One question for Laura Weidinger and Iason Gabriel, research scientists at Google DeepMind, a London-based AI company, where they both focus on the ethical, political, and social implications of AI.
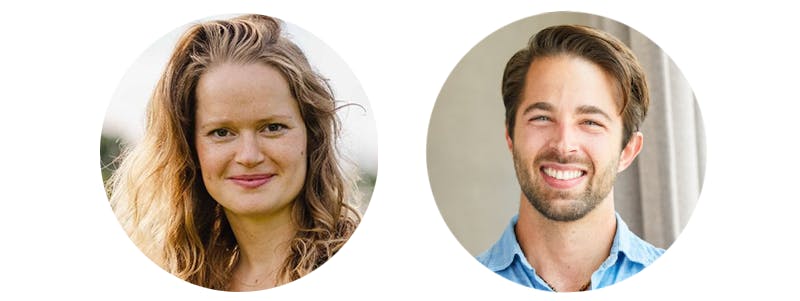
Who should make the rules that govern AI?
We think that everyone who will be affected by these technologies should have a say on what principles govern them. This presents a practical challenge—how do we gather these people’s views, from across the globe? And how do we do this repeatedly, given preferences and views on AI are likely to change over time? We think that gathering these views in a fair, inclusive, and participatory way is a key unresolved issue for building just or fair AI systems. And we try to resolve that issue in our new paper.
We assembled a team of philosophers, psychologists, and AI researchers to gather empirical evidence that complements debates on what mechanisms should be used to identify principles that govern AI. We focus on a proof of concept for a process that lets us gather people’s considered views on these issues. To do that, we ran five studies with a total of 2,508 participants and tested a thought experiment from political philosophy that is meant to elicit principles of justice—meaning principles that lead to fair outcomes and should be acceptable to everyone if they deliberate in an unbiased way.
How can fair principles to govern AI be identified?
This thought experiment is known as the Veil of Ignorance, developed by the political philosopher John Rawls. It is meant to help surface fair principles to govern society. We extended this test to see how it may work when it comes to identifying rules to govern AI. The idea in this thought experiment is that when people choose rules or laws without knowing how those rules would affect them, they cannot choose them in a way that favors their own particular interest. Since nobody can unduly favor themselves, anyone who makes a decision from behind the veil should choose principles that are fair to everyone. We implement this thought experiment in a few different ways where people choose principles to govern AI.
Our study suggests that when people don’t know their own social position—whether they’re advantaged or disadvantaged—they are more likely to pick principles to govern the AI that favor those who are worst off. This mirrors Rawls’ speculation—he thought that people reasoning behind the veil would choose to prioritize those who are worst off in a society.
Our study was inspired by the observation that without a purposeful direction, AI systems are likely to disproportionately benefit those who are already well-off. We also noticed that debates on “AI alignment” were in need of fresh perspectives and novel approaches—ones that directly addressed divergences of belief and opinion among different groups and individuals. So we decided to try a new, interdisciplinary approach—deploying methods from experimental philosophy—to explore the research question of “how can fair principles to govern AI be identified?”
While this is only a proof of concept and a study we ran with a relatively small number of online participants in the United Kingdom, it is encouraging to see that when people reason about AI from behind the veil, they think about what is fair. Moreover, they most often want the AI to follow a rule that prioritizes the worst off, and not a rule that maximizes overall utility if this benefits those who are already well-off. This links to a feminist point of view—that centering and supporting those who are most disadvantaged and marginalized is fair and ultimately better for society at large.
We’re not suggesting that experiments like ours are the way to determine principles that govern AI on their own—democratically legitimate avenues are required for that. However, we do think that we have identified principles that are endorsed by people who reason in a more impartial way, thinking about fairness. So when we think about what principles to build into AI from the beginning in order to build systems that are just, prioritizing the worst-off is a good starting point.
Lead image: icedmocha / Shutterstock