In 2009, researchers working in Thailand made headlines with a small success in a trial of an HIV vaccine. It reduced the rate of infection by 31 percent, the scientists calculated. That may not sound impressive, but in the fight against HIV, it looked like an unprecedented success. The researchers published their results in the influential New England Journal of Medicine, reporting that the data had passed standard statistical tests: If the vaccine had actually been worthless, there was only a 1 in 25 chance that it would have appeared to have the beneficial effect seen in the study.
In medicine, as in most other realms of science, observing low-probability data like that in the HIV study is cause for celebration. Typically, scientists in fields like biology, psychology, and other social sciences rejoice when the chance of a fluke is less than 1 in 20. In some fields, however, such as particle physics, researchers are satisfied only with much lower probabilities, on the order of one chance in 3.5 million. But whatever the threshold, recording low-probability data—data unlikely to be seen if nothing is there to be discovered—is what entitles you to conclude that you’ve made a discovery. Observing low-probability events is at the heart of the scientific method for testing hypotheses.
Scientists use elaborate statistical significance tests to distinguish a fluke from real evidence. But the sad truth is that the standard methods for significance testing are often inadequate to the task. In the case of the HIV vaccine, for instance, further analysis showed the findings not to be as solid as the original statistics suggested. Chances were probably 20 percent or higher that the vaccine was not effective at all.
Thoughtful experts have been pointing out serious flaws in standard statistical methods for decades. In recent years, the depth of the problem has become more apparent and more well-documented. One recent paper found an appallingly low chance that certain neuroscience studies could correctly identify an effect from statistical data. Reviews of genetics research show that the statistics linking diseases to genes are wrong far more often than they’re right. Pharmaceutical companies find that test results favoring new drugs typically disappear when the tests are repeated.
Reviews of genetics research show that the statistics linking diseases to genes are wrong far more often than they’re right.
In fact, in almost all research fields, studies often draw erroneous conclusions. Sometimes the errors arise because statistical tests are misused, misinterpreted, or misunderstood. And sometimes sloppiness, outright incompetence, or possibly fraud is to blame. But even research conducted strictly by the book frequently fails because of faulty statistical methods that have been embedded in the scientific process.
“There is increasing concern that in modern research, false findings may be the majority or even the vast majority of published research claims,” epidemiologist John P.A. Ioannidis declared in a landmark essay published in 2005 in the journal PLoS Medicine.
Even when a claimed effect does turn out to be correct, its magnitude is usually overstated. Columbia University political scientist and statistician Andrew Gelman puts it bluntly: “The scientific method that we love so much is a machine for generating exaggerations.”
Not all science is wrong, of course. When studies are replicated and evidence accumulates from different lines of investigation, scientific research converges on reliable knowledge about nature. But any individual research finding stands a high probability of being bogus. “Methodologists have attempted to draw our attention to the foibles of significance tests for generations,” Intel’s Charles Lambdin wrote last year in Theory & Psychology. “And yet the fad persists.”
Far from merely a technical concern, this issue is literally a matter of life and death. Misuse of statistics generates controversies about the safety of medicines that end up depriving some people of life-saving treatments. And media coverage of such issues—and scientific results in general—is confounded by the diabolical coincidence that headline-grabbing scientific studies are the very ones that are most susceptible to being statistical illusions. And so the common complaint that scientists always change their minds, as one media report is later contradicted by another, has its roots in the math used to analyze the probability of experimental data.
That math traces its origins back to a famous series of letters between mathematicians Blaise Pascal and Pierre de Fermat in the 17th century. Their interest was gambling, and their insights eventually led to modern probability theory. The financial success of today’s casino industry testifies to probability theory’s reliability.
But applying probability theory to testing hypotheses isn’t so simple. Scientists have been struggling with it for almost a century now. Today’s methods were born in the 1920s, when the mathematician Ronald Fisher devised the experimental method called null hypothesis testing. Fisher worked for a British agricultural research station, and he wanted to see whether fertilizing a field produced a higher crop yield. Since yields differ from field to field anyway, for a number of reasons, he wanted to know how big a difference you’d need to see to conclude that fertilizer has a real effect. He showed how to calculate the probability of fertilized fields producing a yield different from that of unfertilized fields for reasons other than the fertilizer itself—the “null” hypothesis. He called that probability the P value. If P is less than 0.05—a 5 percent chance of seeing the observed (or greater) difference even if the factor being studied had no effect—it should be considered “statistically significant,” Fisher said. And you could feel good about recommending fertilizer.
The common complaint that scientists always change their minds, as one media report is later contradicted by another, has its roots in the math used to analyze the probability of experimental data.
Fisher’s approach became very influential, but it didn’t satisfy everybody. Others proposed similar methods but with different interpretations for the P value. Fisher said a low P value merely means that you should reject the null hypothesis; it does not actually tell you how likely the null hypothesis is to be correct. Others interpreted the P value as the likelihood of a false positive: concluding an effect is real when it actually isn’t. Textbook writers merged the contradictory interpretations into a hybrid prescription that psychologist Gerd Gigerenzer calls the “null ritual,” a mindless process for producing data that researchers seldom interpret correctly. Psychology adopted the null ritual early on, and then it spread (like a disease) to many other fields, including biology, economics, and ecology. “People do this in a ritualistic way,” Gigerenzer says. “It’s like compulsive hand washing.”
But while widely used, the ritual really does not work very well, as some astute observers warned early on. “Despite the awesome preeminence this method has attained in our experimental journals and textbooks of applied statistics, it is based upon a fundamental misunderstanding of the nature of rational inference and is seldom if ever appropriate to the aims of scientific research,” philosopher of science William Rozeboom wrote—in 1960.
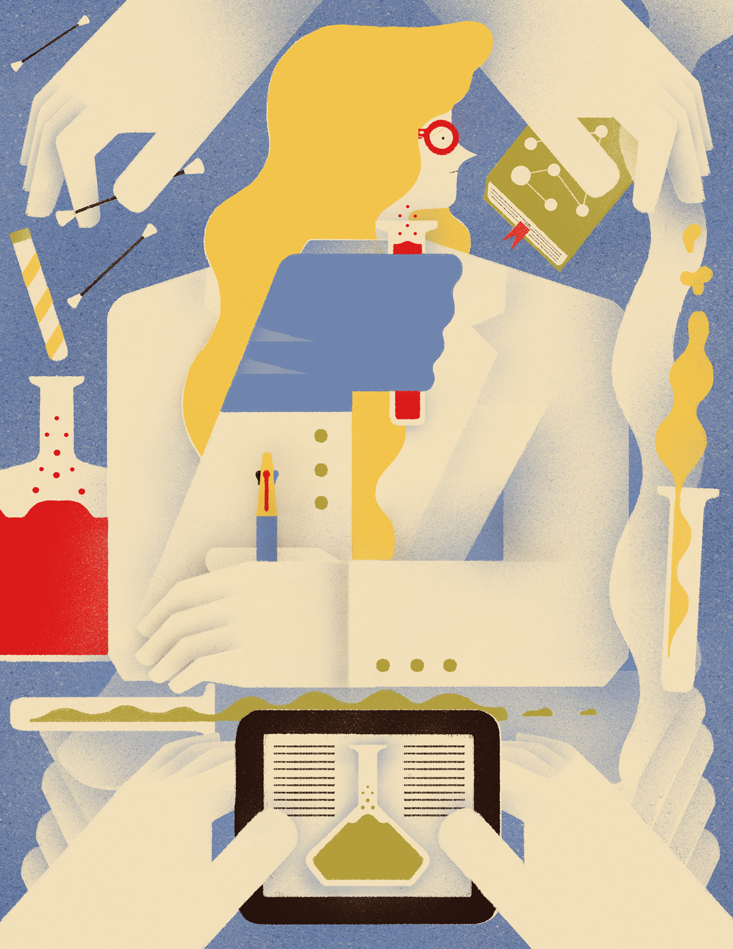
At the heart of the problem is the simple mathematical hitch that a P value really doesn’t mean much. It’s just a measure of how unlikely your result is if there is no real effect. “It doesn’t tell you anything about whether the null hypothesis is true,” Gigerenzer points out. A low P value might mean that fertilizer works, or it might just mean that you witnessed the one time out of 20 that a crop yield was unusually big.
It’s like flipping coins. Sometimes you’ll flip a penny and get several heads in a row, but that doesn’t mean the penny is rigged. Suppose, for instance, that you toss a penny 10 times. A perfectly fair coin (heads or tails equally likely) will often produce more or fewer than five heads. In fact, you’ll get exactly five heads only about a fourth of the time. Sometimes you’ll get six heads, or four. Or seven, or eight. In fact, even with a fair coin, you might get 10 heads out of 10 flips (but only about once for every thousand 10-flip trials).
So how many heads should make you suspicious? Suppose you get eight heads out of 10 tosses. For a fair coin, the chances of eight or more heads are only about 5.5 percent. That’s a P value of 0.055, close to the standard statistical significance threshold. Perhaps suspicion is warranted.
But the truth is, all you know is that it’s unusual to get eight heads out of 10 flips. The penny might be weighted to favor heads, or it might just be one of those 55 times out of a thousand that eight or more heads show up. There’s no logic in concluding anything at all about the penny.
Ah, some scientists would say, maybe you can’t conclude anything with certainty. But with only a 5 percent chance of observing the data if there’s no effect, there’s a 95 percent chance of an effect—you can be 95 percent confident that your result is real. The problem is, that reasoning is 100 percent incorrect. For one thing, the 5 percent chance of a fluke is calculated by assuming there is no effect. If there actually is an effect, the calculation is no longer valid. Besides that, such a conclusion exemplifies a logical fallacy called “transposing the conditional.” As one statistician put it, “it’s the difference between I own the house or the house owns me.”
For a simple example, suppose that each winter, I go swimming only three days—less than 5 percent of the time. In other words, there is less than a 5 percent chance of my swimming on any given day in the winter, corresponding to a P value of less than 0.05. So if you observe me swimming, is it therefore a good bet (with 95 percent confidence) that it’s not winter? No! Perhaps the only time I ever go swimming is while on vacation in Hawaii for three days every January. Then there’s less than a 5 percent chance of observing me swim in the winter, but a 100 percent chance that it is winter if you see me swimming.
That’s a contrived example, of course, but it does expose a real flaw in the standard statistical methods. Studies have repeatedly shown that scientific conclusions based on calculating P values are indeed frequently false.
In one study, researchers collected papers finding statistically significant links of 85 genetic variants to the risk of a disorder called acute coronary syndrome. But when the researchers tested the genes of 811 patients diagnosed with it, only one of the 85 variants actually appeared substantially more often than in a matched group of healthy people. And that could easily have been a fluke. “Our null results provide no support for the hypothesis that any of the 85 genetic variants tested is a susceptibility factor,” Thomas Morgan and collaborators wrote.
But with only a 5 percent chance of observing the data if there’s no effect, there’s a 95 percent chance of an effect—you can be 95 percent confident that your result is real. The problem is, that reasoning is 100 percent incorrect.
More recently, pharmaceutical companies have noted that standard methods for identifying possible drug targets produce results that frequently can’t be replicated. Bayer found that two-thirds of such findings couldn’t be reproduced. And Amgen scientists, following up on 53 studies that at first glance looked worth pursuing, could confirm only six of them.
Just as statistical significance does not mean an effect is real, lack of statistical significance does not mean there is no effect. So many studies miss real connections, especially when the sample size is small.
When an effect is slight, even though real, small studies lack what statisticians call statistical power. If a low risk (say, a 2 percent chance of a heart attack) is doubled by the use of some new medicine, for instance, testing only a few hundred people will not be powerful enough to find the effect. A real doubling of a 2 percent effect in a group that small would not be recognized as statistically significant.
Small samples are a big problem in fields like neuroscience, where one recent study found an average statistical power of about 20 percent, which means only one study in five is able to detect a real effect. “Low statistical power is an endemic problem in neuroscience,” Katherine Button and collaborators wrote in the May issue of Nature Reviews Neuroscience.
Ironically, low statistical power doesn’t only mean real effects can be missed. It also means false effects are more likely to be reported as real, or real effects exaggerated. It’s hard to reach statistical significance with a small sample, so only outlier results are likely to reach the threshold. This problem is known as the winner’s curse—the first scientist to “discover” an effect often records an exaggerated result. Subsequent studies will typically find a lower effect, or no effect at all.
On the other hand, really big trials also pose problems. With a huge sample, even a tiny difference, not significant in a practical sense, can be statistically significant. And some large studies investigate many possible associations at once, so some will appear to be true just by chance. This many-hypothesis problem is especially acute in genetics, where the activity of more than 20,000 genes can be tested simultaneously. The idea is to find which genes are more (or less) active than normal in people with a particular disease. But if your statistical significance threshold is 0.05—1 in 20—then the study could list roughly 1,000 genes as more or less active than usual, even if none actually is. Raising the bar for statistical significance will eliminate some flukes, but only at the cost of also eliminating some truly changed genes. Methods have been devised to ameliorate this problem, but it still afflicts many types of research. Of course, even when only one hypothesis is tested at a time, the scope of the scientific enterprise is so huge that many statistically significant findings will turn out to be flukes. Thousands of scientific papers are published every week; a 1 in 20 threshold for significance guarantees that numerous false claims will be made daily.
That’s why good scientists stress the need for repeating experiments to confirm an initial finding. Any given finding might be wrong, but if the same result is found in subsequent studies, the confidence in its validity rapidly grows. If you toss a penny and get eight heads out of 10 once, you can’t conclude anything. But if you get eight or nine heads in the next trial, and the next after that, you can be pretty sure that the coin is biased. In particle physics, researchers must clear a much higher bar before declaring a discovery, such as the Higgs boson last year, and replication is still essential. Even with only a 1 in 6 million chance of a fluke, few experts would believe the result if not for the fact that two independent experiments both found similar strong evidence.
But often in science, studies are too difficult or expensive to repeat, or nobody wants to bother—sometimes because a lot of money or prestige is on the line. When a study finds nothing interesting, researchers might not even attempt to publish it—or they might not be able to get it published if they tried. Scientific journals themselves are often eager to publish only “new” results, rendering many replications or “no effect” findings tucked away in file drawers or on hard drives.
Low statistical power doesn’t only mean real effects can be missed. It also means false effects are more likely to be reported as real, or real effects exaggerated.
All these factors conspire to give positive findings, often likely to be flukes, more attention than they deserve—especially in the media. It’s not exactly a news bulletin that the media often get science wrong. But even when a journalist faithfully presents a scientific paper’s conclusion just as the scientists do, odds still are that it’s wrong—and not just because most scientific papers may be wrong to begin with. It’s because the qualities of a scientific paper that make it newsworthy are precisely those that make it even more likely to be a statistical fluke.
For one thing, journalists are eager to report the first instance of a finding, just as scientists are. But first reports suffer from the winner’s curse—possibly being wrong, or overstating the magnitude of an effect, if there is one. Even without curses, first reports are likely to be wrong in many cases. Suppose one lab tests an arsenal of 100 candidate drugs to find one that reduces symptoms by a statistically significant amount. Say only one of the candidates actually works. For a P value threshold of 0.05, five additional drugs will appear to work just by chance. So, in this simplified example, the odds that the first report of an effective drug is right are just 1 in 6. The first report will most likely be one of the flukes. And that’s the report that will make the news.
Reporters sometimes do write about subsequent papers in popular research fields, like cancer research or cloning. Hot topics make news, but also magnify errors. “The hotter a scientific field (with more scientific teams involved), the less likely the research findings are to be true,” Ioannidis wrote in his 2005 paper. In these competitive fields, numerous labs around the world pursue the same goal. Say, in the course of a year, 50 published papers reported statistically significant results. But another 950 experiments didn’t find anything worth publishing. Those 50 published papers represent the 5 percent of the time that fluke data would appear significant. News reports on those stories might give the impression that there are lots of important findings when there’s really no good reason to believe any of them.
Another sure way to entice a reporter is a press release that starts out by saying, “Contrary to previous scientific belief…” Here again, these are precisely the results that are least likely to stand up to scrutiny. Presumably (although of course, not always), previous scientific belief is based on previous scientific data. If new data doesn’t correspond to a lot of old data, it’s most likely that the new result is the statistical outlier. There is usually no reason to believe that one new study is right and all previous studies are wrong (unless the new data come from improved methods or a technologically advanced instrument). Ordinarily, “contrary to previous belief” should be a warning flag that the result being reported is likely to be wrong. Instead it is usually a green light to go with the story. So the general criteria of newsworthiness—a first report, in a hot field, producing findings contrary to previous belief—seem designed specifically to select the scientific papers most likely to be bogus.
And there’s one other type of paper that attracts journalists while illustrating the wider point: research about smart animals. One such study involved a fish—an Atlantic salmon—placed in a brain scanner and shown various pictures of human activity. One particular spot in the fish’s brain showed a statistically significant increase in activity when the pictures depicted emotional scenes, like the exasperation on the face of a waiter who had just dropped his dishes.
The scientists didn’t rush to publish their finding about how empathetic salmon are, though. They were just doing the test to reveal the quirks of statistical significance. The fish in the scanner was dead.
Tom Siegfried is a freelance writer in northern Virginia and former editor in chief of Science News.