Here are three versions of the same story:
1. In the fall of 1996, Sally Clark, an English solicitor in Manchester, gave birth to an apparently healthy baby boy who died suddenly when he was 11 weeks old. She was still recovering from the traumatic incident when she had another baby boy the following year. Tragically, he also died, eight weeks after being born. The causes of the two children’s deaths were not readily apparent, but the police suspected they were no coincidence. Clark was arrested and charged with two counts of murder. The pediatrician Roy Meadow, inventor of the term “Munchausen Syndrome by Proxy,” testified at the trial that it was extremely unlikely that two children from an affluent family like the Clarks would die from Sudden Infant Death Syndrome (SIDS) or “cot death.” He estimated the odds were 1 in 73 million, which he colorfully compared to an 80:1 longshot winning the Grand National horse race four years in a row. Clark was convicted and sentenced to life in prison. The press reviled her as a child murderer.
2. Suppose an otherwise healthy woman in her forties notices a suspicious lump in her breast and goes in for a mammogram. The report comes back that the lump is malignant. She wants to know the chance of the diagnosis being wrong. Her doctor answers that, as diagnostic tools go, these scans are very accurate. Such a scan would find nearly 100 percent of true cancers and would only misidentify a benign lump as cancer about 5 percent of the time. Therefore, the probability of this being a false positive is very low, about 1 in 20.
3. In 2012, Professor Ara Norenzayan at the University of British Columbia claimed to have evidence that looking at an image of Rodin’s sculpture “The Thinker” could make people less religious. In a trial of 57 college students, he randomly assigned participants to either view “The Thinker” or a control image, Myron’s Discobolus, a sculpture of a Greek athlete throwing a discus, and then rate their belief in God on a scale from 1 to 100. Subjects who had been exposed to “The Thinker” reported a significantly lower mean God-belief score of 41.42 vs. the control group’s 61.55. The probability of observing a difference at least this large by chance alone was about 3 percent. So he and his coauthor concluded “The Thinker” had prompted their participants to think analytically and that “a novel visual prime that triggers analytic thinking also encouraged disbelief in God.”
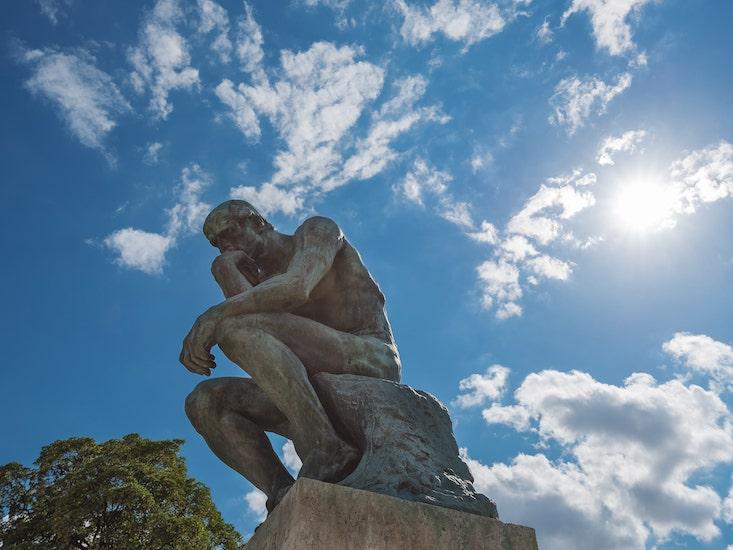
All three of these vignettes involve the same error in reasoning with probabilities. The first two are examples of well-known fallacies, called, respectively, the Prosecutor’s Fallacy and the Base Rate Fallacy. The third is a typical statistical analysis of a scientific study, of the kind you can find in most any reputable journal today. In fact, Norenzayan’s results were published in Science and have to date been cited some 424 times in research literature. Atheists hailed it as scientific proof that religion was irrational; religious people were understandably offended at the suggestion that the source of their faith was a lack of reasoning ability.
The failure in reasoning at the heart of the three examples points to why so many results, in fields from astronomy to zoology, cannot be replicated, a big problem that the world of science is currently turning itself inside out trying to deal with.
The mathematical lens that allows us to see the flaw in these arguments is Bayes’ theorem. The theorem dictates that the probability we assign to a theory (Sally Clark is guilty, a patient has cancer, college students become less theistic when they stare at Rodin), in light of some observation, is proportional both to the conditional probability of the observation assuming the theory is true, and to the prior probability we gave the theory before making the observation. When two theories compete, one may make the observation much more probable, that is, produce a higher conditional probability. But according to Bayes’ rule, we might still consider that explanation unlikely if we gave it a low probability of being true from the start.
So, the missing ingredient in all three examples is the prior probability for the various hypotheses. In the case of Sally Clark, the prosecution’s theory was she had murdered her children, itself an extremely rare event. Suppose, for argument’s sake, by tallying up historical murder records, we arrived at prior odds of 100 million to 1 for any particular mother like her to commit double infanticide. That would have balanced the extreme unlikelihood of the observation (two infants dying) under the alternative hypothesis that they were well cared for. Numerically, Bayes’ theorem would tell us to compare:
(1/73,000,000) * (99,999,999/100,000,000) vs. (1) * (1/100,000,000)
We’d conclude, based on these priors and no additional evidence aside from the children’s deaths, that it was actually about 58 percent likely Clark was innocent.
The mathematical lens that allows us to see the flaw in these arguments is Bayes’ theorem.
For the breast cancer example, the doctor would need to consider the overall incidence rate of cancer among similar women with similar symptoms, not including the result of the mammogram. Maybe a physician would say from experience that about 99 percent of the time a similar patient finds a lump it turns out to be benign. So the low prior chance of a malignant tumor would balance the low chance of getting a false positive scan result. Here we would weigh the numbers:
(0.05) * (0.99) vs. (1) * (0.01)
We’d find there was about an 83 percent chance the patient doesn’t have cancer.
Regarding the study of sculpture and religious sentiment, we need to assess the likelihood, before considering the data, that a brief encounter with art could have such an effect. Past experience should make us pretty skeptical, especially given the size of the claimed effect, about a 33 percent reduction in average belief in God. If art could have such an influence, we’d find any trip to a museum would send us careening between belief and non-belief. Or if somehow “The Thinker” wielded a unique atheistic power, its unveiling in Paris in 1904 should have corresponded with a mass exodus from organized religion. Instead, we experience our own religious beliefs, and those of our society, as relatively stable through time. Maybe we’re not so dogmatic as to rule out “The Thinker” hypothesis altogether, but a prior probability of 1 in 1,000, somewhere between the chance of being dealt a full house and four-of-a-kind in a poker hand, could be around the right order of magnitude.
Norenzayan’s data, which he claimed was unlikely to have arisen by chance, would need to be that much more unlikely to shake us of our skepticism. According to the study, the results were about 12 times more probable under an assumption of an effect of the observed magnitude than they would have been under an assumption of pure chance. Putting this claim into Bayes’ theorem with our prior probability assignment would yield:
(12 p) * (1/1,000) vs. (p) * (999/1,000)
We’d end up saying the probability for “The Thinker”-atheism effect based on this experiment was 0.012, or about 1 in 83, a mildly interesting blip but almost certainly not worth publishing.
The problem, though, is the dominant mode of statistical analysis these days isn’t Bayesian. Since the 1920s, the standard approach to judging scientific theories has been significance testing, made popular by the statistician Ronald Fisher. Fisher’s methods and their latter-day spinoffs are now the lingua franca of scientific data analysis. In particular, Google Scholar currently returns 2.85 million citations including the phrase “statistically significant.” Fisher claimed signficance testing was a universal tool for scientific inference, “common to all experimentation,” a claim that seems borne out by its widespread use across all disciplines.
Fisher hated Bayesian inference with a passion and considered it a great historical error, “the only mistake to which the mathematical world has so deeply committed itself.” As a result, his methods don’t have any place for prior probabilities, which he argued weren’t necessary to make inferences. Significance testing only uses the probability of the data assuming a hypothesis is true, that is, only the conditional probability part of Bayes’ rule. If the observed data (or more extreme data) would be very unlikely under a hypothesis, usually the “null hypothesis” of no effect, the data is deemed “significant” and considered sufficient evidence to reject the hypothesis.
Atheists hailed it as scientific proof that religion was irrational; religious people were understandably offended.
Defending the logic of this approach, Fisher wrote, “A man who ‘rejects’ a hypothesis provisionally, as a matter of habitual practice, when the significance is at the 1 percent level or higher”—that is, when data this extreme could only be expected 1 percent of the time—“will certainly be mistaken in not more than 1 percent of such decisions. For when the hypothesis is correct he will be mistaken in just 1 percent of these cases, and when it is incorrect he will never be mistaken in rejection.”
However, that argument obscures a key point. To understand what’s wrong, consider the following completely true, Fisherian summary of the facts in the breast cancer example (no false negatives, 5 percent false positive rate):
Suppose we scan 1 million similar women, and we tell everyone who tests positive that they have cancer. Then, among those who actually have cancer, we will be correct every single time. And among those who don’t have it, we will be only be incorrect 5 percent of the time. So, overall our procedure will be incorrect less than 5 percent of the time.
Sounds persuasive, right? But here’s another summary of the facts, including the base rate of 1 percent:
Suppose we scan 1 million similar women, and we tell everyone who tests positive that they have cancer. Then we will have correctly told all 10,000 women with cancer that they have it. Of the remaining 990,000 women whose lumps were benign, we will incorrectly tell 49,500 women that they have cancer. Therefore, of the women we identify as having cancer, about 83 percent will have been incorrectly diagnosed.
Imagine you or a loved one received a positive test result. Which summary would you find more relevant? By ignoring the prior probability of the hypothesis, significance testing does the equivalent of diagnosing a medical condition based only on how often a patient would test positive if the condition were absent, or of reaching a legal verdict based only on how unlikely the facts of the case would be if the suspect were innocent. In short, significance testing would have told our hypothetical patient that she probably has cancer and would have wrongfully convicted Sally Clark.
Significance testing has been criticized along these lines for about as long as it’s been around. William Rozeboom, a professor of psychology at St. Olaf College, wrote in 1960 that the true logic of scientific inference was “inverse probability,” a.k.a. Bayes’ theorem. In 1966, David Bakan of the University of Chicago Department of Psychology referred to the logical fallacy of significance testing as something “everybody knows” but nobody would admit out loud, as in the story of the Emperor’s New Clothes. In 1994, the statistician Jacob Cohen wrote a scathing critique called “The Earth Is Round (p 1 called it the “permanent illusion.”
In the case of Sally Clark, the prosecution’s theory was she had murdered her children, itself an extremely rare event.
Thanks mostly to Fisher’s influence, these arguments have historically failed to win many converts to Bayesianism. But practical experience may now be starting to do what theory could not.
Suppose the women who received positive test results and a presumptive diagnosis of cancer in our example were tested again by having biopsies. We would see the majority of the initial results fail to repeat, a “crisis of replication” in cancer diagnoses. That’s exactly what’s happening in science today.
A follow-up study to Norenzayan’s finding, with the same procedure and almost ten times as many participants, found no significant difference in God-belief between the two groups. In fact, the mean God-belief score in “The Thinker” group was slightly higher (62.78) than in the control group (58.82). But because the original study followed all the usual rules of research, the journal was justified in accepting the paper, which means the rules are wrong.
High-profile replication failures like Norenzayan’s have led some scientists to call potentially all previous research into question. Large-scale projects have begun attempting to replicate the established results of various disciplines, and what they’ve found hasn’t been pretty. It started in psychology. A collaborative project involving hundreds of researchers through the Center for Open Science found only 35 of 97 psychology studies (that is, 36 percent) successfully replicated. All had used significance testing.
Just a few of the other casualties of replication include:
- The study in 1988 by Strack, Martin, and Stepper on the “facial feedback hypothesis:” when people are forced to smile, say by holding a pen between their teeth, it raises their feeling of happiness.
- The 1996 result of Bargh, Chen, and Burrows in “social priming,” claiming, for example, when people are exposed to words related to aging, they adopt stereotypically elderly behavior.
- Harvard Business School professor Amy Cuddy’s 2010 study of “power posing:” the idea that adopting a powerful posture for a couple of minutes can change your life for the better by affecting your hormone levels and risk tolerances.
But the crisis won’t stop there. Similar projects have shown the same problem in fields from economics to neuroscience to cancer biology. An analysis of preclinical cancer studies found that only 11 percent of results replicated; of 21 experiments in social science published in the journals Science and Nature, only 13 (62 percent) survived replication; in economics, a study of 18 frequently cited results found 11 (61 percent) that replicated; and an estimate for preclinical pharmacology trials is that only 50 percent of the positive results are reproducible, a situation that, given the immense size of the pharma industry, has been estimated to cost labs something like $28 billion per year in the U.S. alone.
We Bayesians have seen this coming for years. In 2005, John Ioannidis, now a professor at Stanford Medical School and the Department of Statistics, wrote an article titled “Why most published research findings are false.“2 He showed in a straightforward Bayesian argument that if a theory, such as an association between a gene and a disease, had a low prior probability, then even after passing a test for statistical significance it could still have a low probability of being true. He argued that this would be the norm in medicine, where a researcher can sift through many possible associations to find one that meets the threshold of significance merely by chance. Fourteen years later, we’re seeing the same phenomenon in virtually all areas of science.
Now, a consensus is finally beginning to emerge: Something is wrong with science that’s causing established results to fail. One proposed and long overdue remedy has been an overhaul of the use of statistics. In 2015, the journal Basic and Applied Social Psychology took the drastic measure of banning the use of significance testing in all its submissions, and this March, an editorial in Nature co-signed by more than 800 authors argued for abolishing the use of statistical significance altogether. Similar proposals have been tried in the past, but every time the resistance has been beaten back and significance testing has remained the standard. Maybe this time the fear of having a career’s worth of results exposed as irreproducible will provide scientists with the extra motivation they need.
Imagine you or a loved one received a positive test result. Which summary would you find more relevant?
The main reason scientists have historically been resistant to using Bayesian inference instead is that they are afraid of being accused of subjectivity. The prior probabilities required for Bayes’ rule feel like an unseemly breach of scientific ethics. Where do these priors come from? How can we allow personal judgment to pollute our scientific inferences, instead of letting the data speak for itself?
But consider the supposedly “objective” probabilities in the Clark case. Meadow came up with his figure of 1 in 73 million by applying some adjustments to the observed incidence rate of SIDS (about 1 in 1,300) to account for what was known about the Clark family: They were non-smokers with steady jobs and Sally was over the age of 26. How could he know he had adjusted for the all the right factors? Why not include the fact that she and her husband were both solicitors? The more specific information about the Clarks he included, the less available data he would have to go on, until his sample size was reduced to 1. He also assumed pairs of SIDS deaths in a family would be statistically independent, so their probabilities should get multiplied together, like the probability of a coin-flip coming up heads twice in a row. This assumption was roundly criticized at the time, because the independence would be negated by any environmental or hereditary factor the children shared. But given the paucity of data on such rare events, wouldn’t any correction for their dependency be somewhat subjective?
Drawing these lines, based on experience and expert judgment, is no less subjective than assigning a prior probability to a hypothesis such as Norenzayan’s based on what we know about the world. Furthermore, it may not matter too much exactly what prior probability we use. Whether we consider the chance to be 1 in 1 thousand, million, or billion, the Bayesian analysis would tell us Norenzayan’s results were not all that impressive, and we’d still be left extremely dubious. The point is that we have good reason to be skeptical, and we should follow the mantra of the mathematician (and Bayesian) Pierre-Simon Laplace, that extraordinary claims require extraordinary evidence. By ignoring the necessity of priors, significance testing opens the door to false positive results.
To a layperson, this debate about statistical methods may seem like an esoteric squabble, but the implications are much larger. We all have a stake in scientific truth. From small individual decisions about what foods to eat or what health risks to worry about, to public policies about education, healthcare, the environment, and more, we all pay a price when the body of scientific research is polluted by false positives. Eventually replication studies can sort the true science from the noise, but only at considerable cost. In the meantime we may be constantly upended by contradictory findings based only on statistical phantoms.
To address the crisis of replication, we must change the way we quantify and manage uncertainty in science. In its long history, probability has been misused to support bad reasoning in a wide variety of settings, from sports to medicine, economics, and the law. Most of these mistakes have, eventually, been corrected. Sally Clark was acquitted after spending three years in prison when it came to light that the pathologist who examined her second child had withheld key evidence from both the prosecution and the defense. But her appeal also exposed the flaws in Meadow’s statistical argument. Two other women, Angela Cannings and Donna Anthony, who had been convicted in similar cases based on Meadow’s testimony were released, and a third, Trupti Patel, on trial for the murder of her three infants, was acquitted. But the trauma of being wrongfully imprisoned for murdering her children continued to take its toll on Clark. A few years after being released she died of alcohol poisoning.
Medical students are now routinely taught the diagnostic importance of base incidence rates. Bayes’ theorem helps them properly contextualize test results and avoid unnecessarily alarming patients who test positive for something rare. To leave out that final ingredient, the Bayesian prior probability, would be to commit a fallacy of the same species as the one in the Sally Clark case.
The crisis of replication has exposed the fact, which has been the shameful secret of statistics for decades now, that the same fallacy is at the heart of modern scientific practice.
Aubrey Clayton is a mathematician living in Boston. He teaches logic and philosophy of probability at the Harvard Extension School.
References
1. Gigerenzer, Gerd. “The superego, the ego, and the id in statistical reasoning.” A handbook for data analysis in the behavioral sciences: Methodological issues (1993): 311-339.
2. Ioannidis, John PA. “Why most published research findings are false.” PLoS Medicine 2, no. 8 (2005): e124.