On a chilly evening last fall, I stared into nothingness out of the floor-to-ceiling windows in my office on the outskirts of Harvard’s campus. As a purplish-red sun set, I sat brooding over my dataset on rat brains. I thought of the cold windowless rooms in downtown Boston, home to Harvard’s high-performance computing center, where computer servers were holding on to a precious 48 terabytes of my data. I have recorded the 13 trillion numbers in this dataset as part of my Ph.D. experiments, asking how the visual parts of the rat brain respond to movement.
Printed on paper, the dataset would fill 116 billion pages, double-spaced. When I recently finished writing the story of my data, the magnum opus fit on fewer than two dozen printed pages. Performing the experiments turned out to be the easy part. I had spent the last year agonizing over the data, observing and asking questions. The answers left out large chunks that did not pertain to the questions, like a map leaves out irrelevant details of a territory.
But, as massive as my dataset sounds, it represents just a tiny chunk of a dataset taken from the whole brain. And the questions it asks—Do neurons in the visual cortex do anything when an animal can’t see? What happens when inputs to the visual cortex from other brain regions are shut off?—are small compared to the ultimate question in neuroscience: How does the brain work?
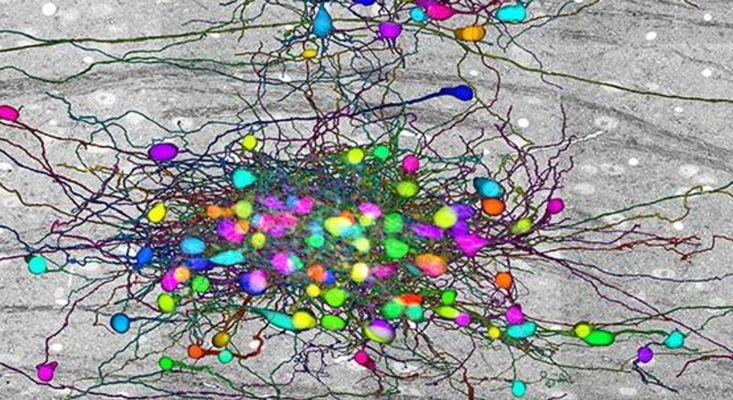
The nature of the scientific process is such that researchers have to pick small, pointed questions. Scientists are like diners at a restaurant: We’d love to try everything on the menu, but choices have to be made. And so we pick our field, and subfield, read up on the hundreds of previous experiments done on the subject, design and perform our own experiments, and hope the answers advance our understanding. But if we have to ask small questions, then how do we begin to understand the whole?
Neuroscientists have made considerable progress toward understanding brain architecture and aspects of brain function. We can identify brain regions that respond to the environment, activate our senses, generate movements and emotions. But we don’t know how different parts of the brain interact with and depend on each other. We don’t understand how their interactions contribute to behavior, perception, or memory. Technology has made it easy for us to gather behemoth datasets, but I’m not sure understanding the brain has kept pace with the size of the datasets.
I was now on a dark path, unsettled by a future filled with big data and small comprehension.
Some serious efforts, however, are now underway to map brains in full. One approach, called connectomics, strives to chart the entirety of the connections among neurons in a brain. In principle, a complete connectome would contain all the information necessary to provide a solid base on which to build a holistic understanding of the brain. We could see what each brain part is, how it supports the whole, and how it ought to interact with the other parts and the environment. We’d be able to place our brain in any hypothetical situation and have a good sense of how it would react.
The question of how we might begin to grasp the entirety of the organ that generates our minds has been pressing me for a while. Like most neuroscientists, I’ve had to cultivate two clashing ideas: striving to understand the brain and knowing that’s likely an impossible task. I was curious how others tolerate this doublethink, so I sought out Jeff Lichtman, a leader in the field of connectomics and a professor of molecular and cellular biology at Harvard.
Lichtman’s lab happens to be down the hall from mine, so on a recent afternoon, I meandered over to his office to ask him about the nascent field of connectomics and whether he thinks we’ll ever have a holistic understanding of the brain. His answer—“No”—was not reassuring, but our conversation was a revelation, and shed light on the questions that had been haunting me. How do I make sense of gargantuan volumes of data? Where does science end and personal interpretation begin? Were humans even capable of weaving today’s reams of information into a holistic picture? I was now on a dark path, questioning the limits of human understanding, unsettled by a future filled with big data and small comprehension.
Lichtman likes to shoot first, ask questions later. The 68-year-old neuroscientist’s weapon of choice is a 61-beam electron microscope, which Lichtman’s team uses to visualize the tiniest of details in brain tissue. The way neurons are packed in a brain would make canned sardines look like they have a highly evolved sense of personal space. To make any sense of these images, and in turn, what the brain is doing, the parts of neurons have to be annotated in three dimensions, the result of which is a wiring diagram. Done at the scale of an entire brain, the effort constitutes a complete wiring diagram, or the connectome.
To capture that diagram, Lichtman employs a machine that can only be described as a fancy deli slicer. The machine cuts pieces of brain tissue into 30-nanometer-thick sections, which it then pastes onto a tape conveyor belt. The tape goes on silicon wafers, and into Lichtman’s electron microscope, where billions of electrons blast the brain slices, generating images that reveal nanometer-scale features of neurons, their axons, dendrites, and the synapses through which they exchange information. The Technicolor images are a beautiful sight that evokes a fantastic thought: The mysteries of how brains create memories, thoughts, perceptions, feelings—consciousness itself—must be hidden in this labyrinth of neural connections.
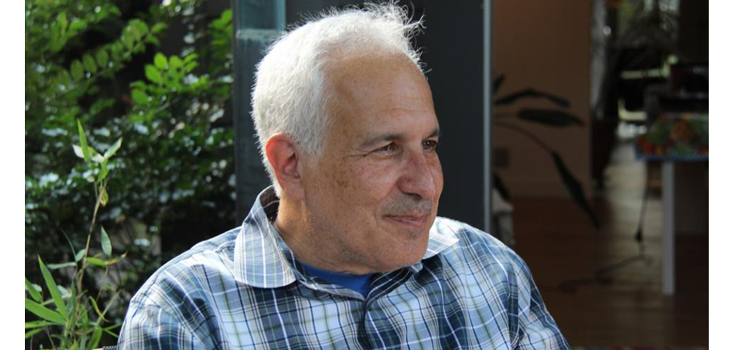
A complete human connectome will be a monumental technical achievement. A complete wiring diagram for a mouse brain alone would take up two exabytes. That’s 2 billion gigabytes; by comparison, estimates of the data footprint of all books ever written come out to less than 100 terabytes, or 0.005 percent of a mouse brain. But Lichtman is not daunted. He is determined to map whole brains, exorbitant exabyte-scale storage be damned.
Lichtman’s office is a spacious place with floor-to-ceiling windows overlooking a tree-lined walkway and an old circular building that, in the days before neuroscience even existed as a field, used to house a cyclotron. He was wearing a deeply black sweater, which contrasted with his silver hair and olive skin. When I asked if a completed connectome would give us a full understanding of the brain, he didn’t pause in his answer. I got the feeling he had thought a great deal about this question on his own.
“I think the word ‘understanding’ has to undergo an evolution,” Lichtman said, as we sat around his desk. “Most of us know what we mean when we say ‘I understand something.’ It makes sense to us. We can hold the idea in our heads. We can explain it with language. But if I asked, ‘Do you understand New York City?’ you would probably respond, ‘What do you mean?’ There’s all this complexity. If you can’t understand New York City, it’s not because you can’t get access to the data. It’s just there’s so much going on at the same time. That’s what a human brain is. It’s millions of things happening simultaneously among different types of cells, neuromodulators, genetic components, things from the outside. There’s no point when you can suddenly say, ‘I now understand the brain,’ just as you wouldn’t say, ‘I now get New York City.’ ”
We have this false belief there’s nothing that humans can’t understand because we have infinite intelligence.
“But we understand specific aspects of the brain,” I said. “Couldn’t we put those aspects together and get a more holistic understanding?”
“I guess I would retreat to another beachhead, which is, ‘Can we describe the brain?’ ” Lichtman said. “There are all sorts of fundamental questions about the physical nature of the brain we don’t know. But we can learn to describe them. A lot of people think ‘description’ is a pejorative in science. But that’s what the Hubble telescope does. That’s what genomics does. They describe what’s actually there. Then from that you can generate your hypotheses.”
“Why is description an unsexy concept for neuroscientists?”
“Biologists are often seduced by ideas that resonate with them,” Lichtman said. That is, they try to bend the world to their idea rather than the other way around. “It’s much better—easier, actually—to start with what the world is, and then make your idea conform to it,” he said. Instead of a hypothesis-testing approach, we might be better served by following a descriptive, or hypothesis-generating methodology. Otherwise we end up chasing our own tails. “In this age, the wealth of information is an enemy to the simple idea of understanding,” Lichtman said.
“How so?” I asked.
“Let me put it this way,” Lichtman said. “Language itself is a fundamentally linear process, where one idea leads to the next. But if the thing you’re trying to describe has a million things happening simultaneously, language is not the right tool. It’s like understanding the stock market. The best way to make money on the stock market is probably not by understanding the fundamental concepts of economy. It’s by understanding how to utilize this data to know what to buy and when to buy it. That may have nothing to do with economics but with data and how data is used.”
“Maybe human brains aren’t equipped to understand themselves,” I offered.
“And maybe there’s something fundamental about that idea: that no machine can have an output more sophisticated than itself,” Lichtman said. “What a car does is trivial compared to its engineering. What a human brain does is trivial compared to its engineering. Which is the great irony here. We have this false belief there’s nothing in the universe that humans can’t understand because we have infinite intelligence. But if I asked you if your dog can understand something you’d say, ‘Well, my dog’s brain is small.’ Well, your brain is only a little bigger,” he continued, chuckling. “Why, suddenly, are you able to understand everything?”
Was Lichtman daunted by what a connectome might achieve? Did he see his efforts as Sisyphean?
“It’s just the opposite,” he said. “I thought at this point we would be less far along. Right now, we’re working on a cortical slab of a human brain, where every synapse is identified automatically, every connection of every nerve cell is identifiable. It’s amazing. To say I understand it would be ridiculous. But it’s an extraordinary piece of data. And it’s beautiful. From a technical standpoint, you really can see how the cells are connected together. I didn’t think that was possible.”
Lichtman stressed his work was about more than a comprehensive picture of the brain. “If you want to know the relationship between neurons and behavior, you gotta have the wiring diagram,” he said. “The same is true for pathology. There are many incurable diseases, such as schizophrenia, that don’t have a biomarker related to the brain. They’re probably related to brain wiring but we don’t know what’s wrong. We don’t have a medical model of them. We have no pathology. So in addition to fundamental questions about how the brain works and consciousness, we can answer questions like, Where did mental disorders come from? What’s wrong with these people? Why are their brains working so differently? Those are perhaps the most important questions to human beings.”
Late one night, after a long day of trying to make sense of my data, I came across a short story by Jorge Louis Borges that seemed to capture the essence of the brain mapping problem. In the story, “On Exactitude in Science,” a man named Suarez Miranda wrote of an ancient empire that, through the use of science, had perfected the art of map-making. While early maps were nothing but crude caricatures of the territories they aimed to represent, new maps grew larger and larger, filling in ever more details with each edition. Over time, Borges wrote, “the Art of Cartography attained such Perfection that the map of a single Province occupied the entirety of a City, and the map of the Empire, the entirety of a Province.” Still, the people craved more detail. “In time, those Unconscionable Maps no longer satisfied, and the Cartographers Guilds struck a Map of the Empire whose size was that of the Empire, and which coincided point for point with it.”
The Borges story reminded me of Lichtman’s view that the brain may be too complex to be understood by humans in the colloquial sense, and that describing it may be a better goal. Still, the idea made me uncomfortable. Much like storytelling, or even information processing in the brain, descriptions must leave some details out. For a description to convey relevant information, the describer has to know which details are important and which are not. Knowing which details are irrelevant requires having some understanding about the thing you’re describing. Will my brain, as intricate as it may be, ever be able to make sense of the two exabytes in a mouse brain?
The Borges story reminded me of the view that the brain may be too complex to be understood by humans.
Humans have a critical weapon in this fight. Machine learning has been a boon to brain mapping, and the self-reinforcing relationship promises to transform the whole endeavor. Deep learning algorithms (also known as deep neural networks, or DNNs) have in the past decade allowed machines to perform cognitive tasks once thought impossible for computers—not only object recognition, but text transcription and translation, or playing games like Go or chess. DNNs are mathematical models that string together chains of simple functions that approximate real neurons. These algorithms were inspired directly by the physiology and anatomy of the mammalian cortex, but are crude approximations of real brains, based on data gathered in the 1960s. Yet they have surpassed expectations of what machines can do.
The secret to Lichtman’s progress with mapping the human brain is machine intelligence. Lichtman’s team, in collaboration with Google, is using deep networks to annotate the millions of images from brain slices their microscopes collect. Each scan from an electron microscope is just a set of pixels. Human eyes easily recognize the boundaries of each blob in the image (a neuron’s soma, axon, or dendrite, in addition to everything else in the brain), and with some effort can tell where a particular bit from one slice appears on the next slice. This kind of labeling and reconstruction is necessary to make sense of the vast datasets in connectomics, and have traditionally required armies of undergraduate students or citizen scientists to manually annotate all chunks. DNNs trained on image recognition are now doing the heavy lifting automatically, turning a job that took months or years into one that’s complete in a matter of hours or days. Recently, Google identified each neuron, axon, dendrite, and dendritic spike—and every synapse—in slices of the human cerebral cortex. “It’s unbelievable,” Lichtman said.
Scientists still need to understand the relationship between those minute anatomical features and dynamical activity profiles of neurons—the patterns of electrical activity they generate—something the connectome data lacks. This is a point on which connectomics has received considerable criticism, mainly by way of example from the worm: Neuroscientists have had the complete wiring diagram of the worm C. elegans for a few decades now, but arguably do not understand the 300-neuron creature in its entirety; how its brain connections relate to its behaviors is still an active area of research.
Still, structure and function go hand-in-hand in biology, so it’s reasonable to expect one day neuroscientists will know how specific neuronal morphologies contribute to activity profiles. It wouldn’t be a stretch to imagine a mapped brain could be kickstarted into action on a massive server somewhere, creating a simulation of something resembling a human mind. The next leap constitutes the dystopias in which we achieve immortality by preserving our minds digitally, or machines use our brain wiring to make super-intelligent machines that wipe humanity out. Lichtman didn’t entertain the far-out ideas in science fiction, but acknowledged that a network that would have the same wiring diagram as a human brain would be scary. “We wouldn’t understand how it was working any more than we understand how deep learning works,” he said. “Now, suddenly, we have machines that don’t need us anymore.”
Yet a masterly deep neural network still doesn’t grant us a holistic understanding of the human brain. That point was driven home to me last year at a Computational and Systems Neuroscience conference, a meeting of the who’s-who in neuroscience, which took place outside Lisbon, Portugal. In a hotel ballroom, I listened to a talk by Arash Afraz, a 40-something neuroscientist at the National Institute of Mental Health in Bethesda, Maryland. The model neurons in DNNs are to real neurons what stick figures are to people, and the way they’re connected is equally as sketchy, he suggested.
Afraz is short, with a dark horseshoe mustache and balding dome covered partially by a thin ponytail, reminiscent of Matthew McConaughey in True Detective. As sturdy Atlantic waves crashed into the docks below, Afraz asked the audience if we remembered René Magritte’s “Ceci n’est pas une pipe” painting, which depicts a pipe with the title written out below it. Afraz pointed out that the model neurons in DNNs are not real neurons, and the connections among them are not real either. He displayed a classic diagram of interconnections among brain areas found through experimental work in monkeys—a jumble of boxes with names like V1, V2, LIP, MT, HC, each a different color, and black lines connecting the boxes seemingly at random and in more combinations than seems possible. In contrast to the dizzying heap of connections in real brains, DNNs typically connect different brain areas in a simple chain, from one “layer” to the next. Try explaining that to a rigorous anatomist, Afraz said, as he flashed a meme of a shocked baby orangutan cum anatomist. “I’ve tried, believe me,” he said.
A network with the same diagram as the human brain would be scary. We’d have machines that don’t need us anymore.
I, too, have been curious why DNNs are so simple compared to real brains. Couldn’t we improve their performance simply by making them more faithful to the architecture of a real brain? To get a better sense for this, I called Andrew Saxe, a computational neuroscientist at Oxford University. Saxe agreed that it might be informative to make our models truer to reality. “This is always the challenge in the brain sciences: We just don’t know what the important level of detail is,” he told me over Skype.
How do we make these decisions? “These judgments are often based on intuition, and our intuitions can vary wildly,” Saxe said. “A strong intuition among many neuroscientists is that individual neurons are exquisitely complicated: They have all of these back-propagating action potentials, they have dendritic compartments that are independent, they have all these different channels there. And so a single neuron might even itself be a network. To caricature that as a rectified linear unit”—the simple mathematical model of a neuron in DNNs—“is clearly missing out on so much.”
As 2020 has arrived, I have thought a lot about what I have learned from Lichtman, Afraz, and Saxe and the holy grail of neuroscience: understanding the brain. I have found myself revisiting my undergrad days, when I held science up as the only method of knowing that was truly objective (I also used to think scientists would be hyper-rational, fair beings paramountly interested in the truth—so perhaps this just shows how naive I was).
It’s clear to me now that while science deals with facts, a crucial part of this noble endeavor is making sense of the facts. The truth is screened through an interpretive lens even before experiments start. Humans, with all our quirks and biases, choose what experiment to conduct in the first place, and how to do it. And the interpretation continues after data are collected, when scientists have to figure out what the data mean. So, yes, science gathers facts about the world, but it is humans who describe it and try to understand it. All these processes require filtering the raw data through a personal sieve, sculpted by the language and culture of our times.
It seems likely that Lichtman’s two exabytes of brain slices, and even my 48 terabytes of rat brain data, will not fit through any individual human mind. Or at least no human mind is going to orchestrate all this data into a panoramic picture of how the human brain works. As I sat at my office desk, watching the setting sun tint the cloudless sky a light crimson, my mind reached a chromatic, if mechanical, future. The machines we have built—the ones architected after cortical anatomy—fall short of capturing the nature of the human brain. But they have no trouble finding patterns in large datasets. Maybe one day, as they grow stronger building on more cortical anatomy, they will be able to explain those patterns back to us, solving the puzzle of the brain’s interconnections, creating a picture we understand. Out my window, the sparrows were chirping excitedly, not ready to call it a day.
Grigori Guitchounts is a neuroscientist. You can read a bit about his 48 terabytes of rat brain data here.
Lead image: A rendering of dendrites (red)—a neuron’s branching processes—and protruding spines that receive synaptic information, along with a saturated reconstruction (multicolored cylinder) from a mouse cortex. Courtesy of Lichtman Lab at Harvard University.
This article first appeared online in our “Maps” issue in January, 2020.