Presbyterian reverend Thomas Bayes had no reason to suspect he’d make any lasting contribution to humankind. Born in England at the beginning of the 18th century, Bayes was a quiet and questioning man. He published only two works in his lifetime. In 1731, he wrote a defense of God’s—and the British monarchy’s—“divine benevolence,” and in 1736, an anonymous defense of the logic of Isaac Newton’s calculus. Yet an argument he wrote before his death in 1761 would shape the course of history. It would help Alan Turing decode the German Enigma cipher, the United States Navy locate Soviet subs, and statisticians determine the authorship of the Federalist Papers. Today it has helped unlock the secrets of the brain.
It all began in 1748, when the philosopher David Hume published An Enquiry Concerning Human Understanding, calling into question, among other things, the existence of miracles. According to Hume, the probability of people inaccurately claiming that they’d seen Jesus’ resurrection far outweighed the probability that the event had occurred in the first place. This did not sit well with the reverend.
Inspired to prove Hume wrong, Bayes tried to quantify the probability of an event. He came up with a simple fictional scenario to start: Consider a ball thrown onto a flat table behind your back. You can make a guess as to where it landed, but there’s no way to know for certain how accurate you were, at least not without looking. Then, he says, have a colleague throw another ball onto the table and tell you whether it landed to the right or left of the first ball. If it landed to the right, for example, the first ball is more likely to be on the left side of the table (such an assumption leaves more space to the ball’s right for the second ball to land). With each new ball your colleague throws, you can update your guess to better model the location of the original ball. In a similar fashion, Bayes thought, the various testimonials to Christ’s resurrection suggested the event couldn’t be discounted the way Hume asserted.
In 1767, Richard Price, Bayes’ friend, published “On the Importance of Christianity, its Evidences, and the Objections which have been made to it,” which used Bayes’ ideas to mount a challenge to Hume’s argument. “The basic probabilistic point” of Price’s article, says statistician and historian Stephen Stigler, “was that Hume underestimated the impact of there being a number of independent witnesses to a miracle, and that Bayes’ results showed how the multiplication of even fallible evidence could overwhelm the great improbability of an event and establish it as fact.”
The statistics that grew out of Bayes and Price’s work became powerful enough to account for wide ranges of uncertainties. In medicine, Bayes’ theorem helps measure the relationship between diseases and possible causes. In battle, it narrows the field to locate an enemy’s position. In information theory, it can be applied to decrypt messages. And in the brain, it helps make sense of sensory input processes.
The application of Bayes’ theorem to the brain began in the late 19th century. German physicist Hermann von Helmholtz used Bayes’ ideas to introduce the idea of converting sensory data like, say, spatial awareness, into information through a process he called “unconscious inference.” As Bayesian statistics gained popularity, the idea that unconscious mental calculations are probabilistic in nature didn’t seem so farfetched. According to the “Bayesian brain hypothesis,” the brain constantly uses Bayesian inference to “fill in” missing sensory information, just as each successive ball thrown on the table in Bayes’ thought experiment “fills in” information about the location of the first ball. The “Bayesian brain” has an internal model of the world—expectations (or hypotheses) about how objects should look, feel, sound, behave, and interact—that takes in sensory input and constructs, to a certain approximation, what’s actually happening around us.
Take vision. Light bounces off objects surrounding us and hits the surface of the retina, and somehow the brain must generate a three-dimensional image from that two-dimensional data. Many three-dimensional scenes could be generated from it, so how does the brain decide which to show you? Perhaps it’s using a Bayesian model to do so. But for the brain to have evolved to make such near-perfect statistical computations seems unlikely. Our computers can’t handle performing the enormous number of probabilistic calculations we seem to be making every moment. Maybe the brain can’t either. According to one theory, called sampling, the brain may be approximating Bayesian inference: Rather than simultaneously representing all hypotheses that explain a given sensory input, it takes into account only a few hypotheses sampled randomly (the number of times each hypothesis is sampled is based on its prior likelihood of occurrence).
That might explain why we experience visual illusions the way we do: The brain makes a “best guess” according to the rules of Bayesian inference—one that ends up being incorrect because the visual system fills in missing details by sampling from an internal model that doesn’t apply. Two squares on a chess board appear to be different shades of gray, for example, or a circle seems concave at first but becomes convex when flipped 180 degrees, because the brain makes a wrong initial assumption about something as simple as lighting.
It also helps explain why people—and their recollections, impressions, decisions—are more heavily influenced by early information they receive, says Adam Sanborn, a behavioral scientist at the University of Warwick. People implicitly prefer to buy goods from the first salesperson they meet. Gamblers may be more likely to continue to play at the slot machine if they experience early winnings that day. And first impressions are often difficult to shake, even if they’re dead wrong. “With the first information you get,” Sanborn says, “you’ll think of hypotheses that are consistent with that information.”
This variability takes place all the way down on the neuronal level. “The idea is that the activity of a neuron represents the value of a random variable you’re trying to infer,” says Máté Lengyel, a computational neuroscientist at the University of Cambridge. In other words, the variability of a neuron’s activity describes an event’s probability. Consider a simplified example, a neuron that represents “tiger,” he says. The neuron will fluctuate between two activity levels: sometimes high, signaling the tiger is there, and sometimes low, meaning there’s no tiger. The fraction of the time the neuron spends in the high activity state approximates the probability of the tiger’s presence. “Essentially, in this case we can say the neuron’s activity is sampling from the distribution that needs to be represented,” Lengyel says. “It turns out that if you pursue this idea in a more realistic, less simplistic way, it captures a lot of the things we know about neurons and the variability in their responses.”
In some sense, says Thomas Hills, one of Sanborn’s colleagues, the way we sample from mental representations is similar to how we forage for physical objects in space. If you have most often found milk in the back of a supermarket, that’s the first place you’ll look when buying milk at a new store. It’s no different when it comes down to searching through the internal representations housed in the mind. “You can think of memories as some kind of record of the rational frequency of events in the world,” Hills says. “They’re encoded in your mental representation in proportion to the extent you’ve experienced them in the past. So if I ask you about your relationship with your mom, you may go: I have this memory of a good interaction, this memory of a good interaction, this memory of a bad interaction. But on average the memories that come to mind are good, so you’d answer ‘good.’” The brain is a kind of search engine that samples from memories to build what Hills calls our “belief structures”—about our ties with our parents, about how we define “dog” or “friend” or “love,” about everything.
And if this search process goes wrong—if the brain retrieves information from samples that aren’t representative of a person’s experience, if there’s mismatch between expectation and sensory input—it can lead to depression, obsessive-compulsive disorder, PTSD, or a number of other illnesses.
This is not to say that the Bayesian brain hypothesis doesn’t have critics. “I think the Bayesian framework as a sort of mathematical language is a really powerful and useful way of expressing psychological theories,” says Matt Jones, a cognitive neuroscientist at the University of Colorado Boulder. “But it’s important to think about what parts of the theory are really doing the explanation.” He thinks Bayesian brain proponents give too much credit to the statistical-analysis part of the theory. “That by itself doesn’t explain a lot of behavior,” he says. “It’s only meaningful when you couple it with what turns out to be rich assumptions about the nature of knowledge representation—how we organize concepts, search through memory, and use knowledge to do reasoning and problem solving.”
In other words, the claims we make about psychological processing—what traditional cognitive science is all about—inform how Bayesian statistics is applied to the brain’s functions. The model expresses those theories in the language of mathematics, but old-fashioned psychology is doing the actual explaining. It may end up being the case that other, non-Bayesian models—or different Bayesian models—are a better fit for the assortment of mental processes that underlie our sensory perceptions and higher cognition.
While Sanborn might disagree with Jones’ opinions on the Bayesian brain hypothesis, he also believes the next step is to narrow down the models that work. “You can say that sampling itself is a useful way to conceive of what the brain is trying to do,” he says, “but there are a lot of samplers out there.” Whether those samplers are Bayesian remains to be seen. What is apparent is that Bayes’ defense of Christianity in the 18th century has helped scientists make great strides through the 21st century.
Jordana Cepelewicz is an editorial fellow at Nautilus.
Watch: The neuroscientist Christof Koch explains how a computer or the Internet could be conscious.
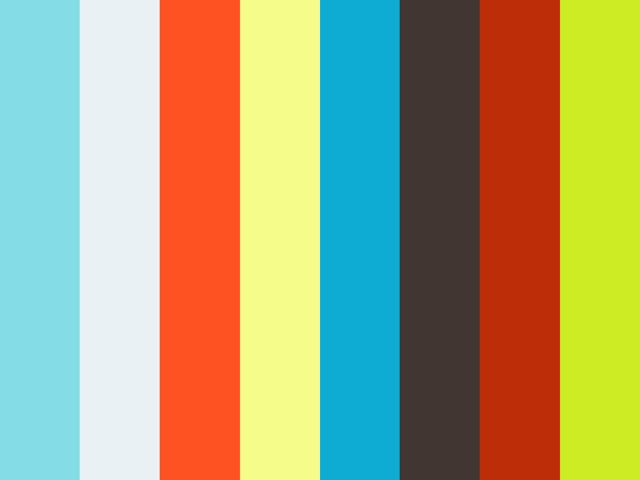