely. In a typical model we find that among all the life courses that last exceptionally long, the most likely are those that have, purely by chance, kept their vitality unusually high, rather than those that have followed the usual downward trajectory and happened not to die.
This suggests that the mortality rate—the probability of dying in the next year for an individual of a given age—increases with age through the adult years then levels off in extreme old age. Such a pattern, called a mortality plateau, is readily apparent in organisms such as fruitflies and roundworms when large numbers of them are observed under identical laboratory conditions—the mortality rate levels off in the most common laboratory fruitflies, Drosophila melanogaster, when they are only 4 weeks old.3
Human mortality plateaus didn’t become apparent until increasing populations and improving health conditions allowed a large number of individuals to reach 100 years old or more. In general, human mortality rates double about every 8 years between about the mid-30s and the mid-90s. If we look at the cohort of Americans born in 1900, their mortality rate at age 90 was about 0.16, meaning that about 16 percent of those still alive died in that year. That more than doubles to 0.35 at age 98, but then never quite doubles again. The highest recorded mortality rate for the cohort is 0.62, at age 108. The data get fairly thin out there, but careful analysis of supercentenarian (age 110+) mortality data collected from across the world has shown, reasonably convincingly, that under current conditions mortality rates level off somewhere between 0.4 and 0.7.4
David Steinsaltz is an associate professor of statistics at the University of Oxford. He blogs at Common Infirmities.
References
1. Wang, J. & Paschalidis, I.C. Botnet detection based on anomaly and community detection. IEEE Transactions on Control of Network Systems (2016). Retrieved from DOI:10.1109/TCNS.2016.2532804.
2. Steinsaltz, D. & Tuljapurkar, S. Stochastic growth rates for life histories with rare migration or diapause. arXiv:1505.00116 (2015).
3. Vaupel, J.W., et al. Biodemographic trajectories of longevity. Science 280, 855-860 (1998).
4. Vaupel, J.W. & Robine, J.M. Emergence of supercentenarians in low-mortality countries. North American Actuarial Journal 6, 54-63 (2002).
Lead image collage credit: Scitoys.com