In Ithaca, New York, a virtual machine in a laboratory at the Cornell Lab of Ornithology sits in the night, humming. The machine’s name is Bubo, after the genus for horned owls. About every five minutes, Bubo grabs an image from Northeast weather radar stations, and feeds it through a pipeline of artificial-intelligence algorithms. What does this radar image show me? Bubo asks. Is it rain? Are these insects? Could it be pollen? Bubo doesn’t care about those things; all it wants to see are birds in flight. To find them, Bubo analyzes the velocity and direction of targets seen by the radar station. Bubo knows birds have a velocity different from wind and insects, and filters those out. Now Bubo sees only birds. But how dense are they? How fast are they going? How high in the sky are they flying? The machine makes these calculations and creates an image of countless birds in flight, traveling under cover of darkness.
“If we could see at night, we would see millions of birds flying overhead,” says Thomas Dietterich, a professor of computer science at Oregon State University, who works with the Cornell Lab of Ornithology. Black-chinned hummingbirds fly along the Mexican coast on their way to Alaska. Yellow-throated vireos soar over the Gulf Coast, headed to Ontario. Olive-and-yellow flycatchers sail across Central America, bound for the Northwest Territories. “It’s just so awe-inspiring that there’s this huge, secret thing happening that we’re unaware of.”
Scientists have long sought to penetrate the secrets of bird migrations. They have illuminated the remarkable means by which birds find their way across the globe. These include birds’ cognitive maps of terrain and continents, and mechanisms in their eyes that detect the earth’s magnetic poles and orient flight direction.
Andrew Farnsworth, a research associate at the Cornell Lab of Ornithology’s Information Science department, wants to understand bird migrations beyond biology. He’s after the big picture. “How does migration function within a larger ecosystem?” he asks. “What does it mean when ecosystems change?”
For the past four years, Farnsworth and his colleagues, a group of ecologists, statisticians, computer scientists, and a meteorologist, have been working on a project called BirdCast, leading to the machine learning of Bubo, to uncover the secrets of migration. Some of these secrets are contained within the estimated 100 million weather radar scans taken over the last 20 years and archived by the National Oceanic and Atmospheric Administration. Others are within tens of millions of bird sightings reported every year on eBird, an online platform where bird watchers around the world log species-specific observations. And still more are in the thousands of hours of nighttime birdcalls recorded by acoustic monitoring devices scattered around the country.
Ornithologists working for Britain’s war effort guessed the objects were birds but few people believed it—they didn’t think birds would fly at night.
With BirdCast, the Big Data revolution meets wildlife conservation. Imagine municipalities that turn lights off in advance of approaching birds so as not to disorient them, or areas where birds stop to rest and feed during the day that are protected from pesticides and wind turbines. “Most traditional conservation is setting aside particular areas; it’s static,” says Farnsworth. “This is becoming the age of more dynamic conservation. How can we change our behavior before something happens?” This predictive ability could help conservationists mitigate threats to birds from sprawl, development, and environmental changes wrought by climate change. But first, says Dietterich, “we need to have much better models of where birds are going and what paths they are following.”
Before radar, few people knew much about nocturnal migrations. In the 1930s, militaries on both sides of the Atlantic raced to develop technology that would give them advance warning of enemy aircraft. Weather radars send out bursts of radio waves, called pulses, that bounce off objects in the atmosphere. The radar calculates the shape and distance of an object based on the speed and power of the returning echo. Radar turned out to be capable of detecting Heinkels and Messerschmitts and weather fronts. But other objects moving in the atmosphere eluded categorization. The British military’s radar operators called the mysterious objects angels and the Germans called them Scheinziele, “spurious echoes.” Whatever they were, they had been creating havoc for everyone, sending men to battle stations and chasing after ghost planes. Ornithologists working for Britain’s war effort guessed the objects were birds but few people believed it—they didn’t think birds would fly at night.
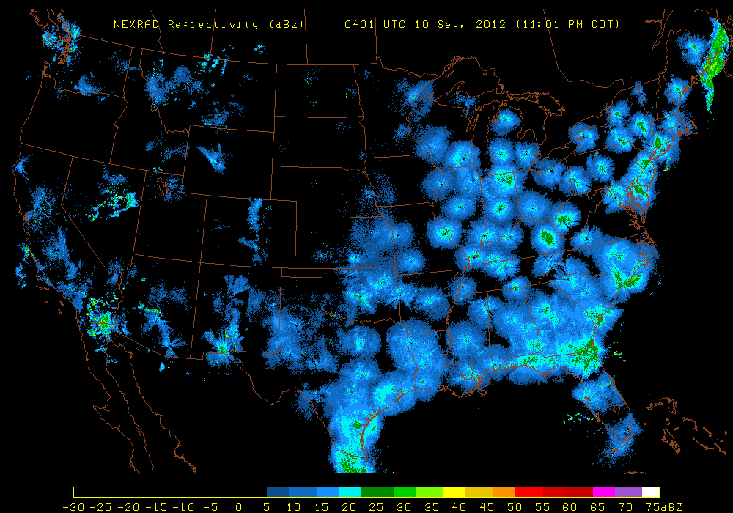
In the years following World War II, one of the pioneers of the field called radar ornithology was a kid in New Orleans. Sidney Gauthreaux’s hometown was in the pathway of one of the busiest migration corridors in North America. The most direct route for species returning from winter grounds in the Caribbean, South America, and Central America is over the Gulf of Mexico, which they cross in a single trip—400 to 600 miles—to make landfall. As a child, Gauthreaux lay awake at night listening to the flight calls in the dark outside his bedroom. “My career has been devoted to understanding what’s happening in the atmosphere at night because we can’t see it,” Gauthreaux says today.
When Gauthreaux was in high school in the 1950s, the first modern weather radar system was installed along the Gulf, part of a national network of 50 stations called WSR-57. If the stations were sensitive enough to detect raindrops, Gauthreaux thought, shouldn’t they pick up moisture on the bodies of the birds he heard in the night? He got his hands on radar images and could see clouds of snowy masses that he knew had to be birds. The discovery fueled Gauthreaux’s passion for bird migration, and in the late 1970s he built the first mobile radar lab for bird studies.
In 1990, Gauthreaux founded the Radar Ornithology Laboratory at Clemson University, around the same period the national weather system was upgraded. The weather system consisted of 159 stations that emitted microwave energy to capture the density of targets, and used Doppler to record radial velocity (the speed at which a target is approaching or departing from the radar beam) and direction. Radar data from the network, called WSR-88D, allowed ornithologists to not only estimate how many birds were in flight, but at what speed and direction they were heading.
The problem of predicting migration is it involves potentially billions of birds making individual decisions about whether to fly that night and where to go.
In 1999, one of Gauthreaux’s graduate students was Farnsworth, who focused his master’s thesis on the correlation between nocturnal flight calls and the density of birds recorded by radar. The work required a formidable amount of time and effort. Farnsworth had to listen to hours of recorded flight calls, and manually categorize each radar image after distinguishing between weather, insects, bats, and birds—an expert skill that required a lot of practice. For his thesis, Farnsworth analyzed changes in hour-to-hour bird density and bird vocalizations from 556 hours over 58 nights. It took him eight months.
In 2000, with funding from the Environmental Protection Agency, the precursor to BirdCast was born in a collaboration that included the Radar Ornithology Lab at Clemson, Cornell’s Lab of Ornithology, and the National Audubon Society. The goal was to forecast bird migrations for the mid-Atlantic corridor, based on radar scans and weather forecasts, as well as on sightings amassed by citizen scientists. The project, which demanded incredible amounts of human and financial resources, ended after two years. “The connectivity of the world was not at a place where this idea was practical,” Farnsworth says. “It was pre-Big Data, the whole idea of citizen science had not exploded.”
By 2011, the context had changed. If the original BirdCast couldn’t grow because of human constraints, the scientists decided to take humans out of the loop. The challenge was whether an artificial intelligence model could acquire the expertise required to analyze radar images, not only by discriminating between weather, insects, and birds, but inferring the velocities and direction of migrating birds at different elevations. If it could, petabytes of historical data would become available for study, as well as the possibility of tracking migrating birds quickly and efficiently in near real-time at both regional and national scales. With funding from the National Science Foundation, the nascent BirdCast team, joined by Daniel Sheldon, an assistant professor in computer science at the University of Massachusetts, Amherst, got down to work and Bubo was hatched.
Now, every night, Bubo downloads data from 17 radar stations as well as weather data that will help it distinguish birds from wind and precipitation. Bubo’s method is derived from one used by meteorologists to profile wind direction and speed through radar, but it has a novel feature to deal with the problem of “aliasing.” Aliasing occurs because WSR-88D’s radars can’t calculate radial velocities above a certain amount, and these errors can distort the scans, showing objects above a certain speed as moving outbound from the radar station rather than inbound and vice versa. Aliasing isn’t new but Sheldon and his BirdCast colleagues came up with a new approach by developing a probabilistic model that accounts for aliasing in the radial velocity values, and writing an inference algorithm to reconstruct a complete velocity field. In 2013, the innovations earned the team a best paper award from the Association for the Advancement of Artificial Intelligence. Bubo’s machine learning pipeline can analyze a radar image in 17 seconds, and a night’s worth of images in less than an hour.
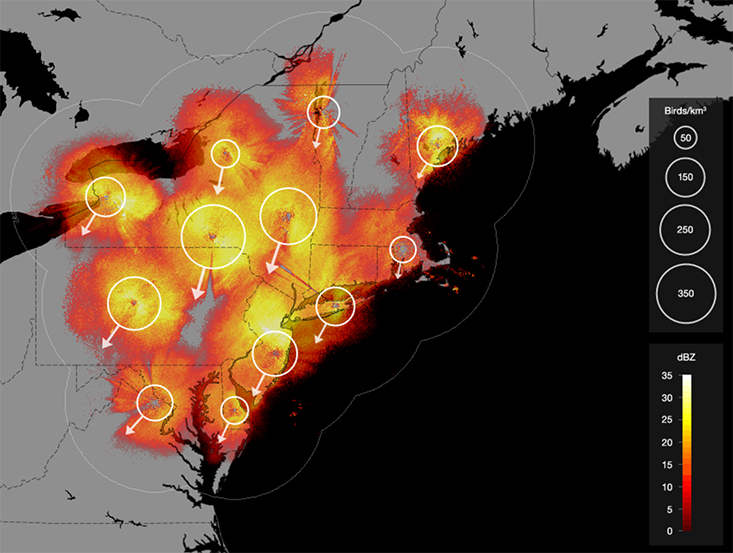
“We know birds fly south in the fall and north in the spring,” says Jeffrey Buler, an assistant professor of wildlife ecology at the University of Delaware, who uses radar data to study bird migration, though is not a member of BirdCast. “Now we have a way to directly observe these things and ask nuanced questions. What’s also nice about the work they’re doing is they are starting to tap into the potential of the radar archive.”
Bubo has been in operation since May and won’t generate data for an entire migration period until this fall. But the BirdCast team has begun to analyze historical data, including nearly 40,000 radar scans from the northeastern United States from two fall migrations in 2010 and 2011. The study shows that birds migrating inter-continentally over the Atlantic leave earlier and use a different route than intra-continental migrants coming later. Findings like these could help biologists understand how climate change is affecting migrant species, something Gauthreaux has been researching at Clemson University. His preliminary findings have led him to believe that whereas shorter-distance migrants are responding to seasonality changes in recent decades, long-distance species aren’t changing their timetables. “The consequences are that these species may be out of sync with the production of food and breeding grounds,” Gauthreaux says. “Initially that could result in fairly dramatic population decline. And for species that aren’t very healthy that could mean extinction: The population could get so low that they couldn’t adapt and the species would blink out.”
As Bubo collects data, BirdCast is preparing to expand two other artificial intelligence experiments. The first uses machine learning algorithms to identify nocturnal flight calls of migrating birds that are recorded by acoustic devices. So far, there are 10 devices in New York state, each equipped with a detector that screens for flight calls. The calls are uploaded to a central server, where they are processed by an algorithm that can identify six different bird species with 95 percent accuracy. “It’s one of the first times we’ve been able to train machines to automatically detect and identify a bird by the sound it makes,” says Steve Kelling, director of information science at the Cornell Lab of Ornithology.
Second, BirdCast will begin testing a statistical model to use eBird data to predict bird migrations one to two days before they happen. Since it launched in 2002, eBird has become an impressive source of citizen-generated data. Amateur bird watchers, many using the eBird app, report millions of sightings each month. In February of 2015, during a four-day global bird count, over 140,000 people from 100 countries submitted sightings to eBird. All that data, though, presents a challenge. The problem of predicting migration, Dietterich explains, is migration involves potentially billions of birds making individual decisions about whether to fly that night and where to go. If a computer had to consider all of these variables, it would be computationally intractable. Sheldon’s breakthrough was a “Collective Graphical Model.” Instead of including a variable for every member of the bird population, the Collective Graphical Model avoids reasoning about individuals and focuses on groups, using an algorithm to infer how many birds migrate from one spot to another. “It sounds obvious but the breakthrough was to realize that the probability model can lift from individual to aggregate,” Sheldon says.
For BirdCast, the grail is creating a model that integrates data from all three streams—radar, acoustic, and eBird. The model could test hypotheses about the forces shaping migration, revealing relationships between migration and the atmosphere that currently elude our perception. These are insights, Farnsworth says, that can’t come soon enough. “There are some fundamental natural history questions to which we don’t know answers,” he says. “What does it mean when things start to change? Patterns in the jet stream? Polar vortex? Changes in the atmosphere over broad scales of space? These are the sorts of things that we’re right on the tip of understanding.”
M.R. O’Connor is a journalist based in Brooklyn whose first book Resurrection Science: Conservation, De-Extinction and the Precarious Future of Wild Things will be published in September.
Photocollage compiled from images from Richard Bartz, Paul Souders, and Michael J. Bennett.