The brain is often called a black box but any neuroscientist who has looked inside knows that’s a sobering understatement. Technological advances are making our neural circuitries increasingly accessible, allowing us to closely watch any number of neurons in action. And yet the mystery of the brain only deepens. What’s the meaning embedded in the collective chorus of spiking neurons? How does their activity turn light and soundwaves into our subjective experience of vision and hearing? What computations do neurons perform and what are the broad governing principles they follow? The brain is not a black box—it’s an alien world, where the language and local laws have yet to be cracked, and intuitions go to die.
Could artificial intelligence figure it out for us? Perhaps. But a recent recognition is that even our newest, most powerful tools that have achieved great success in AI technology are stumbling at decoding the brain. Machine learning algorithms, such as artificial neural networks, have solved many complex tasks. They can predict the weather and the stock market or recognize objects and faces, and crucially, they do so without us telling them the rules. They should, at least in theory, be able to learn the hidden patterns in brain activity data by themselves and tell us a story of how the brain operates. And they do tell a story. It’s just that, as some scientists are finding, that story is not necessarily of our brain.
Computational attempts at describing the behavior of neurons have always led to humble lessons.
That’s what Tatiana Engel, assistant professor at Cold Spring Harbor Laboratory, discovered recently when investigating decision-making in the brain. A physicist turned computational neuroscientist, Engel works on developing mathematical models that could help explain what neurons do when we make decisions. While neuroscientists have some theories, they have yet to arrive at an all agreed-upon account of how decisions, even the simplest ones, are implemented in the brain. Hoping to explore a wider range of possibilities, Engel turned to machine learning: Instead of working up from specific hypotheses to model the neural activity, she started with flexible models that can mold themselves to the data and figure out the parameters of their equations on their own.
In this method, the resulting models are then judged by how well they can predict a new set of brain measurements they have not seen before. But along the way, Engel wondered, just how sure are we that the best-scoring model reflects the underlying logic of the brain?
“It’s more and more common now in neuroscience, as well as in other fields, to use this type of flexible models as a tool to understand the real physical, biological systems,” says Engel. “So we build a model, and it can predict the data from a system very well. Then there is this kind of assumption that such a model should function in a way similar to the real system and therefore, by studying how the model works, we will understand how the system works.”
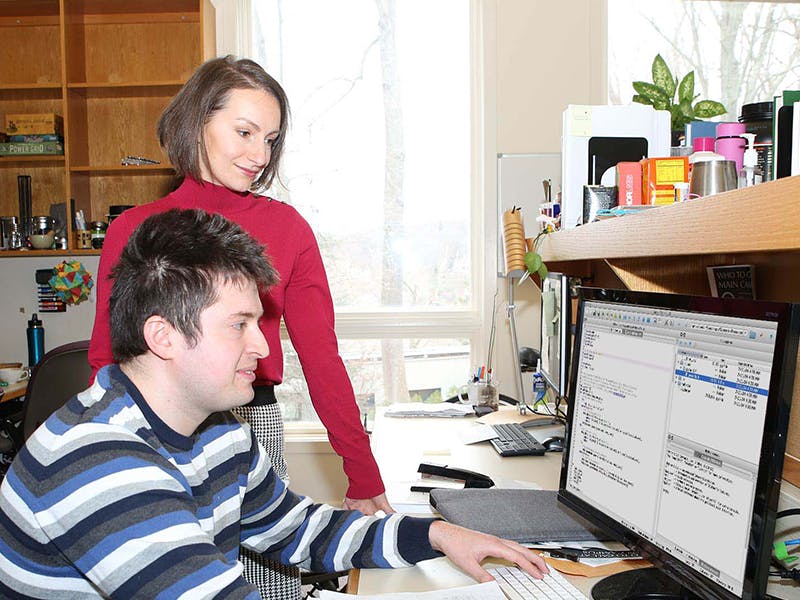
More often than not, that assumption may be unwarranted. In a 2020 study, Engel and her colleague Mikhail Genkin, a postdoc at CSHL, examined how well flexible models would work on synthetic data, whose internal dynamics was known to the researchers.1 They found that counterintuitively, sometimes models that ranked as the strongest predictors turned out to be the farthest from mirroring the core features of the original system that generated the data. “They can have features or attributes that are not present in the system at all,” Engel says. “A model can make good predictions about data and still be wrong.” In other words, prediction power, the gold standard for machine learning algorithms, can be a misleading metric when it comes to neuroscience applications.
Without working computational models, scientists may have very little chance at making sense of the deluge of brain data and explaining how neural activity gives rise to brain functions. Engel’s findings and those of other researchers may feel like a blow to the highly touted promises of AI’s ability to aid with modeling the brain. These problems, however, are not unsurmountable, Engel says. She and others are already coming up with ideas to avoid these traps. They are developing methods that would allow them to continue using AI’s powerful tools without falling for misleading stories.
Computational attempts at describing the behavior of neurons have always led to humble lessons, even when those attempts were successful. In 1952, Alan Hodgkin and Andrew Huxley imagined a neuron as an electrical circuit whose carefully arranged resistors and capacitors could generate a current similar to the neuron’s characteristic spike, the building block of communications in the brain. The model proved to be a pivotal achievement, but it was far from straight-forward to know that by just looking at the equations. As Huxley spent days painstakingly entering voltage numbers into a mechanical calculator to see whether the circuit’s outcomes matched those of a real neuron, he marveled at the sophisticated behavior of this relatively simple model. “Very often my expectations turned out to be wrong,” he recounted a decade later in his Nobel prize lecture. “An important lesson I learnt from these manual computations was the complete inadequacy of one’s intuition in trying to deal with a system of this degree of complexity.”
Neuroscientists now face orders of magnitude higher complexity as they have moved on to studying populations of neurons in living animals and people. The data, even from just 100 neurons, is dizzyingly large. It varies dynamically with no obvious rhyme and reason. And it’s rarely clear which parts of it are truly relevant to the brain function being studied. These factors have made it much harder to come up with models, conceptual or mathematical, to describe the neural activity.
The data, even from just 100 neurons, is dizzyingly large
Even harder is figuring out which proposed model explains something real about the neurons, and which one is a lucky mathematical match for data. Without knowing the ground rules in the brain, the best scientists can do is to see how the models stack up against each other.
“It’s like all we see is a moving car, and we have to find out how [it moves] by making assumptions about what’s happening under the hood,” says Chandramouli Chandrasekaran, a neuroscientist at Boston University who collaborates with Engel on decision-making research. “We then try to figure out which of the proposed ideas, say model A and model B, does better at matching our measurements of the car’s movements.”
Although an increasingly popular method, this approach can still fail in important ways, Chandrasekaran says. As a hybrid computational and experimental researcher working directly with brain measurements, Chandrasekaran knows firsthand that neural activity is nothing like a smooth riding car—it’s naturally too complex to ever fit squarely inside the lines of our roughly sketched models. “Experimental data is often much more complex and heterogeneous. It’s what it is. It’s not as simple and beautifully boxed in as what you think it is,” he says. What this means in practice, Chandrasekaran has shown, is that tangential variations in neural activity can sometimes cause it to be classified as model A, when in reality it abides by model B, or vice versa.2 That’s one reason why comparing two models head-to-head is not guaranteed to identify the correct one.
A raging debate that erupted recently in the field of decision-making highlights these difficulties. It started with controversial findings of a 2015 paper in Science that compared two models for how the brain makes decisions, specifically perceptual ones.3 Perceptual decisions involve the brain making judgments about what sensory information it receives: Is it red or green? Is it moving to the right or to the left? Simple decisions, but with big consequences if you are at a traffic stop. To study how the brain makes them, researchers have been recording the activity of groups of neurons in animals for decades. When the firing rate of neurons is plotted and averaged over trials, it gives the appearance of a gradually rising signal, “ramping up” to a decision.
Neuroscientists don’t want to just fit a model to the data, but to discover hypotheses from the data.
In the standard narrative based on an influential model that has been around since the 1990s, the ramp reflects the gradual accumulation of evidence by neurons. In other words, that is how neurons signal a decision: by increasing their firing rate as they collect evidence in favor of one choice or the other until they are satisfied. The 2015 study, however, asked whether the ramping is an artifact of averaging over trials. It’s much harder to analyze the messy limited data of a single trial, but what happens in it? Does a neuron’s firing rate really ramp up or does it make discrete jumps? The distinction could point to different strategies underlying decision-making. Their analysis suggested that responses of neurons match a jumping model better than a ramping model. Several years and many studies later, scientists still don’t have a firm conclusion on which model is correct.
And the situation could be even worse: Neither model may be correct. “What if there’s a model C? Or D?” Engel says. What if instead of two models, she could test a continuum of models? That’s where flexible modeling would be most useful, as it wouldn’t constrain her to just a handful of scenarios. But Engel had found that this approach could also select scenarios that didn’t have much in common with the physical reality under the hood. First, she had to find a way around that problem.
Flexible models are developed with the goals of machine learning in mind: optimizing for prediction ability. In this way, they can take what they learn from a set of data and apply it to new data they have not seen before. For example, when building a classifier to tell cats apart from dogs, the goal is that out in the real world it can still tell cats from dogs. It doesn’t matter if the algorithm achieves this using the same strategy as our brains or not. In fact, in this case, it definitely does not.
Neuroscientists on the other hand have a fundamentally different goal: They don’t want to just fit a model to the data, but to discover hypotheses from the data. They want to have a model that can learn from neural activity how to be like neurons. “We had to abandon this idea of optimizing models for predictions, and come up with a new approach which puts a different objective forward,” Engel says. Together with Genkin she focused on the fact that across different data samples, true features are the same but noise is different. So they developed a new procedure that allows discovering models on different data samples, and extracting the features they have in common. This new approach identified the correct model of the synthetic data. And when applied to real brain data, it arrived at similar models for each sample, suggesting that unlike the wild guesses of the conventional method, these models had captured some of the true features of the system.
This solution, published in Nature Machine Intelligence, would make flexible models more extendable beyond their original purposes and more useful to biological sciences.1 It may not be a solution to every case of AI tools used in neuroscience, Engel says, but it can improve the application of flexible models, which is used widely by neuroscientists.
For Engel herself it has already started to yield new insights into decision-making. Collaborating with Chandrasekaran, the team is exploring their original question: What kind of model best describes neural activity during decision-making? So far what they see is neither ramping or jumping. Would their findings settle the debate? Or kick it further into another round? Hopefully, we’ll know sometime soon.
Bahar Gholipour is a science writer and editor covering biomedical sciences, genetics, neuroscience, and AI. Her work has appeared in The Atlantic, Scientific American, Wired and many other publications. She has also written and produced for the PBS YouTube channels Brain Craft and Space Time. She has a bachelor’s in computer engineering from Sharif University in Tehran, received a master’s in neuroscience from École Normale Supérieure in Paris, and has published academic papers in brain imaging. Her writing has been featured in The Language of Composition and in The Best American Science and Nature Writing. She lives in Woodstock and New York City.
Lead image: Golden Sikorka / Shutterstock
References
1. Genkin, M. & Engel, T.A. Moving beyond generalization to accurate interpretation of flexible models. Nature Machine Intelligence 2, 674-683 (2020).
2. Chandrasekaran, C., et al. Brittleness in model selection analysis of single neuron firing rates. BioRxiv (2018). Retrieved from DOI:10.1101/430710
3. Latimer, K.W., Yates, J.L., Meister, M.L.R., Huk, A.C., & Pillow, J.W. Single-trial spike trains in parietal cortex reveal discrete steps during decision-making. Science 349, 184-187 (2015).